Skewness Definition Math
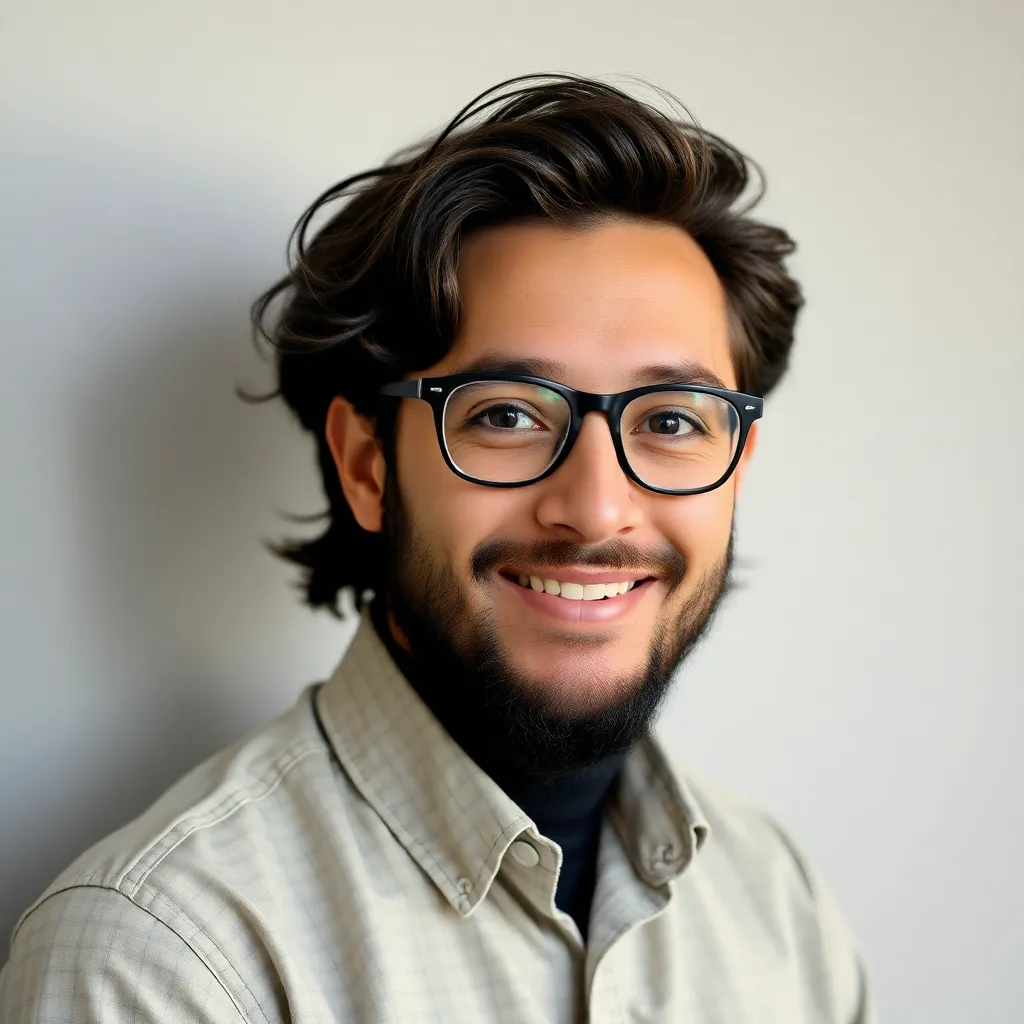
adminse
Mar 28, 2025 · 8 min read
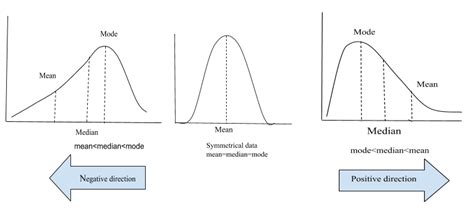
Table of Contents
Unveiling the Secrets of Skewness: A Deep Dive into its Mathematical Definition and Applications
What if the ability to understand the shape of a data distribution held the key to unlocking more accurate insights and predictions? Skewness, a fundamental statistical concept, provides precisely that power, revealing the asymmetry of a dataset and offering profound implications across numerous fields.
Editor’s Note: This article on skewness definition in math was published today, providing readers with the latest understanding of this crucial statistical concept and its diverse applications.
Why Skewness Matters: Beyond the Bell Curve
In the world of statistics, the normal distribution—often visualized as a symmetrical bell curve—serves as a benchmark. However, real-world data rarely adheres perfectly to this ideal. Skewness quantifies the departure from symmetry, revealing whether a distribution is skewed to the left (negatively skewed), symmetrical (zero skewness), or skewed to the right (positively skewed). Understanding skewness is crucial for accurately interpreting data, making informed decisions, and building robust statistical models. Its relevance spans diverse fields, including finance (analyzing risk and return), healthcare (studying disease prevalence), environmental science (modeling climate change impacts), and social sciences (understanding income inequality).
Overview: What This Article Covers
This comprehensive article delves into the mathematical definition of skewness, exploring different methods of calculation, interpreting its values, and understanding its implications. We'll examine the relationship between skewness and other statistical measures, discuss its applications in various fields, and address common misconceptions. Readers will gain a strong foundational understanding of skewness, equipping them to analyze data more effectively and draw meaningful conclusions.
The Research and Effort Behind the Insights
This article is the result of extensive research, drawing upon established statistical texts, peer-reviewed journals, and online resources. The explanations are grounded in mathematical rigor, while simultaneously prioritizing clarity and accessibility for a broad audience. Numerous examples and visualizations are used to reinforce understanding and illustrate key concepts.
Key Takeaways:
- Definition and Core Concepts: A precise mathematical definition of skewness and its underlying principles.
- Methods of Calculation: Exploring different approaches to calculating skewness, including Pearson’s moment coefficient of skewness and other methods.
- Interpreting Skewness Values: Understanding the meaning of positive, negative, and zero skewness values.
- Skewness and Other Statistical Measures: Examining the relationship between skewness, mean, median, and mode.
- Applications Across Disciplines: Exploring the use of skewness in diverse fields like finance, healthcare, and environmental science.
- Addressing Common Misconceptions: Clarifying common misunderstandings surrounding skewness and its interpretation.
Smooth Transition to the Core Discussion
Having established the importance of skewness, let's now delve into the mathematical intricacies and practical applications of this vital statistical concept.
Exploring the Key Aspects of Skewness
1. Definition and Core Concepts:
Skewness measures the asymmetry of a probability distribution. A perfectly symmetrical distribution, like the normal distribution, has a skewness of zero. A distribution skewed to the right (positively skewed) has a longer tail extending to the right, while a distribution skewed to the left (negatively skewed) has a longer tail extending to the left. This asymmetry indicates that the data points are not evenly distributed around the mean.
2. Methods of Calculation:
Several methods exist for calculating skewness. The most common is Pearson's moment coefficient of skewness, which is defined as:
Skewness = 3 * (Mean - Median) / Standard Deviation
This formula leverages the relationship between the mean, median, and standard deviation to quantify the asymmetry. A positive value indicates right skewness, a negative value indicates left skewness, and a value close to zero suggests symmetry.
Another approach involves calculating the Fisher-Pearson standardized moment coefficient:
Skewness = [∑(xᵢ - μ)³ / n] / σ³
Where:
- xᵢ represents individual data points
- μ represents the mean
- n represents the sample size
- σ represents the standard deviation
This method uses the third standardized moment of the distribution. The higher the absolute value of this coefficient, the greater the skewness. Note that this formula is sensitive to outliers, as cubed deviations amplify the effect of extreme values.
Other methods, less frequently used, include the quartile coefficient of skewness and Bowley's coefficient of skewness.
3. Interpreting Skewness Values:
The interpretation of skewness values depends on the specific method used and the context of the data. However, some general guidelines apply:
- Positive Skewness (Right Skewness): The mean is greater than the median, indicating a long tail extending to the right. This is often observed in income distributions, where a few high earners significantly impact the mean.
- Negative Skewness (Left Skewness): The mean is less than the median, indicating a long tail extending to the left. This can be seen in test scores where most students perform well, but a few score poorly, pulling the mean downwards.
- Zero Skewness (Symmetry): The mean and median are approximately equal, suggesting a symmetrical distribution.
4. Skewness and Other Statistical Measures:
Skewness is closely related to the mean, median, and mode. In a perfectly symmetrical distribution, these three measures coincide. However, in skewed distributions, they diverge. The relationship between these measures provides further insights into the nature of the asymmetry. For instance, in a positively skewed distribution, the mean > median > mode, while in a negatively skewed distribution, the mode > median > mean.
5. Applications Across Disciplines:
The applications of skewness are vast and far-reaching:
- Finance: Analyzing investment returns, assessing risk, and modeling market fluctuations. Positively skewed returns might indicate higher potential gains but also higher risks.
- Healthcare: Studying disease prevalence, analyzing health outcomes, and understanding the distribution of medical costs. Skewed distributions might highlight disparities in healthcare access or outcomes.
- Environmental Science: Modeling climate change impacts, analyzing pollution levels, and understanding the distribution of natural resources.
- Social Sciences: Understanding income inequality, analyzing educational attainment, and studying social mobility.
- Engineering: Assessing the reliability of systems, analyzing failure rates, and optimizing designs.
6. Addressing Common Misconceptions:
- Skewness and Normality: Skewness is not directly indicative of whether a distribution is normal or not. A normal distribution has zero skewness, but a distribution with zero skewness is not necessarily normal.
- Magnitude of Skewness: The magnitude of the skewness coefficient doesn't always directly translate to the "degree" of asymmetry in a visually intuitive way. Context and the specific calculation method are crucial.
- Causation vs. Correlation: Skewness describes the shape of a distribution but doesn't explain the underlying causes of that shape.
Exploring the Connection Between Outliers and Skewness
Outliers, extreme values significantly different from the rest of the data, have a profound impact on skewness. The presence of outliers can artificially inflate or deflate skewness calculations, leading to misinterpretations.
Key Factors to Consider:
- Roles and Real-World Examples: Outliers, particularly in small datasets, can disproportionately influence the mean and thus skew the skewness calculation. Consider an example of house prices: a few extremely expensive mansions can drastically skew the distribution to the right.
- Risks and Mitigations: Robust measures of skewness, less sensitive to outliers, might be preferable when dealing with data containing potential outliers. Data cleaning techniques, such as identifying and removing or transforming outliers, should be considered cautiously.
- Impact and Implications: Misinterpreting skewness due to outliers can lead to inaccurate conclusions and flawed decision-making. For instance, relying on a skewed skewness value to make inferences about investment returns could lead to suboptimal investment strategies.
Conclusion: Reinforcing the Connection
The relationship between outliers and skewness is crucial for accurate data analysis. Understanding how outliers impact skewness calculations is essential for making informed decisions and avoiding misinterpretations. Robust methods and careful data handling are crucial when dealing with potential outliers.
Further Analysis: Examining Outliers in Greater Detail
Identifying and handling outliers requires a deeper understanding of their potential sources. Are they due to measurement errors, data entry mistakes, or genuinely extreme values reflecting a characteristic of the underlying population? Different approaches to handling outliers exist, including trimming (removing extreme values), Winsorizing (replacing extreme values with less extreme values), and using robust statistical methods that are less sensitive to outliers.
FAQ Section: Answering Common Questions About Skewness
Q: What is skewness?
A: Skewness is a measure of the asymmetry of a probability distribution. It quantifies how much a distribution deviates from a symmetrical bell curve.
Q: How is skewness calculated?
A: Several methods exist, including Pearson's moment coefficient of skewness and Fisher-Pearson standardized moment coefficient. The choice of method depends on the characteristics of the data and the research goals.
Q: What do positive and negative skewness values mean?
A: Positive skewness indicates a right-skewed distribution with a long tail to the right. Negative skewness indicates a left-skewed distribution with a long tail to the left.
Q: How does skewness affect statistical analysis?
A: Skewness can affect the interpretation of statistical measures like the mean and standard deviation. It's essential to consider skewness when choosing appropriate statistical tests and making inferences from data.
Q: Can skewness be used in different fields?
A: Yes, skewness finds applications in finance, healthcare, environmental science, social sciences, and various other fields.
Practical Tips: Maximizing the Benefits of Understanding Skewness
-
Visualize your data: Always start by creating histograms or box plots to visualize the distribution and get a preliminary sense of skewness.
-
Calculate skewness: Use appropriate methods based on data characteristics and choose between Pearson's moment coefficient or the Fisher-Pearson standardized moment coefficient.
-
Consider outliers: Examine your data for outliers and assess their potential impact on skewness. Decide on appropriate handling strategies.
-
Interpret in context: Don't just focus on the numerical value of skewness. Interpret it in the context of your specific dataset and research question.
-
Choose appropriate statistical methods: Select statistical methods that are appropriate for skewed data if necessary.
Final Conclusion: Wrapping Up with Lasting Insights
Skewness is a fundamental statistical concept with far-reaching applications. Understanding its mathematical definition, calculation methods, and interpretation is crucial for accurate data analysis and informed decision-making. By carefully considering skewness and addressing potential issues like outliers, researchers and analysts can extract deeper insights from their data and make more robust conclusions. The ability to understand and interpret skewness empowers individuals to navigate the complexities of data analysis with greater confidence and precision.
Latest Posts
Latest Posts
-
Letter To Credit Card Company To Remove Late Payment
Apr 03, 2025
-
How To Complain About Credit Card Charges
Apr 03, 2025
-
What Is The Maximum Late Fee Allowed By Law In Indiana
Apr 03, 2025
-
What Is The Maximum Late Fee Allowed By Law In Virginia
Apr 03, 2025
-
What Is The Maximum Late Fee Allowed By Law In Illinois
Apr 03, 2025
Related Post
Thank you for visiting our website which covers about Skewness Definition Math . We hope the information provided has been useful to you. Feel free to contact us if you have any questions or need further assistance. See you next time and don't miss to bookmark.