Sampling Errors In Statistics Definition Types And Calculation
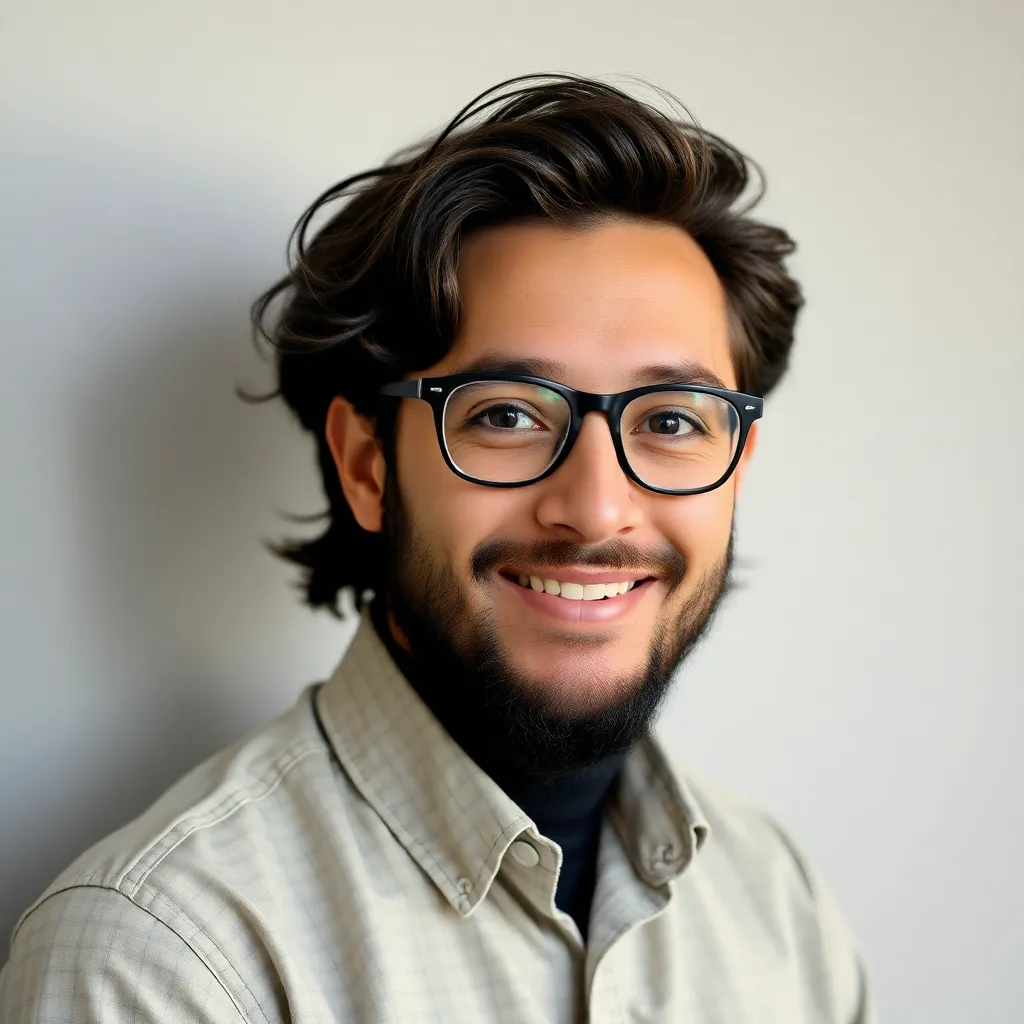
adminse
Apr 29, 2025 · 10 min read

Table of Contents
Unveiling the Uncertainty: Sampling Errors in Statistics – Definition, Types, and Calculation
What if the accuracy of our conclusions hinges on understanding sampling errors? These seemingly small discrepancies can significantly impact the validity of statistical inferences, leading to flawed decisions across numerous fields.
Editor’s Note: This article on sampling errors in statistics provides a comprehensive overview of their definition, types, and calculation methods. It aims to equip readers with a solid understanding of this critical concept, enabling them to interpret statistical results more critically and make informed decisions.
Why Sampling Errors Matter: Relevance, Practical Applications, and Industry Significance
Sampling error is an unavoidable aspect of statistical analysis whenever a sample is used to represent a larger population. It represents the difference between the statistic obtained from the sample and the true population parameter. Understanding sampling errors is crucial for various reasons. In fields like market research, political polling, medical research, and quality control, inaccurate estimations due to sampling errors can lead to flawed conclusions, wasted resources, and even harmful consequences. The implications extend far beyond simple miscalculations; they impact the reliability of predictions, the effectiveness of strategies, and the overall validity of research findings. The ability to quantify and minimize sampling error is a cornerstone of robust statistical analysis.
Overview: What This Article Covers
This article will provide a detailed exploration of sampling errors. We will delve into the definition, explore various types of sampling errors, and examine the methods used to calculate and reduce their impact. We will further analyze the relationship between sample size, sampling methods, and the magnitude of sampling errors. By the end, you will possess the knowledge to better understand and interpret the limitations of statistical inferences based on samples.
The Research and Effort Behind the Insights
This article draws upon established statistical principles, textbooks, and research papers to ensure accuracy and credibility. The information presented reflects widely accepted methodologies and is supported by numerous peer-reviewed publications. A structured approach has been adopted to present complex concepts in a clear and accessible manner.
Key Takeaways:
- Definition and Core Concepts: A precise definition of sampling error and its core components.
- Types of Sampling Errors: A detailed examination of different types of sampling errors, including random and non-random errors.
- Calculating Sampling Error: Methods for estimating the magnitude of sampling error for various statistics (e.g., mean, proportion).
- Minimizing Sampling Error: Strategies to reduce the impact of sampling errors, including increasing sample size and employing appropriate sampling techniques.
- Interpreting Results: Guidance on how to interpret statistical results in light of potential sampling errors.
Smooth Transition to the Core Discussion
Having established the significance of understanding sampling errors, let's now delve into a detailed examination of its definition, types, and calculation methods.
Exploring the Key Aspects of Sampling Errors
1. Definition and Core Concepts:
Sampling error arises from the inherent randomness of selecting a subset (sample) from a larger population. Because a sample is only a part of the whole, it will almost never perfectly represent the population. This discrepancy between the sample statistic (e.g., sample mean, sample proportion) and the true population parameter (e.g., population mean, population proportion) constitutes the sampling error. It’s important to note that sampling error is not a mistake; it's a natural consequence of sampling.
2. Types of Sampling Errors:
Sampling errors can be broadly classified into two main categories:
-
Random Sampling Error: This type of error occurs purely due to chance. Even with a perfectly designed sampling method, random variation in selecting individuals for the sample will inevitably lead to some difference between the sample statistic and the population parameter. This error is inherently unpredictable and unavoidable. However, its magnitude can be estimated using statistical methods and probability theory.
-
Non-Random Sampling Error (Bias): This type of error is introduced due to flaws in the sampling design or the sampling process itself. These flaws can systematically skew the results, leading to a sample that does not accurately reflect the population. Several sources of non-random sampling error exist:
- Selection Bias: Occurs when the selection process favors certain individuals or groups over others, leading to an unrepresentative sample. For example, using a phone survey might exclude individuals without phones.
- Non-response Bias: Occurs when a significant portion of selected individuals do not respond to the survey. This can lead to bias if non-respondents differ systematically from respondents.
- Measurement Bias: Occurs when the method used to collect data is flawed, leading to inaccurate or inconsistent measurements. For example, poorly worded survey questions can lead to biased responses.
3. Calculating Sampling Error:
The calculation of sampling error depends on the statistic of interest and the sampling distribution of that statistic. The most common calculations involve:
-
Sampling Error of the Mean: The sampling error of the mean is estimated using the standard error of the mean (SEM). The SEM is calculated as the standard deviation of the sample divided by the square root of the sample size:
SEM = σ/√n (where σ is the population standard deviation and n is the sample size)
If the population standard deviation (σ) is unknown (which is usually the case), the sample standard deviation (s) is used as an estimate:
SEM ≈ s/√n
-
Sampling Error of the Proportion: The sampling error of a proportion is estimated using the standard error of the proportion (SEP). The SEP is calculated as:
SEP = √[p(1-p)/n] (where p is the sample proportion and n is the sample size)
4. Minimizing Sampling Error:
Several strategies can be employed to minimize sampling error:
-
Increasing Sample Size: A larger sample size generally leads to a smaller sampling error because it provides a more accurate representation of the population. The relationship is not linear, however; the reduction in error diminishes with increasing sample size.
-
Using Appropriate Sampling Techniques: Employing probability sampling methods (such as simple random sampling, stratified sampling, cluster sampling) ensures that every member of the population has a known probability of being selected, reducing the risk of bias.
-
Careful Data Collection: Implementing rigorous data collection procedures, including well-designed questionnaires and trained interviewers, helps to minimize measurement error and non-response bias.
-
Stratification: Dividing the population into relevant subgroups (strata) and sampling from each stratum proportionally reduces the impact of variations within the population.
-
Pilot Studies: Conducting a small-scale pilot study before the main study allows for testing the methodology and identifying potential sources of error.
5. Impact on Innovation:
Understanding and managing sampling error is vital for innovation across numerous fields. Inaccurate statistical inferences due to sampling errors can lead to the development of ineffective products, inefficient processes, or misleading marketing campaigns. Accurate sampling and subsequent analysis are crucial for evidence-based decision-making that underpins innovation.
Closing Insights: Summarizing the Core Discussion
Sampling error is an inherent aspect of using samples to infer population characteristics. By understanding the different types of sampling errors and the methods for estimating and minimizing them, researchers and decision-makers can improve the accuracy and reliability of their statistical analyses. Minimizing bias and maximizing the precision of estimates are crucial steps in ensuring that inferences drawn from samples accurately reflect the population.
Exploring the Connection Between Sample Size and Sampling Error
The relationship between sample size and sampling error is inversely proportional. As the sample size (n) increases, the standard error (both SEM and SEP) decreases. This is because a larger sample provides a more precise estimate of the population parameter. The square root in the formulas for SEM and SEP highlights this relationship; doubling the sample size reduces the standard error by a factor of √2 (approximately 1.414). However, the reduction in error diminishes with increasingly larger sample sizes; obtaining perfect accuracy would require a sample size equal to the entire population. Determining the optimal sample size is a crucial aspect of statistical planning, often involving considerations of cost, time, and the desired level of precision.
Key Factors to Consider:
-
Roles and Real-World Examples: A larger sample size is critical in medical research where small errors can have significant implications. In contrast, smaller sample sizes may suffice in some exploratory research where perfect accuracy is less critical.
-
Risks and Mitigations: Using a small sample size increases the risk of obtaining misleading results due to larger sampling error. Mitigations include employing more robust statistical methods or carefully considering the implications of higher uncertainty.
-
Impact and Implications: The choice of sample size has a profound impact on the precision and reliability of statistical inferences. Underpowered studies (too small a sample size) can fail to detect true effects, while overly large samples may be wasteful and unnecessary.
Conclusion: Reinforcing the Connection
The relationship between sample size and sampling error is fundamental to the practice of statistics. Careful consideration of sample size is essential in planning research, ensuring sufficient precision while managing costs and resources. The choice of sample size directly impacts the reliability of statistical conclusions, making it a critical decision in any statistical study.
Further Analysis: Examining Sample Size Determination in Greater Detail
The determination of appropriate sample size is a complex process influenced by multiple factors, including the desired level of confidence, the acceptable margin of error, the estimated population variability, and the type of statistical test to be employed. Power analysis is a statistical technique commonly used to determine the minimum sample size required to detect a statistically significant effect with a specific level of power. Power analysis considers factors such as the significance level (alpha), the desired power (1-beta), and the effect size. Statistical software packages and online calculators can be used to perform power analysis and determine an appropriate sample size for a given study design.
FAQ Section: Answering Common Questions About Sampling Errors
Q: What is the difference between sampling error and bias?
A: Sampling error is the natural variation resulting from using a sample to represent a population, while bias is a systematic error introduced by flaws in the sampling design or data collection process. Sampling error is random, while bias is non-random.
Q: How can I reduce non-random sampling error?
A: Careful planning of the sampling methodology is crucial. This involves using appropriate probability sampling techniques, developing well-designed questionnaires, training data collectors thoroughly, and addressing potential sources of bias during data collection.
Q: Is it possible to completely eliminate sampling error?
A: No, it is not possible to completely eliminate sampling error. Sampling error is inherent in using samples to represent populations. The goal is to minimize it through appropriate sampling techniques and sufficiently large sample sizes.
Q: What should I do if my sampling error is too large?
A: If the sampling error is unacceptably large, the study may need to be redesigned. This could involve increasing the sample size, employing more effective sampling methods, improving data collection techniques, or refining the study design.
Practical Tips: Maximizing the Benefits of Understanding Sampling Errors
-
Understand the Basics: Begin by grasping the fundamental concepts of sampling error, its types, and its implications for statistical inference.
-
Choose the Right Sampling Method: Select a sampling method appropriate for your research question and population. Consider stratified, cluster, or other techniques to address potential biases.
-
Calculate Sample Size Carefully: Use power analysis or other methods to determine an appropriate sample size that balances precision with cost and feasibility.
-
Document Your Methods: Meticulously document your sampling methods, including the population definition, sampling frame, and selection process. Transparency is crucial for evaluating the validity of your findings.
-
Report Sampling Error: Clearly report the sampling error associated with your estimates. This provides crucial information for interpreting the results and understanding their limitations.
Final Conclusion: Wrapping Up with Lasting Insights
Sampling errors are an unavoidable aspect of statistical analysis based on samples. However, understanding their nature, types, and methods for calculation and minimization is essential for conducting rigorous and meaningful research. By carefully planning sample designs, employing appropriate sampling techniques, and utilizing large enough samples, the influence of sampling errors can be significantly reduced, resulting in more accurate and reliable statistical inferences. The principles discussed here are applicable across a vast range of disciplines, contributing to better decision-making and more robust conclusions in various fields.
Latest Posts
Latest Posts
-
Seasonal Industry Definition
Apr 29, 2025
-
Seasonal Credit Definition
Apr 29, 2025
-
Seasonal Adjustment Definition Calculation Methods Example
Apr 29, 2025
-
Sealed Bid Auction Definition How It Works In Real Estate Sales
Apr 29, 2025
-
What Is A Seagull Option Definition And How They Work In Trading
Apr 29, 2025
Related Post
Thank you for visiting our website which covers about Sampling Errors In Statistics Definition Types And Calculation . We hope the information provided has been useful to you. Feel free to contact us if you have any questions or need further assistance. See you next time and don't miss to bookmark.