Sample Selection Bias Definition Examples And How To Avoid
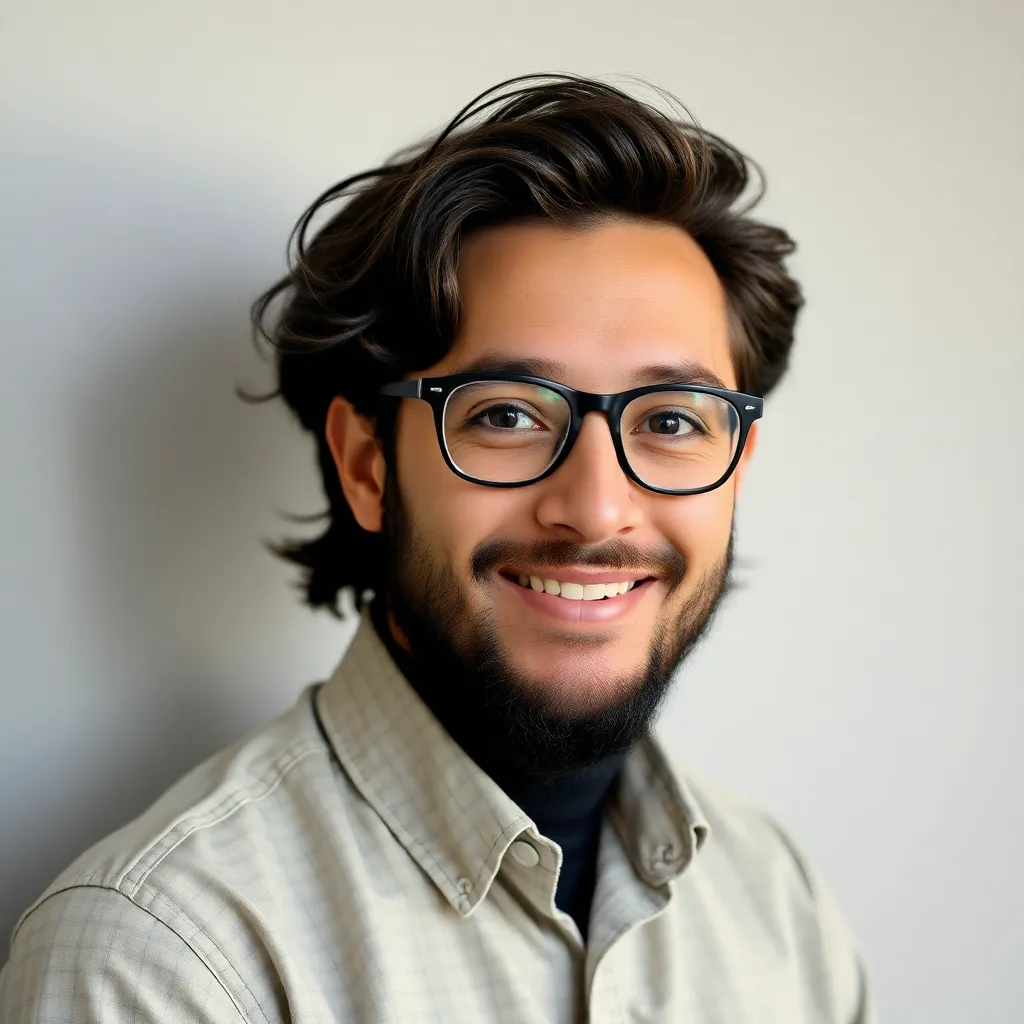
adminse
Apr 29, 2025 · 8 min read

Table of Contents
Unveiling the Perils of Sample Selection Bias: Definition, Examples, and Mitigation Strategies
What if the validity of your research hinges on the seemingly insignificant act of selecting a sample? Sample selection bias, a pervasive threat to research integrity, can subtly distort findings, leading to inaccurate conclusions and flawed decision-making.
Editor’s Note: This article on sample selection bias provides a comprehensive overview of this critical issue in research methodology. We delve into its definition, explore diverse examples across various fields, and offer practical strategies to minimize its impact on your research. The information presented here is intended to equip researchers and analysts with the tools necessary to conduct robust and reliable studies.
Why Sample Selection Bias Matters: Relevance, Practical Applications, and Industry Significance
Sample selection bias, a systematic error introduced during the selection process of a sample, profoundly impacts the generalizability and validity of research findings. Its implications span diverse fields, from medical research and clinical trials to market research and social science studies. Inaccurate data derived from biased samples can lead to misguided policy decisions, ineffective treatments, flawed product development, and misallocation of resources. Understanding and mitigating sample selection bias is paramount for maintaining research integrity and ensuring reliable results.
Overview: What This Article Covers
This article provides a detailed exploration of sample selection bias. It begins by defining the concept and then delves into diverse examples across various disciplines. We then examine the various types of sample selection bias, explore their underlying causes, and finally present practical strategies for prevention and mitigation. The article concludes with a FAQ section and actionable tips to improve sampling practices.
The Research and Effort Behind the Insights
This article synthesizes information from numerous peer-reviewed publications, textbooks on research methodology, and reputable online resources. The examples provided are drawn from real-world studies, highlighting the practical relevance of understanding and addressing sample selection bias. The insights are data-driven and supported by evidence from established research practices.
Key Takeaways:
- Definition and Core Concepts: A clear understanding of sample selection bias and its underlying mechanisms.
- Types and Causes: Identification of different forms of selection bias and their root causes.
- Examples Across Disciplines: Real-world illustrations of selection bias in various research areas.
- Mitigation Strategies: Practical techniques and best practices for preventing and minimizing selection bias.
- Actionable Steps: Specific steps researchers can take to enhance sampling procedures.
Smooth Transition to the Core Discussion:
Having established the importance of understanding sample selection bias, let's now delve into its core definition and explore its various manifestations.
Exploring the Key Aspects of Sample Selection Bias
Definition and Core Concepts:
Sample selection bias arises when the method used to select a sample for a study results in a sample that is not representative of the target population. This non-representativeness introduces systematic error, leading to skewed results and inaccurate conclusions. Essentially, the sample systematically differs from the population in ways relevant to the study’s objectives. This bias doesn't stem from random chance; it's a consequence of the sampling method itself.
Types and Causes of Sample Selection Bias:
Several types of sample selection bias exist, each stemming from different aspects of the sampling process:
- Self-selection bias: Participants volunteer for the study, leading to a non-representative sample. For instance, a survey on political views sent out online might attract more politically active individuals, skewing the results.
- Convenience sampling bias: Researchers select readily available participants, ignoring the broader population. This might involve surveying only students in a particular class, rather than a representative sample of the university population.
- Exclusion bias: Certain subgroups within the population are systematically excluded from the sample. This might occur if a health study excludes individuals with pre-existing conditions.
- Healthy worker effect: In occupational health studies, the sample might be skewed towards healthier individuals because those who are ill are less likely to participate.
- Attrition bias: Participants drop out of a longitudinal study, potentially leading to a sample that's systematically different from the original sample. Those who drop out might share characteristics that influence the study's results.
- Length-time bias: In retrospective studies examining disease incidence, the inclusion of longer-duration diseases can lead to a biased sample.
Examples Across Disciplines:
- Medical Research: A clinical trial investigating a new drug only includes patients from a specific hospital, potentially overlooking the diversity of patient populations.
- Market Research: A company surveys customer satisfaction only among those who actively use their website, ignoring feedback from customers who may have had negative experiences.
- Social Science: A study examining social attitudes uses a survey that is only accessible to individuals with internet access, excluding those without digital access.
- Environmental Science: A study of air pollution only samples air quality in one specific location, failing to represent the broader area's pollution levels.
Impact on Innovation:
Sample selection bias hinders innovation by leading to inaccurate insights. Inaccurate data may lead to the development of products or services that don't meet the needs of the target population, or to the implementation of policies that fail to achieve their intended goals.
Exploring the Connection Between Sample Size and Sample Selection Bias:
While sample size relates to the precision of estimates, sample selection bias fundamentally affects the accuracy of those estimates. A large sample size does not negate the impact of selection bias; a large biased sample will still produce inaccurate results.
Key Factors to Consider:
Roles and Real-World Examples: The role of sample selection bias in misleading results is illustrated in numerous studies. For example, a study on the effectiveness of a new teaching method that only uses students from high-achieving schools will likely overestimate the method's effectiveness.
Risks and Mitigations: The primary risk is the generation of unreliable and potentially harmful conclusions. Mitigation strategies include using probability sampling techniques, such as stratified random sampling or cluster sampling, to ensure representativeness.
Impact and Implications: The implications extend far beyond individual studies. Biased findings can influence policy decisions, resource allocation, and future research directions, potentially wasting resources and hindering progress.
Conclusion: Reinforcing the Connection:
The relationship between sample size and selection bias is crucial: a large sample size can increase the precision of estimates, but it cannot correct for bias. Addressing selection bias is paramount for ensuring the reliability and validity of research.
Further Analysis: Examining Probability Sampling Techniques in Greater Detail
Probability sampling methods, including simple random sampling, stratified random sampling, cluster sampling, and systematic sampling, are crucial for minimizing sample selection bias. These methods ensure that each member of the population has a known and non-zero probability of being selected, increasing the likelihood of obtaining a representative sample.
Simple Random Sampling: Every member of the population has an equal chance of being selected. This method, while straightforward, might not be effective if the population is highly heterogeneous.
Stratified Random Sampling: The population is divided into strata (subgroups) based on relevant characteristics, and random samples are drawn from each stratum. This ensures representation from all subgroups.
Cluster Sampling: The population is divided into clusters (groups), and a random sample of clusters is selected. All members within the selected clusters are then included in the sample. This method is efficient for large, geographically dispersed populations.
Systematic Sampling: Every kth member of the population is selected after a random starting point. This method is simple and easy to implement, but it can be susceptible to bias if there is a pattern in the population that aligns with the sampling interval.
FAQ Section: Answering Common Questions About Sample Selection Bias
What is sample selection bias? Sample selection bias occurs when the method of selecting a sample leads to a sample that is not representative of the population being studied.
How can sample selection bias be avoided? The primary method for avoiding sample selection bias is to use probability sampling techniques, which ensure every member of the population has a known chance of being included in the sample. Careful consideration of the study’s objectives and the characteristics of the target population is crucial in choosing the most appropriate sampling method.
What are the consequences of sample selection bias? Biased samples lead to inaccurate conclusions and flawed generalizations. This can have significant implications, especially in fields like healthcare and policy-making.
Can a large sample size eliminate sample selection bias? No, a large sample size increases precision but does not correct for bias. A large biased sample will still produce inaccurate results.
Practical Tips: Maximizing the Benefits of Robust Sampling
- Clearly Define the Target Population: Precisely identify the population you are studying to ensure your sample accurately reflects it.
- Choose the Appropriate Sampling Method: Select a sampling method that aligns with your research objectives and the characteristics of the population.
- Develop a Detailed Sampling Plan: Outline the steps involved in selecting your sample, including the method, sample size calculation, and procedures for data collection.
- Implement Quality Control Measures: Employ checks and balances throughout the sampling process to identify and correct any potential biases.
- Document Your Methodology: Thoroughly document your sampling procedures to ensure transparency and reproducibility.
Final Conclusion: Wrapping Up with Lasting Insights
Sample selection bias is a significant threat to the validity and generalizability of research findings. By understanding its various forms, causes, and mitigation strategies, researchers can significantly enhance the quality and reliability of their studies. Investing in robust sampling methods and meticulously planning the selection process are essential for producing accurate, insightful, and impactful research. The ultimate goal is to move beyond simply gathering data to obtaining data that truly reflects the reality being investigated.
Latest Posts
Latest Posts
-
Seasonal Industry Definition
Apr 29, 2025
-
Seasonal Credit Definition
Apr 29, 2025
-
Seasonal Adjustment Definition Calculation Methods Example
Apr 29, 2025
-
Sealed Bid Auction Definition How It Works In Real Estate Sales
Apr 29, 2025
-
What Is A Seagull Option Definition And How They Work In Trading
Apr 29, 2025
Related Post
Thank you for visiting our website which covers about Sample Selection Bias Definition Examples And How To Avoid . We hope the information provided has been useful to you. Feel free to contact us if you have any questions or need further assistance. See you next time and don't miss to bookmark.