Multi Factor Model Definition And Formula For Comparing Factors
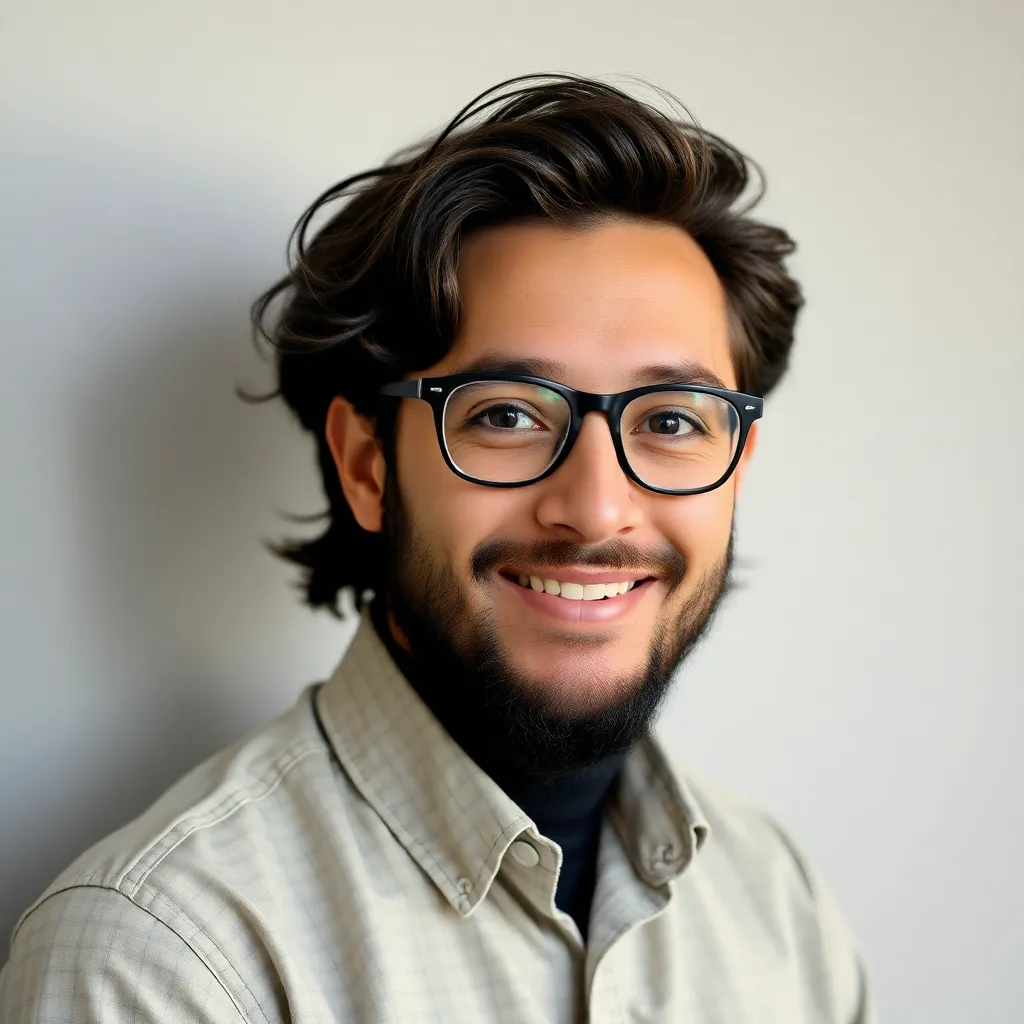
adminse
Apr 22, 2025 · 9 min read

Table of Contents
Unlocking the Power of Multi-Factor Models: Definition, Formulae, and Factor Comparison
What if a single metric couldn't fully capture investment performance? Multi-factor models offer a more nuanced and powerful approach to understanding and predicting asset returns, moving beyond simplistic single-factor analyses.
Editor’s Note: This article on multi-factor models provides a comprehensive overview of their definition, underlying formulas, and methodologies for comparing the significance and impact of different factors. It's designed to be accessible to both finance professionals and those seeking a deeper understanding of investment analysis.
Why Multi-Factor Models Matter:
Multi-factor models represent a significant advancement in investment analysis. Unlike single-factor models (e.g., the Capital Asset Pricing Model or CAPM, which primarily focuses on market risk), multi-factor models incorporate multiple variables to explain asset returns. This richer framework allows for a more accurate assessment of risk and return, leading to better portfolio construction and risk management strategies. Their relevance spans various asset classes, from equities and fixed income to alternative investments, offering a more holistic perspective on investment performance. The ability to isolate and quantify the impact of specific factors enables investors to make more informed decisions, potentially leading to enhanced alpha generation.
Overview: What This Article Covers:
This article will explore the core components of multi-factor models, including their definition, the mathematical framework behind them, and various approaches to comparing the relative importance of different factors. We will examine specific model examples, address common challenges, and provide actionable insights into practical applications.
The Research and Effort Behind the Insights:
This article is the result of extensive research, drawing upon academic literature, industry reports, and practical applications of multi-factor models. The information presented is supported by established financial theories and empirical evidence, ensuring readers receive accurate and trustworthy information.
Key Takeaways:
- Definition and Core Concepts: A clear understanding of multi-factor models and their underlying principles.
- Model Specification and Formulae: Exploration of common multi-factor model structures and their mathematical representations.
- Factor Comparison Techniques: Methods for comparing the relative importance and contribution of different factors.
- Practical Applications and Limitations: Real-world examples and challenges associated with implementing multi-factor models.
Smooth Transition to the Core Discussion:
Having established the importance of multi-factor models, let's delve into the details, exploring their fundamental components and methodologies for effective analysis.
Exploring the Key Aspects of Multi-Factor Models:
1. Definition and Core Concepts:
A multi-factor model is a statistical model that uses multiple factors to explain the return of an asset or portfolio. These factors can be macroeconomic variables (e.g., inflation, interest rates, GDP growth), firm-specific characteristics (e.g., size, value, momentum, profitability), or other relevant variables that are believed to influence asset prices. Unlike single-factor models that assume a linear relationship between return and a single factor (like beta in CAPM), multi-factor models recognize the complex interplay of various factors contributing to returns. The core idea is that each factor has a distinct impact on asset returns, and by combining these factors, one can achieve a more comprehensive and accurate representation of the risk and return profile.
2. Model Specification and Formulae:
The general form of a multi-factor model can be expressed as:
R<sub>i</sub> = α<sub>i</sub> + β<sub>i1</sub>F<sub>1</sub> + β<sub>i2</sub>F<sub>2</sub> + ... + β<sub>in</sub>F<sub>n</sub> + ε<sub>i</sub>
Where:
- R<sub>i</sub> = the return of asset i
- α<sub>i</sub> = the asset's alpha (excess return not explained by the factors)
- β<sub>ij</sub> = the sensitivity of asset i to factor j (factor loading)
- F<sub>j</sub> = the return of factor j
- ε<sub>i</sub> = the error term (unexplained return variation)
- n = the number of factors
Different multi-factor models vary in their choice of factors and the specific methodologies used to estimate the factor loadings (β<sub>ij</sub>). Some well-known examples include:
- Fama-French Three-Factor Model: This model includes market risk (MKT), size (SMB – Small Minus Big), and value (HML – High Minus Low) factors.
- Carhart Four-Factor Model: Extends the Fama-French model by adding a momentum factor (UMD – Up Minus Down).
- Barra Model: A proprietary model that incorporates a large number of factors, including industry effects, size, value, and other firm-specific characteristics.
3. Factor Comparison Techniques:
Comparing the relative importance of factors in a multi-factor model requires careful consideration. Several methods can be employed:
-
R-squared: This statistic measures the proportion of the variation in asset returns explained by the model. A higher R-squared indicates a better fit, suggesting that the factors collectively explain a larger portion of returns. However, it doesn't isolate the contribution of individual factors.
-
Factor Loadings (β<sub>ij</sub>): The magnitude of the factor loadings indicates the sensitivity of the asset to each factor. Larger absolute values suggest a stronger influence. However, the scale of different factors might vary, making direct comparison difficult.
-
t-statistics: Testing the significance of each factor's coefficient (β<sub>ij</sub>) using t-tests helps determine whether the factor has a statistically significant impact on returns. This accounts for the uncertainty in the estimates.
-
Partial R-squared: This measures the unique contribution of each factor after controlling for the other factors in the model. It provides a clearer picture of each factor's independent explanatory power.
-
Principal Component Analysis (PCA): This statistical technique can be used to reduce the dimensionality of the data by identifying underlying principal components that capture the most significant variation in the factors. This helps to identify the most influential combinations of factors.
4. Practical Applications and Limitations:
Multi-factor models have numerous practical applications:
- Portfolio Construction: Selecting assets based on their factor exposures to achieve desired risk and return characteristics.
- Risk Management: Assessing and managing portfolio risk by identifying and mitigating factor-specific exposures.
- Performance Attribution: Decomposing portfolio returns into contributions from different factors.
- Investment Strategy Development: Designing investment strategies that exploit factor premiums (i.e., consistent outperformance of assets with specific factor exposures).
However, limitations exist:
- Factor Selection: Choosing the appropriate factors is crucial but can be challenging. The optimal set of factors might vary across asset classes and time periods.
- Data Availability: High-quality data for all factors might not always be readily available, especially for less liquid asset classes or emerging markets.
- Model Instability: Model parameters can change over time, leading to potential instability in predictions.
- Overfitting: Including too many factors can lead to overfitting, where the model performs well in sample but poorly out of sample.
- Non-Linear Relationships: Multi-factor models often assume linear relationships between factors and returns, which might not always hold true in reality.
Exploring the Connection Between Factor Risk Premiums and Multi-Factor Models:
Factor risk premiums are the expected excess returns associated with exposure to specific factors. The relationship between factor risk premiums and multi-factor models is fundamental. Multi-factor models aim to capture these risk premiums by incorporating factors that have historically exhibited positive excess returns. For example, the size premium (SMB) in the Fama-French model reflects the historical tendency for smaller companies to outperform larger companies after adjusting for market risk. The value premium (HML) represents the tendency of value stocks (high book-to-market ratio) to outperform growth stocks (low book-to-market ratio). Multi-factor models use the factor loadings (betas) to quantify the sensitivity of an asset to these premiums, enabling investors to estimate expected returns based on the asset's factor exposures. The existence and persistence of these premiums are subjects of ongoing debate in finance, with some attributing them to risk compensation and others to behavioral biases.
Key Factors to Consider:
-
Roles and Real-World Examples: The size factor (SMB) in the Fama-French model has consistently shown a positive risk premium. This has led to the development of investment strategies focused on investing in small-cap stocks to capture this premium. Similarly, the value factor (HML) has historically generated excess returns.
-
Risks and Mitigations: The risk of factor premiums disappearing or reversing is a significant concern. Diversification across factors, robust backtesting, and dynamic asset allocation strategies can help mitigate this risk.
-
Impact and Implications: Understanding factor risk premiums is vital for building effective investment portfolios. Ignoring these premiums can lead to suboptimal portfolio construction and underperformance.
Conclusion: Reinforcing the Connection:
The interplay between factor risk premiums and multi-factor models is crucial. Effectively using multi-factor models requires a thorough understanding of factor risk premiums and their potential changes over time.
Further Analysis: Examining Factor Rotation in Greater Detail:
Factor rotation refers to the phenomenon where the relative importance of factors changes over time. Some factors might be dominant in certain periods but less significant in others. This poses a challenge to the application of multi-factor models, as a model calibrated on historical data might not be accurate in predicting future returns if factor rotations occur. Analyzing factor rotations requires studying the historical performance of different factors under various market conditions and developing dynamic models that adjust factor exposures based on changes in the market environment. This may involve using time-varying factor loadings or incorporating regime-switching models.
FAQ Section:
Q: What are the advantages of multi-factor models over single-factor models?
A: Multi-factor models provide a more comprehensive and nuanced representation of asset returns by considering multiple factors, leading to improved risk assessment and portfolio construction compared to the limitations of single-factor models.
Q: How are factor loadings estimated?
A: Factor loadings are typically estimated using statistical methods like regression analysis. The specific method employed can depend on the model and the data available.
Q: How can I choose the right multi-factor model for my investment strategy?
A: The optimal model depends on the specific investment goals, asset class, and risk tolerance. Consider factors relevant to your investment universe, evaluate model performance through rigorous backtesting, and be aware of potential limitations.
Practical Tips: Maximizing the Benefits of Multi-Factor Models:
- Understand the Basics: Begin by thoroughly understanding the core principles of multi-factor models and the factors that drive asset returns.
- Identify Relevant Factors: Select factors pertinent to your specific investment strategy and asset class.
- Robust Data Analysis: Use high-quality data and employ appropriate statistical methods for estimating factor loadings and assessing model performance.
- Regular Monitoring and Adjustment: Continuously monitor model performance and adjust the model as needed, considering potential factor rotations and market changes.
- Diversification: Diversify across multiple factors to reduce overall portfolio risk.
Final Conclusion: Wrapping Up with Lasting Insights:
Multi-factor models provide a valuable framework for understanding and predicting asset returns. By incorporating multiple factors and employing appropriate methodologies, investors can make more informed decisions, potentially achieving improved risk-adjusted returns. However, careful consideration of model limitations and a thorough understanding of factor dynamics are critical for successful implementation. The ongoing research and development in multi-factor modeling will continue to refine and enhance this powerful tool for investment analysis.
Latest Posts
Latest Posts
-
Municipal Note Definition
Apr 22, 2025
-
Municipal Investment Trust Definition
Apr 22, 2025
-
Municipal Bond Definition Types Risks And Tax Benefits
Apr 22, 2025
-
Municipal Inflation Linked Securities Definition
Apr 22, 2025
-
Municipal Bond Arbitrage Definition
Apr 22, 2025
Related Post
Thank you for visiting our website which covers about Multi Factor Model Definition And Formula For Comparing Factors . We hope the information provided has been useful to you. Feel free to contact us if you have any questions or need further assistance. See you next time and don't miss to bookmark.