Skewness Definition Simple
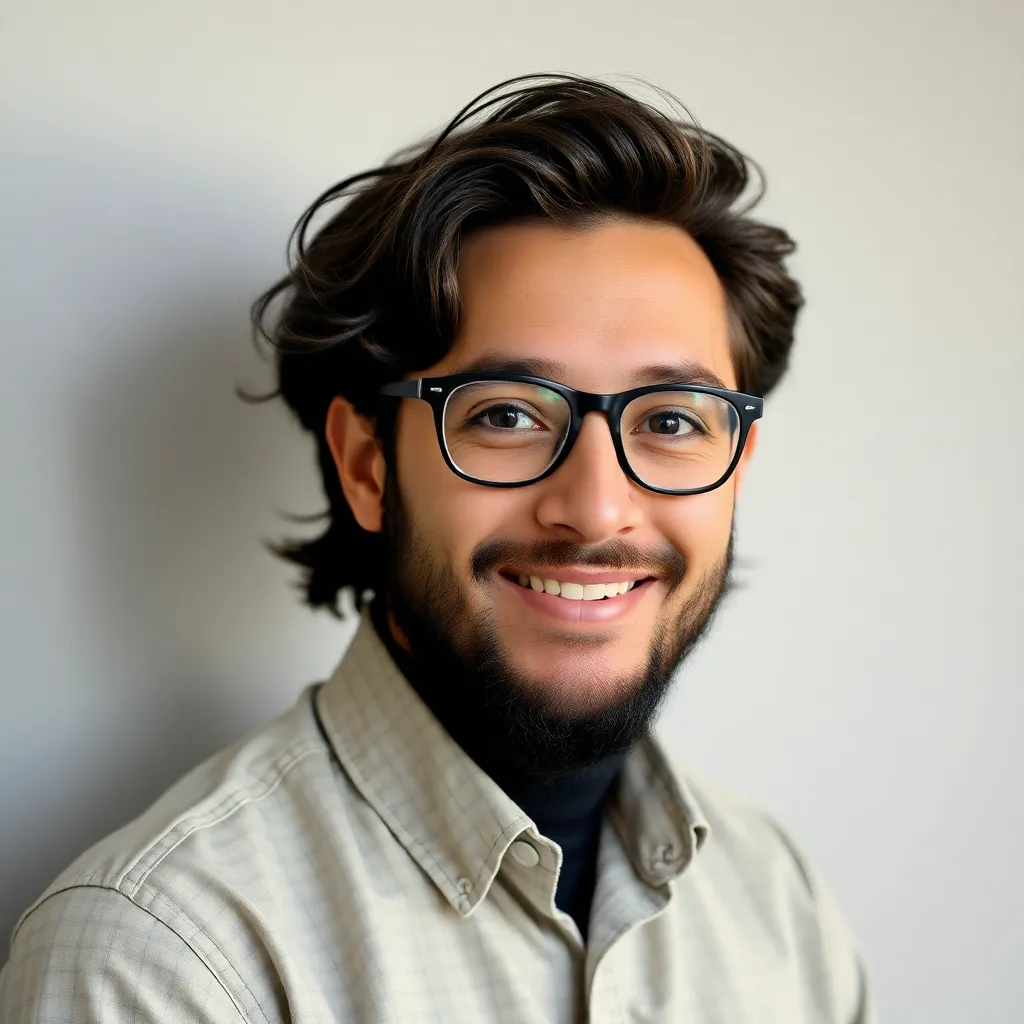
adminse
Mar 28, 2025 · 8 min read
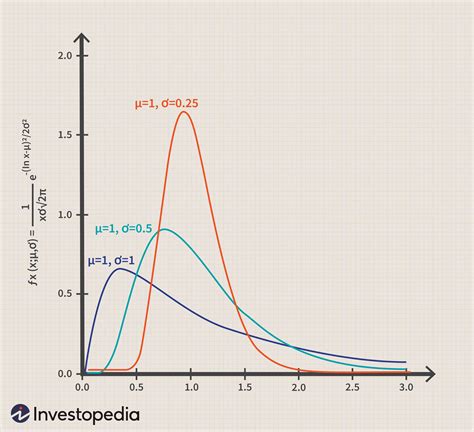
Table of Contents
Unmasking Skewness: A Simple Definition and Comprehensive Guide
What if understanding skewness unlocks a deeper understanding of data and its implications? This fundamental statistical concept is crucial for interpreting data accurately and making informed decisions.
Editor’s Note: This article on skewness provides a straightforward explanation, avoiding complex mathematical formulas. It's designed to be easily understandable for anyone working with data, regardless of their statistical background. Updated [Date of Publication].
Why Skewness Matters: Relevance, Practical Applications, and Industry Significance
Skewness is a critical concept in statistics because it reveals the shape of a data distribution. Unlike measures of central tendency (mean, median, mode) which describe the center, skewness describes the symmetry (or lack thereof). Understanding skewness is vital for:
- Accurate Data Interpretation: Skewed data can lead to misleading conclusions if interpreted without considering the distribution's shape.
- Informed Decision-Making: Knowing the skewness of data is crucial for selecting appropriate statistical tests and making reliable predictions.
- Risk Assessment: In finance, skewness helps assess the risk associated with investments, as highly skewed distributions can indicate potential for extreme losses or gains.
- Improved Data Analysis: Recognizing skewness allows for better data visualization and the selection of more appropriate statistical methods.
Overview: What This Article Covers
This article provides a comprehensive guide to skewness, starting with a simple definition and progressing to practical applications. We will explore:
- A clear and concise definition of skewness.
- Different types of skewness (positive, negative, and zero).
- How to visually identify skewness using histograms and box plots.
- The relationship between mean, median, and mode in skewed distributions.
- Practical applications of skewness in various fields.
- How to interpret skewness in real-world scenarios.
- Addressing common misconceptions about skewness.
The Research and Effort Behind the Insights
This article draws upon established statistical principles and widely accepted methodologies. The explanations are designed for clarity and accessibility, focusing on the practical interpretation of skewness rather than complex mathematical derivations. Examples are drawn from various fields to illustrate the real-world relevance of this crucial concept.
Key Takeaways:
- Definition: Skewness measures the asymmetry of a probability distribution.
- Types: Positive, negative, and zero skewness.
- Visual Identification: Histograms and box plots effectively show skewness.
- Mean, Median, Mode: Their relationship reveals the direction and degree of skewness.
- Applications: Wide-ranging applications across various fields.
Smooth Transition to the Core Discussion
Now that we understand the importance of skewness, let's delve into its core aspects, starting with a straightforward definition.
Exploring the Key Aspects of Skewness
Definition and Core Concepts:
Skewness, in simple terms, describes the lack of symmetry in a data distribution. A perfectly symmetrical distribution has a skewness of zero. Imagine a perfectly balanced bell curve – that's zero skewness. However, most real-world data sets are not perfectly symmetrical. They either lean to the left (negative skew) or to the right (positive skew).
Types of Skewness:
-
Positive Skewness (Right Skewed): The tail of the distribution extends more to the right. The mean is typically greater than the median, which is greater than the mode. Think of income distribution – most people earn a moderate income, but a few earn extremely high incomes, pulling the mean to the right.
-
Negative Skewness (Left Skewed): The tail of the distribution extends more to the left. The mean is typically less than the median, which is less than the mode. Consider test scores where most students score high, but a few score very low, pulling the mean to the left.
-
Zero Skewness (Symmetrical): The distribution is perfectly symmetrical. The mean, median, and mode are equal. This is ideal but rarely seen in real-world data.
Applications Across Industries:
Skewness finds application in diverse fields:
- Finance: Assessing investment risk, portfolio optimization. Highly positive skew can indicate high potential returns but also high risk.
- Healthcare: Analyzing health outcomes, identifying outliers in patient data.
- Marketing: Understanding customer behavior, preferences, and market trends.
- Engineering: Quality control, identifying defects in manufacturing processes.
- Environmental Science: Analyzing pollution levels, climate change data.
Challenges and Solutions:
One common challenge is interpreting skewness correctly. It's important to remember that skewness doesn't inherently indicate "good" or "bad" data. It simply describes the shape. The appropriate response depends on the context and the research question. For example, a positively skewed income distribution might be considered desirable by some, while a negatively skewed test score distribution might signal a need for improved teaching methods.
Impact on Innovation:
Understanding skewness leads to better statistical modeling, allowing for more accurate predictions and informed decision-making, driving innovation across many sectors.
Closing Insights: Summarizing the Core Discussion
Skewness is a fundamental statistical concept that helps us understand the shape of our data. It’s not just a theoretical measure; it has practical implications for data analysis, decision-making, and problem-solving across various fields. Recognizing and interpreting skewness allows for more robust analyses and more informed conclusions.
Exploring the Connection Between Visualizations and Skewness
Histograms and box plots are powerful visual tools for identifying skewness.
Key Factors to Consider:
Roles and Real-World Examples:
-
Histograms: A histogram is a bar graph showing the frequency distribution of a data set. A right-skewed histogram will have a longer tail extending to the right, and vice-versa for a left-skewed histogram. For example, a histogram of housing prices in a city might show positive skewness, with a few very expensive houses pulling the mean to the right.
-
Box Plots: Box plots display the median, quartiles, and potential outliers of a dataset. In a right-skewed distribution, the median will be closer to the bottom of the box, and the upper whisker will be longer. In a left-skewed distribution, the median will be closer to the top of the box, and the lower whisker will be longer. Consider a box plot representing student exam scores; a left skew would indicate many high scores and a few low ones.
Risks and Mitigations:
Misinterpreting skewness can lead to flawed conclusions. It's crucial to consider the context and the research question before drawing any inferences. For instance, high positive skewness in investment returns may appear attractive, but it's crucial to understand the associated risks.
Impact and Implications:
Using appropriate visualizations aids in readily identifying skewness, leading to more accurate interpretations and improved data-driven decision-making.
Conclusion: Reinforcing the Connection
Visualizations are essential tools for understanding and communicating skewness. Histograms and box plots provide an intuitive way to identify the direction and degree of skewness, allowing for a more comprehensive analysis of the data.
Further Analysis: Examining the Relationship Between Mean, Median, and Mode
In a symmetrical distribution, the mean, median, and mode are equal. However, in skewed distributions, their relationship reveals valuable information about the shape of the data.
- Positive Skewness: Mean > Median > Mode
- Negative Skewness: Mean < Median < Mode
Understanding this relationship helps confirm the presence and direction of skewness, enhancing the accuracy of interpretation.
FAQ Section: Answering Common Questions About Skewness
Q: What is the best way to measure skewness?
A: Several methods exist, including Pearson's moment coefficient of skewness and the median-based skewness coefficient. However, visual inspection using histograms and box plots often provides a quick and insightful assessment.
Q: Can skewness be negative and positive at the same time?
A: No. Skewness describes the overall asymmetry of the distribution. It can be positive (right-skewed), negative (left-skewed), or zero (symmetrical), but not both simultaneously.
Q: How does skewness affect statistical analysis?
A: Skewness can influence the choice of statistical tests and the interpretation of results. Some statistical tests assume a normal (symmetrical) distribution. If the data is heavily skewed, transformations may be necessary to meet these assumptions or alternative, non-parametric tests should be used.
Q: What if I have multiple peaks in my data?
A: This indicates multimodality, which is a different characteristic from skewness. Multimodal distributions have more than one mode (peak). Skewness still applies, describing the asymmetry even in a multimodal distribution.
Practical Tips: Maximizing the Benefits of Understanding Skewness
- Visualize Your Data: Always start by creating histograms and box plots to visually assess the distribution’s shape.
- Calculate Descriptive Statistics: Compute the mean, median, and mode to further confirm and quantify skewness.
- Consider Data Transformations: If your data is heavily skewed, consider transformations (e.g., logarithmic, square root) to make it more symmetrical for certain analyses.
- Choose Appropriate Statistical Tests: Select statistical methods appropriate for the shape of your data.
- Interpret Results Cautiously: Always consider the skewness of your data when interpreting results and drawing conclusions.
Final Conclusion: Wrapping Up with Lasting Insights
Understanding skewness is paramount for accurate data interpretation and informed decision-making. By learning to identify, quantify, and interpret skewness, you can enhance your data analysis skills and make more reliable inferences from your data. The concept of skewness, though seemingly simple, underpins many advanced statistical techniques and is a cornerstone of insightful data analysis. Its application extends far beyond the realm of statistics, influencing critical decisions across multiple industries and domains.
Latest Posts
Latest Posts
-
Letter To Credit Card Company To Remove Late Payment
Apr 03, 2025
-
How To Complain About Credit Card Charges
Apr 03, 2025
-
What Is The Maximum Late Fee Allowed By Law In Indiana
Apr 03, 2025
-
What Is The Maximum Late Fee Allowed By Law In Virginia
Apr 03, 2025
-
What Is The Maximum Late Fee Allowed By Law In Illinois
Apr 03, 2025
Related Post
Thank you for visiting our website which covers about Skewness Definition Simple . We hope the information provided has been useful to you. Feel free to contact us if you have any questions or need further assistance. See you next time and don't miss to bookmark.