Definition Z Test
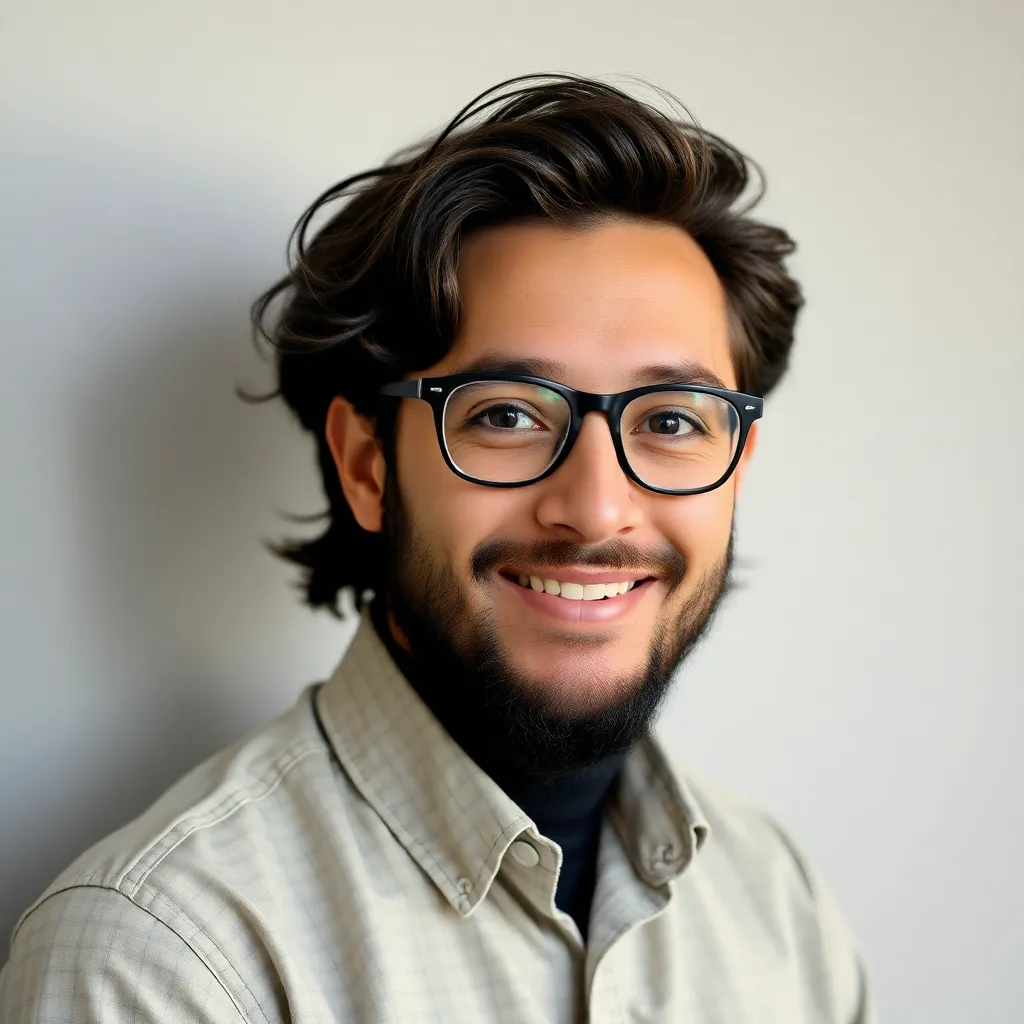
adminse
Apr 02, 2025 · 10 min read

Table of Contents
Decoding the Z-Test: A Comprehensive Guide
What if understanding the nuances of a z-test unlocks the power of statistical inference for your data analysis? This powerful statistical tool is fundamental to drawing meaningful conclusions from your data.
Editor’s Note: This article on z-tests provides a detailed explanation, covering its definition, applications, and interpretations. It aims to equip readers with the knowledge to confidently utilize this crucial statistical method. Updated October 26, 2023.
Why Z-Tests Matter: Relevance, Practical Applications, and Industry Significance
Z-tests are a cornerstone of inferential statistics, allowing researchers and analysts to draw conclusions about a population based on a sample of data. Their application spans diverse fields, from healthcare and finance to marketing and social sciences. In healthcare, z-tests might be used to compare the effectiveness of a new drug against a placebo. Financial analysts might employ them to determine if the average return of a specific investment strategy significantly differs from the market average. Marketers leverage z-tests to assess the impact of advertising campaigns on sales figures. The widespread applicability of z-tests highlights their importance in making data-driven decisions. Understanding z-tests facilitates informed choices based on statistically sound evidence rather than mere speculation. The ability to identify statistically significant differences or relationships empowers individuals to develop more effective strategies and make better predictions.
Overview: What This Article Covers
This article offers a comprehensive exploration of z-tests. We'll cover the underlying principles, different types of z-tests, the assumptions required for valid results, step-by-step procedures for conducting a z-test, and finally, how to interpret the results. Readers will gain a solid understanding of this powerful statistical tool and its applications.
The Research and Effort Behind the Insights
This article synthesizes information from numerous statistical textbooks, research papers, and online resources. Emphasis is placed on clarity and accuracy, ensuring the information presented is both comprehensive and easily understandable. Each step in the z-test procedure is explained, and examples are provided to illustrate the application in real-world scenarios.
Key Takeaways:
- Definition and Core Concepts: A clear definition of the z-test, including its purpose and underlying assumptions.
- Types of Z-Tests: An exploration of different z-tests, including one-sample, two-sample, and z-tests for proportions.
- Step-by-Step Procedure: A detailed guide on how to perform a z-test, including calculations and interpretation.
- Interpreting Results: Understanding p-values, critical values, and the significance of the results.
- Common Errors: Identifying and avoiding pitfalls when conducting and interpreting z-tests.
Smooth Transition to the Core Discussion
Now that we've established the importance of z-tests, let's delve into the specifics, starting with a precise definition and exploring the various types and applications.
Exploring the Key Aspects of Z-Tests
1. Definition and Core Concepts:
A z-test is a statistical test used to determine whether two population means are different when the population variances are known and the sample size is large (generally considered n ≥ 30). It's based on the z-statistic, a measure of how many standard deviations a data point is from the population mean. The test assumes that the data follows a normal distribution. The z-test helps determine if observed differences between sample means are likely due to random chance or represent a real difference in the population means. Central to the z-test is the concept of the standard error, which measures the variability of the sample mean. A smaller standard error indicates a more precise estimate of the population mean.
2. Types of Z-Tests:
-
One-Sample Z-Test: This test compares the mean of a single sample to a known or hypothesized population mean. For example, a researcher might use a one-sample z-test to determine if the average height of students in a specific school is significantly different from the national average height.
-
Two-Sample Z-Test: This test compares the means of two independent samples. For instance, a company might use a two-sample z-test to compare the average sales performance of two different sales teams.
-
Z-Test for Proportions: This test compares two population proportions. It's useful when dealing with categorical data, such as determining if the proportion of men who prefer a certain brand is significantly different from the proportion of women who prefer the same brand.
3. Assumptions of Z-Tests:
Several assumptions must be met for a z-test to yield valid results:
- Data Normality: The data should be approximately normally distributed, or the sample size should be large enough for the Central Limit Theorem to apply.
- Independence: Observations within each sample must be independent of each other.
- Known Population Variance: The population variance (or standard deviation) must be known for the test to be accurate. If the population variance is unknown, a t-test should be used instead.
4. Step-by-Step Procedure for a One-Sample Z-Test:
-
State the Hypotheses: Define the null hypothesis (H₀) and the alternative hypothesis (H₁). For example:
- H₀: μ = μ₀ (The population mean is equal to the hypothesized mean)
- H₁: μ ≠ μ₀ (The population mean is not equal to the hypothesized mean) (This is a two-tailed test. One-tailed tests (μ > μ₀ or μ < μ₀) are also possible.)
-
Determine the Significance Level (α): This is the probability of rejecting the null hypothesis when it is actually true (Type I error). Commonly, α = 0.05 is used.
-
Calculate the Test Statistic (z): z = (x̄ - μ₀) / (σ / √n) Where:
- x̄ is the sample mean
- μ₀ is the hypothesized population mean
- σ is the population standard deviation
- n is the sample size
-
Determine the Critical Value: Based on the significance level (α) and whether it's a one-tailed or two-tailed test, find the critical z-value from a z-table or statistical software.
-
Make a Decision:
- If the absolute value of the calculated z-statistic is greater than the critical z-value, reject the null hypothesis.
- If the absolute value of the calculated z-statistic is less than or equal to the critical z-value, fail to reject the null hypothesis.
-
Interpret the Results: State your conclusion in the context of the problem.
5. Interpreting Results:
The p-value is another crucial component of interpreting the results. The p-value represents the probability of observing the obtained results (or more extreme results) if the null hypothesis were true. A small p-value (typically less than α) provides strong evidence against the null hypothesis, leading to its rejection. A large p-value suggests that there is not enough evidence to reject the null hypothesis.
Closing Insights: Summarizing the Core Discussion
Z-tests provide a robust framework for making inferences about population means and proportions based on sample data. Understanding the different types of z-tests, their assumptions, and the steps involved in conducting and interpreting them is crucial for effective data analysis across various fields. Careful consideration of the assumptions and a clear interpretation of the results are essential for drawing valid and meaningful conclusions.
Exploring the Connection Between Sample Size and Z-Tests
The sample size (n) plays a significant role in the power and reliability of a z-test. A larger sample size generally leads to a smaller standard error, resulting in a more precise estimate of the population mean and increased power to detect a true difference. The Central Limit Theorem states that as the sample size increases, the sampling distribution of the sample mean approaches a normal distribution, regardless of the shape of the population distribution. This is why z-tests are often valid even when the population data isn't perfectly normally distributed, provided the sample size is sufficiently large.
Key Factors to Consider:
-
Roles and Real-World Examples: Larger sample sizes are crucial in studies requiring high precision, such as clinical trials or financial forecasting. Smaller sample sizes can lead to less reliable results, especially when dealing with populations exhibiting high variability.
-
Risks and Mitigations: A small sample size increases the risk of Type II error (failing to reject a false null hypothesis). This risk can be mitigated by increasing the sample size or using a more powerful statistical test.
-
Impact and Implications: The sample size directly impacts the confidence interval associated with the estimated population mean. Larger sample sizes produce narrower confidence intervals, indicating a more precise estimate.
Conclusion: Reinforcing the Connection
The relationship between sample size and the validity and power of a z-test is undeniable. Researchers and analysts should carefully consider the sample size when designing studies and interpreting results. Appropriate sample size calculation is critical to ensure the reliability and validity of z-test findings.
Further Analysis: Examining the Role of the Population Standard Deviation
The population standard deviation (σ) is another critical element in the z-test formula. Its accurate estimation is vital for obtaining reliable results. If the population standard deviation is unknown (which is usually the case in real-world scenarios), the t-test should be used instead. The t-test uses the sample standard deviation as an estimate of the population standard deviation, accounting for the additional uncertainty introduced by this estimation. The choice between a z-test and a t-test depends on whether the population standard deviation is known or unknown.
FAQ Section: Answering Common Questions About Z-Tests
Q: What is a z-test?
A: A z-test is a statistical test used to determine if there's a significant difference between a sample mean and a population mean (one-sample z-test) or between the means of two independent samples (two-sample z-test), or between two proportions. It assumes the data is normally distributed and the population standard deviation is known.
Q: When should I use a z-test versus a t-test?
A: Use a z-test when the population standard deviation is known. If the population standard deviation is unknown, use a t-test.
Q: What is a p-value, and how is it interpreted?
A: The p-value is the probability of obtaining results as extreme as, or more extreme than, the observed results, assuming the null hypothesis is true. A small p-value (typically less than 0.05) indicates strong evidence against the null hypothesis.
Q: What is the difference between a one-tailed and a two-tailed z-test?
A: A one-tailed z-test tests for an effect in a specific direction (e.g., greater than or less than). A two-tailed z-test tests for an effect in either direction.
Practical Tips: Maximizing the Benefits of Z-Tests
-
Verify Assumptions: Before conducting a z-test, ensure that the data meets the assumptions of normality and independence. Visual inspection of data through histograms or Q-Q plots can help assess normality.
-
Appropriate Sample Size: Ensure an adequate sample size to increase the power and reliability of the test.
-
Choose the Right Z-Test: Select the appropriate type of z-test based on the research question and the nature of the data.
-
Interpret Cautiously: Remember that statistical significance doesn't always imply practical significance. Consider the effect size and the context of the problem when interpreting the results.
Final Conclusion: Wrapping Up with Lasting Insights
The z-test is a fundamental tool in statistical inference. By understanding its principles, applications, and limitations, researchers and analysts can effectively utilize this powerful technique to draw meaningful conclusions from their data. Careful attention to assumptions, appropriate interpretation of results, and consideration of the practical implications are crucial for obtaining valid and insightful outcomes. The z-test, when applied correctly, provides a valuable pathway to data-driven decision-making in diverse fields.
Latest Posts
Latest Posts
-
How To Set Up Automatic Late Fees In Quickbooks Desktop
Apr 04, 2025
-
How To Charge Late Fees In Quickbooks
Apr 04, 2025
-
How To Apply Late Fees In Quickbooks
Apr 04, 2025
-
How To Set Up Automatic Late Fees In Quickbooks Online
Apr 04, 2025
-
How Do I Set Up Automatic Late Fees In Quickbooks Desktop
Apr 04, 2025
Related Post
Thank you for visiting our website which covers about Definition Z Test . We hope the information provided has been useful to you. Feel free to contact us if you have any questions or need further assistance. See you next time and don't miss to bookmark.