Sample Distribution Definition How Its Used With An Example
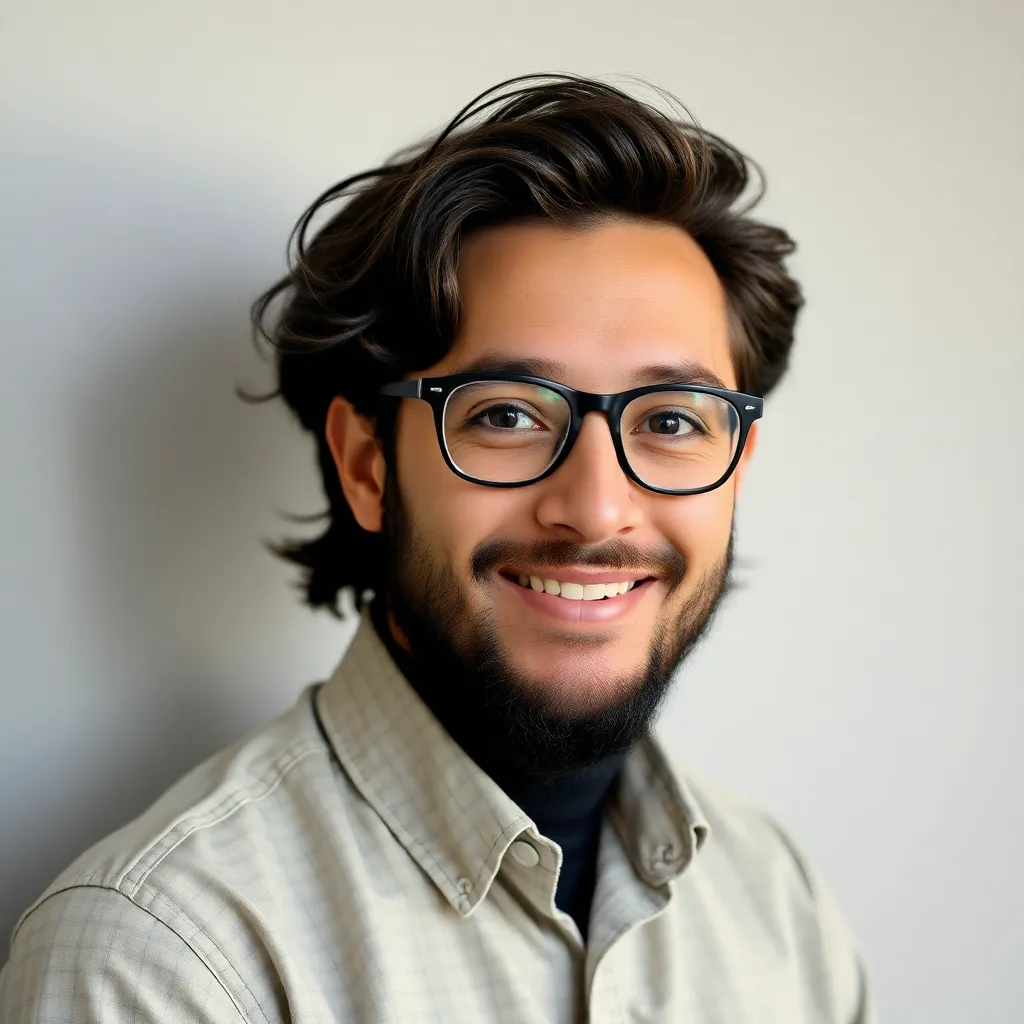
adminse
Apr 29, 2025 · 9 min read

Table of Contents
Decoding Sample Distribution: How It Shapes Data Analysis and Decision-Making
What if the accuracy of your conclusions hinges on how you distribute your samples? Understanding sample distribution is paramount for deriving reliable insights from data and making informed decisions.
Editor’s Note: This article on sample distribution was published today, providing readers with current and relevant information on this crucial statistical concept. It aims to demystify sample distribution, providing clear explanations, practical examples, and actionable insights.
Why Sample Distribution Matters:
Sample distribution is the cornerstone of statistical inference. It's the foundation upon which researchers build their understanding of populations, allowing them to draw conclusions about a larger group based on a smaller, representative subset. Its relevance spans numerous fields, including market research, healthcare, engineering, and social sciences. Understanding how samples are distributed allows for accurate estimations of population parameters like mean, median, and standard deviation, enabling more precise predictions and more effective decision-making. Inaccurate or biased sampling leads to flawed conclusions and potentially costly mistakes.
Overview: What This Article Covers:
This article delves into the core concept of sample distribution, exploring its definition, different types, its crucial role in various applications, and the potential pitfalls of improper sampling. Readers will gain a practical understanding, supported by examples and case studies, allowing for effective application in their respective fields.
The Research and Effort Behind the Insights:
This article is the result of extensive research, drawing upon established statistical principles, published studies, and real-world examples from diverse industries. The information presented is supported by credible sources, ensuring accuracy and providing readers with trustworthy insights.
Key Takeaways:
- Definition and Core Concepts: A clear explanation of sample distribution and its underlying principles.
- Types of Sample Distributions: Exploration of different distributions, including normal, binomial, and Poisson distributions, and when each is appropriate.
- Applications Across Industries: Examples of how sample distribution is used in various sectors.
- Challenges and Solutions: Identification of common pitfalls in sample distribution and strategies to mitigate them.
- Impact on Data Analysis: How sample distribution affects the validity and reliability of statistical conclusions.
Smooth Transition to the Core Discussion:
With an understanding of the importance of sample distribution, let's now explore its core aspects in detail, beginning with a formal definition.
Exploring the Key Aspects of Sample Distribution:
1. Definition and Core Concepts:
Sample distribution refers to the probability distribution of a statistic obtained from a random sample of a population. Instead of focusing on individual data points, sample distribution examines the distribution of a specific statistic (like the mean, median, or variance) calculated from numerous random samples drawn from the same population. This distribution provides insight into the variability of the statistic and allows for estimations about the population parameter. Crucially, the sample distribution is different from the population distribution; it describes the behavior of a sample statistic, not the individual data points within the population.
2. Types of Sample Distributions:
The shape and characteristics of the sample distribution depend on several factors, including the size of the sample and the distribution of the population itself. Some common sample distributions include:
-
Normal Distribution: This bell-shaped curve is the most commonly encountered distribution. The Central Limit Theorem states that the distribution of sample means will approximate a normal distribution, regardless of the population distribution's shape, as the sample size increases (generally above 30). This makes the normal distribution incredibly useful for statistical inference.
-
Binomial Distribution: Used when the outcome of an experiment is binary (success or failure), with a fixed number of independent trials and a constant probability of success for each trial. For instance, flipping a coin 10 times and counting the number of heads follows a binomial distribution.
-
Poisson Distribution: Models the probability of a given number of events occurring in a fixed interval of time or space, given an average rate of occurrence. This is used in scenarios like counting the number of cars passing a point on a highway per hour.
-
t-Distribution: Similar to the normal distribution, but used when the population standard deviation is unknown and must be estimated from the sample. The t-distribution has heavier tails than the normal distribution, reflecting the increased uncertainty.
3. Applications Across Industries:
Sample distribution finds extensive application across various domains:
-
Market Research: Determining consumer preferences, predicting market trends, and assessing the effectiveness of marketing campaigns rely heavily on sample distributions. Surveys and polls use samples to represent the broader population.
-
Healthcare: Clinical trials use sample distributions to determine the effectiveness of new drugs or treatments. By comparing treatment and control groups, researchers assess the statistical significance of observed differences.
-
Quality Control: In manufacturing, sample distribution helps assess the quality of products by randomly selecting items and analyzing their characteristics. This allows manufacturers to identify and rectify production issues.
-
Environmental Science: Studying pollution levels, analyzing wildlife populations, and monitoring climate change often involve collecting samples and examining their distributions.
-
Social Sciences: Researchers use sample distributions to draw conclusions about social behaviors, attitudes, and trends.
4. Challenges and Solutions:
Several challenges can arise when dealing with sample distributions:
-
Sampling Bias: This occurs when the sample is not representative of the population, leading to inaccurate conclusions. Strategies to mitigate this include using random sampling techniques, stratified sampling (ensuring representation from different subgroups), and cluster sampling (sampling groups within the population).
-
Small Sample Size: Small samples can lead to highly variable sample statistics and inaccurate estimations of population parameters. Increasing the sample size improves the accuracy and reliability of the results.
-
Non-Normality: When the sample distribution significantly deviates from normality, certain statistical tests may not be applicable. Transformations of the data (e.g., logarithmic transformation) or the use of non-parametric tests can address this issue.
5. Impact on Data Analysis:
The choice of sample distribution significantly influences the statistical methods used for data analysis. The appropriateness of various statistical tests depends on the assumed distribution of the data. For instance, hypothesis testing relies heavily on the assumed distribution of the sample statistic to determine the probability of observing the obtained results if the null hypothesis were true. Incorrect assumptions about the sample distribution can lead to inaccurate conclusions and flawed interpretations.
Exploring the Connection Between Sampling Methods and Sample Distribution:
The sampling method employed directly impacts the resulting sample distribution. Different sampling techniques lead to different distributions and affect the ability to generalize findings to the broader population. For example:
-
Simple Random Sampling: Aims to create a sample where each member of the population has an equal chance of selection, ideally leading to a sample distribution that reflects the population distribution.
-
Stratified Sampling: Divides the population into subgroups (strata) and then samples randomly from each stratum. This ensures representation from all subgroups and can lead to a more accurate reflection of the population, even if the population distribution is not homogeneous.
-
Cluster Sampling: Samples groups (clusters) within the population. This can be more cost-effective but may lead to less accurate representations of the population.
Key Factors to Consider:
-
Roles and Real-World Examples: Consider a pharmaceutical company testing a new drug. Using simple random sampling, they select participants for the trial. The distribution of the measured variable (e.g., blood pressure) in the sample provides data on the drug's efficacy.
-
Risks and Mitigations: A potential risk is sampling bias—perhaps participants in the drug trial are not representative of the intended patient population. This can be mitigated through careful study design and rigorous recruitment strategies.
-
Impact and Implications: An accurate sample distribution allows for reliable inferences about the drug's effectiveness in the broader population. An inaccurate distribution leads to flawed conclusions and potentially unsafe or ineffective treatment.
Conclusion: Reinforcing the Connection:
The sampling method's influence on sample distribution underscores the importance of careful study design and appropriate statistical analysis. A well-designed study, utilizing a suitable sampling method, ensures a representative sample, leading to accurate inferences and reliable conclusions.
Further Analysis: Examining Sampling Bias in Greater Detail:
Sampling bias, as previously mentioned, is a significant threat to the validity of research. It arises when certain subgroups within the population are over-represented or under-represented in the sample. This can stem from various sources, including selection bias (systematic errors in selecting participants), non-response bias (individuals refusing to participate), and measurement bias (errors in data collection). Addressing sampling bias requires careful planning and execution of the sampling procedure.
Example: Sample Distribution in a Market Research Scenario
Let’s imagine a company wants to determine the proportion of consumers who prefer a new product flavor. They conduct a survey using a simple random sample of 1000 customers. The results show that 60% of the sample prefers the new flavor. The sample distribution in this case is a binomial distribution, representing the probability of observing various proportions of "prefer" and "don't prefer" responses in samples of size 1000. This binomial distribution allows the researchers to estimate the margin of error and construct a confidence interval for the true proportion of customers who prefer the new flavor within the broader population. This is crucial for making informed decisions regarding product launch and marketing strategy.
FAQ Section: Answering Common Questions About Sample Distribution
-
What is sample distribution? Sample distribution is the probability distribution of a statistic (like the mean or proportion) calculated from multiple random samples drawn from a population.
-
How is sample distribution used in hypothesis testing? Hypothesis testing relies on the assumed distribution of the sample statistic to determine the probability of observing the results if the null hypothesis is true.
-
What are the different types of sample distributions? Common types include normal, binomial, Poisson, and t-distributions, each suited to specific scenarios.
-
How can I ensure my sample is representative of the population? Use random sampling techniques, appropriately sized samples, and consider stratified or cluster sampling when appropriate.
Practical Tips: Maximizing the Benefits of Understanding Sample Distribution
-
Clearly Define the Population: Before sampling, precisely identify the target population you are trying to study.
-
Choose the Right Sampling Method: Select a sampling method appropriate for your research question and resources.
-
Ensure Adequate Sample Size: Use sample size calculators to determine an appropriate sample size to minimize sampling error.
-
Check for Sampling Bias: Carefully examine the sample to identify potential biases that might affect the results.
-
Select Appropriate Statistical Tests: Choose statistical tests suitable for your data and assumed sample distribution.
Final Conclusion: Wrapping Up with Lasting Insights
Understanding sample distribution is not merely an academic exercise; it's a critical skill for anyone working with data. From market research to healthcare, the ability to accurately interpret sample distributions is essential for drawing valid conclusions and making informed decisions. By mastering the principles of sample distribution, researchers and analysts can enhance the reliability and impact of their findings, significantly contributing to effective problem-solving and strategic planning across various fields.
Latest Posts
Latest Posts
-
Seasoned Security Definition
Apr 29, 2025
-
Seasonal Industry Definition
Apr 29, 2025
-
Seasonal Credit Definition
Apr 29, 2025
-
Seasonal Adjustment Definition Calculation Methods Example
Apr 29, 2025
-
Sealed Bid Auction Definition How It Works In Real Estate Sales
Apr 29, 2025
Related Post
Thank you for visiting our website which covers about Sample Distribution Definition How Its Used With An Example . We hope the information provided has been useful to you. Feel free to contact us if you have any questions or need further assistance. See you next time and don't miss to bookmark.