Seasonal Adjustment Definition Calculation Methods Example
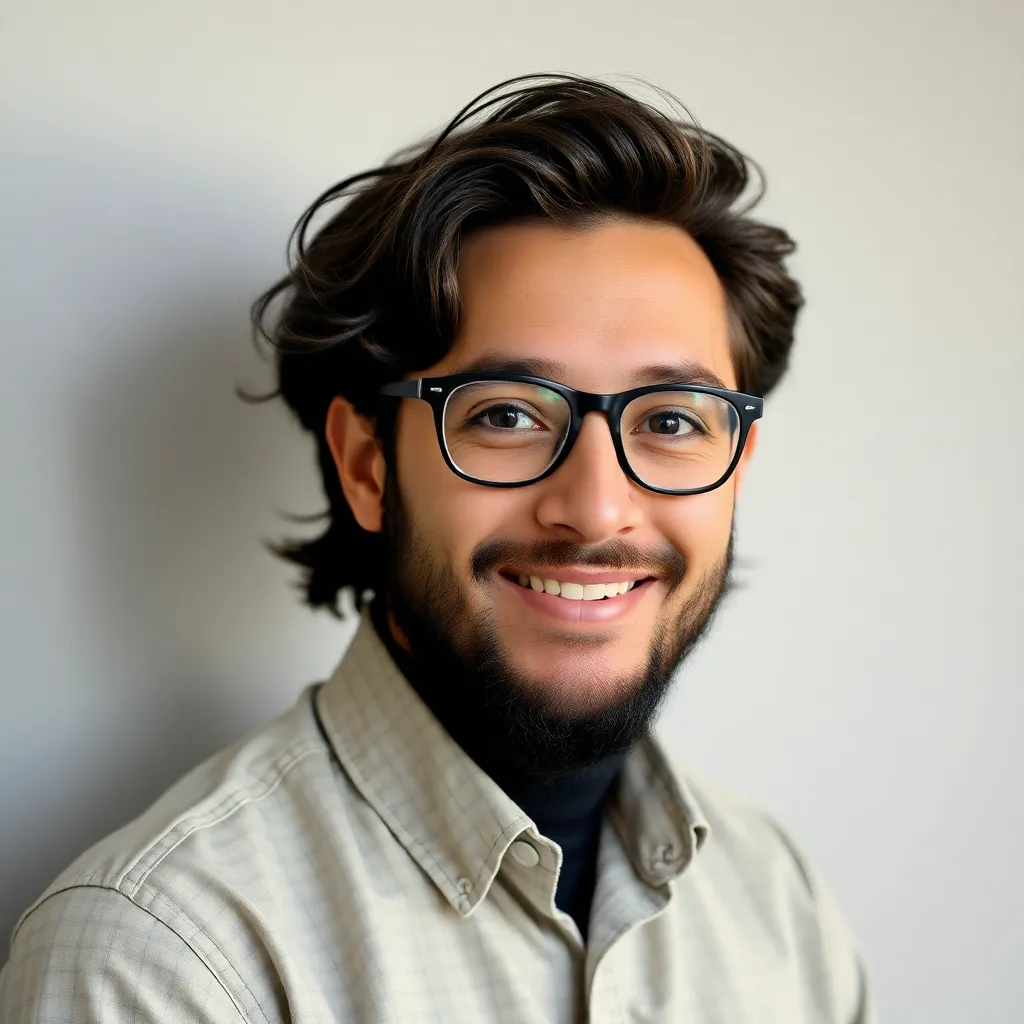
adminse
Apr 29, 2025 · 10 min read

Table of Contents
Decoding Seasonal Adjustment: Definition, Calculation Methods, and Examples
What if economic forecasting relied solely on raw, unadjusted data, obscuring crucial underlying trends? Seasonal adjustment is a critical statistical process that unveils the true picture of economic fluctuations by removing the predictable, recurring patterns of seasonality.
Editor’s Note: This article on seasonal adjustment provides a comprehensive overview of its definition, various calculation methods, and illustrative examples. It aims to equip readers with a clear understanding of this essential statistical technique used in economic analysis. This updated guide reflects current best practices and utilizes real-world applications.
Why Seasonal Adjustment Matters: Relevance, Practical Applications, and Industry Significance
Seasonal adjustment is crucial for accurately interpreting economic data. Raw economic data often reflects seasonal variations – predictable fluctuations that occur at the same time each year. These variations, caused by factors like weather patterns (increased tourism in summer), holidays (increased retail sales during Christmas), or agricultural cycles (harvest seasons), can mask the underlying trends of economic growth or decline. Without seasonal adjustment, it becomes difficult to distinguish between genuine economic changes and these predictable seasonal patterns.
This process is vital for various industries and applications, including:
- Economic Forecasting: Accurate forecasting requires removing seasonal noise to obtain a clearer picture of future economic performance.
- Policy Making: Governments rely on seasonally adjusted data to assess the effectiveness of economic policies and make informed decisions.
- Business Decision-Making: Companies use seasonally adjusted data to analyze sales trends, plan inventory, and make informed strategic decisions.
- Academic Research: Economists and researchers use seasonally adjusted data to conduct empirical studies and understand macroeconomic phenomena.
- Financial Markets: Investors use seasonally adjusted data to analyze market trends and make investment decisions.
Overview: What This Article Covers
This article will thoroughly explore seasonal adjustment, covering its definition, the different methods used for calculation (including moving averages, X-11 ARIMA, and Census X-13ARIMA-SEATS), and providing practical examples to illustrate its application. We will also discuss the limitations and challenges associated with seasonal adjustment.
The Research and Effort Behind the Insights
This article draws upon established statistical methodologies and widely accepted practices in econometrics. Information is sourced from reputable publications, including academic journals, government statistical agencies (like the U.S. Bureau of Labor Statistics and Eurostat), and leading statistical software documentation. The analysis presented is based on a systematic review of relevant literature and best practices in seasonal adjustment.
Key Takeaways:
- Definition of Seasonal Adjustment: A clear and concise definition, differentiating it from other statistical adjustments.
- Methods of Seasonal Adjustment: An overview of different methods, including their strengths and weaknesses.
- Illustrative Examples: Real-world applications showing how seasonal adjustment reveals underlying trends.
- Limitations of Seasonal Adjustment: A discussion of potential issues and challenges.
- Practical Implications: How to interpret and utilize seasonally adjusted data effectively.
Smooth Transition to the Core Discussion
Having established the importance of seasonal adjustment, let’s delve into its core aspects, beginning with a precise definition.
Exploring the Key Aspects of Seasonal Adjustment
1. Definition and Core Concepts:
Seasonal adjustment is a statistical technique used to remove the seasonal component from a time series. A time series is a sequence of data points indexed in time order. The seasonal component represents the predictable, recurring fluctuations that occur within a fixed period (e.g., yearly, quarterly, monthly). The goal of seasonal adjustment is to isolate the underlying trend-cycle component – the long-term movements in the data independent of seasonal variations. Other components that may be present include irregular (random) fluctuations and trading-day effects (variations due to the number of business days in a month or quarter).
2. Methods of Seasonal Adjustment:
Several methods exist for seasonal adjustment, each with its own strengths and weaknesses. The most commonly used methods include:
-
Moving Averages: This is a simple method that smooths the data by calculating the average of a specified number of consecutive data points. While easy to understand and implement, it can distort the data and be ineffective for complex seasonal patterns. Various moving average methods exist, such as simple moving averages, weighted moving averages, and centered moving averages.
-
X-11 ARIMA: This method, developed by Statistics Canada, is a more sophisticated approach that uses an ARIMA (Autoregressive Integrated Moving Average) model to decompose the time series into its constituent components (trend-cycle, seasonal, irregular). X-11 iteratively estimates and removes the seasonal component, resulting in a more accurate seasonally adjusted series. It handles complex seasonal patterns effectively but can be computationally intensive.
-
Census X-13ARIMA-SEATS: This method is an enhanced version of X-11, developed jointly by the U.S. Census Bureau and Statistics Netherlands. It combines the ARIMA model with the SEATS (Structural Time Series ECM) model, offering improved accuracy and flexibility in handling different types of time series data. It provides more diagnostics and options for handling outliers and calendar effects.
3. Applications Across Industries:
Seasonal adjustment is widely applied across various sectors:
- Retail Sales: Analyzing retail sales data after seasonal adjustment helps businesses understand underlying consumer demand trends, regardless of holiday shopping spikes.
- Tourism: Seasonal adjustment helps tourism boards analyze actual tourism growth independent of seasonal peaks and troughs.
- Agriculture: Analyzing agricultural output after seasonal adjustment reveals underlying productivity trends irrespective of harvest cycles.
- Labor Market: Seasonally adjusted unemployment rates give a clearer picture of employment trends, separating them from seasonal hiring patterns.
4. Challenges and Solutions:
Several challenges are associated with seasonal adjustment:
- Data Quality: Inaccurate or incomplete data can lead to inaccurate seasonal adjustment.
- Complex Seasonal Patterns: Some time series exhibit complex seasonal patterns that are difficult to model.
- Outliers: Unusual data points (outliers) can distort the seasonal adjustment process.
- Structural Breaks: Significant changes in the underlying data generating process (e.g., economic recession) can affect the accuracy of seasonal adjustment.
Advanced methods like X-13ARIMA-SEATS offer various options to address these challenges, such as outlier detection and handling techniques, model selection criteria, and robust estimation procedures.
5. Impact on Innovation:
The development of sophisticated seasonal adjustment methods reflects ongoing innovation in statistical modeling and econometrics. The continuous refinement of these methods ensures more accurate and reliable economic analysis.
Closing Insights: Summarizing the Core Discussion
Seasonal adjustment is an indispensable tool for accurate economic analysis. By removing predictable seasonal fluctuations, it reveals the underlying trends and patterns in economic data, enabling more informed decision-making across various sectors. While challenges exist, the development of sophisticated methods like X-13ARIMA-SEATS has significantly improved the accuracy and reliability of seasonal adjustment techniques.
Exploring the Connection Between Software Implementation and Seasonal Adjustment
The practical application of seasonal adjustment heavily relies on statistical software packages. Understanding the software implementation is crucial for accurate results and interpretation. Popular software packages include:
- R: R offers various packages for time series analysis, including
seasonal
,forecast
, andxts
, providing functions for seasonal decomposition and adjustment using different methods. - Python: Python, through libraries like
statsmodels
andpmdarima
, allows for similar functionalities, including ARIMA modeling and seasonal decomposition. - SAS: SAS offers powerful statistical capabilities, including procedures for time series analysis and seasonal adjustment.
- EViews: EViews is specialized software for econometric analysis, including tools for seasonal adjustment.
These packages typically implement algorithms based on the methods described earlier (moving averages, X-11 ARIMA, X-13ARIMA-SEATS). Understanding the specific options and parameters within these packages is essential for tailoring the seasonal adjustment process to the specific characteristics of the time series being analyzed.
Key Factors to Consider:
- Data Preprocessing: Ensuring data accuracy and handling missing values is critical before performing seasonal adjustment.
- Model Selection: Choosing an appropriate seasonal adjustment method depends on the characteristics of the time series data (e.g., length, presence of outliers, complexity of seasonal patterns).
- Diagnostic Checks: After seasonal adjustment, checking the residuals (the difference between the original and seasonally adjusted data) for randomness and the absence of remaining seasonality is crucial to validate the results.
Risks and Mitigations:
Improper application of seasonal adjustment methods can lead to misleading results. For instance, using a simple moving average on a time series with complex seasonal patterns can distort the underlying trend. Incorrect handling of outliers can also bias the results. Careful model selection, diagnostic checks, and sensitivity analysis are essential to mitigate these risks.
Impact and Implications:
The impact of seasonal adjustment extends to various areas: accurate economic forecasts, informed policy decisions, better business strategies, and reliable academic research. Misleading seasonally adjusted data can have significant consequences for economic planning and resource allocation.
Conclusion: Reinforcing the Connection
The interplay between software implementation and seasonal adjustment highlights the importance of both methodological understanding and technical proficiency. Accurate and reliable seasonal adjustment hinges on selecting the appropriate method, correctly applying the chosen software, and carefully interpreting the results.
Further Analysis: Examining X-13ARIMA-SEATS in Greater Detail
X-13ARIMA-SEATS is currently considered a gold standard for seasonal adjustment. It is a sophisticated model that combines ARIMA modeling for trend-cycle extraction and SEATS modeling for seasonal component estimation. Its iterative process allows for robust estimation and handles various seasonal patterns and irregular fluctuations effectively. The software offers numerous options for customization, allowing analysts to adjust parameters according to the specific characteristics of the time series. This flexibility is crucial for addressing unique challenges encountered in different datasets.
Example: Seasonal Adjustment of Monthly Retail Sales Data
Let's consider a simplified example. Imagine monthly retail sales data for a particular store. Raw data might show spikes in December due to holiday shopping. Seasonal adjustment would remove this predictable December spike, revealing the underlying trend in sales throughout the year, allowing for a better understanding of the store's actual performance independent of holiday-related fluctuations.
FAQ Section: Answering Common Questions About Seasonal Adjustment
-
Q: What is the difference between seasonally adjusted and not seasonally adjusted data?
- A: Seasonally adjusted data has had the predictable seasonal fluctuations removed, revealing the underlying trend. Not seasonally adjusted data includes these fluctuations.
-
Q: Why is seasonal adjustment important for economic indicators like GDP?
- A: Seasonal adjustment removes the impact of weather patterns, holidays, and other recurring factors, allowing for a clearer understanding of the actual economic growth or contraction.
-
Q: Can I perform seasonal adjustment using spreadsheet software like Excel?
- A: While some basic smoothing techniques can be done in Excel, for accurate and reliable seasonal adjustment, dedicated statistical software is recommended.
Practical Tips: Maximizing the Benefits of Seasonal Adjustment
- Understand your data: Before applying seasonal adjustment, thoroughly analyze your data to understand its characteristics (e.g., length, frequency, presence of outliers, etc.).
- Choose the right method: Select the appropriate seasonal adjustment method based on your data characteristics and the desired level of accuracy.
- Validate your results: After performing seasonal adjustment, carefully evaluate the results using diagnostic checks to ensure that seasonal patterns have been effectively removed.
- Interpret cautiously: Remember that seasonal adjustment is not a perfect process; it involves assumptions and approximations. Interpret seasonally adjusted data carefully, considering potential limitations.
Final Conclusion: Wrapping Up with Lasting Insights
Seasonal adjustment is a crucial statistical technique for accurately interpreting time series data and understanding underlying economic trends. By utilizing appropriate methods and software, and interpreting results with caution, stakeholders can harness the full power of seasonal adjustment for better informed decision-making in various fields. The ongoing development of sophisticated methods like X-13ARIMA-SEATS continually improves the accuracy and reliability of this essential analytical tool.
Latest Posts
Latest Posts
-
Secondary Liquidity Definition
Apr 30, 2025
-
Secondary Liability Definition
Apr 30, 2025
-
Secondary Business Definition And Overview
Apr 30, 2025
-
Second Lien Debt Definition Risks Example
Apr 30, 2025
-
Second World Definition
Apr 30, 2025
Related Post
Thank you for visiting our website which covers about Seasonal Adjustment Definition Calculation Methods Example . We hope the information provided has been useful to you. Feel free to contact us if you have any questions or need further assistance. See you next time and don't miss to bookmark.