Cut Off Score Definition And What It Means To Consumers
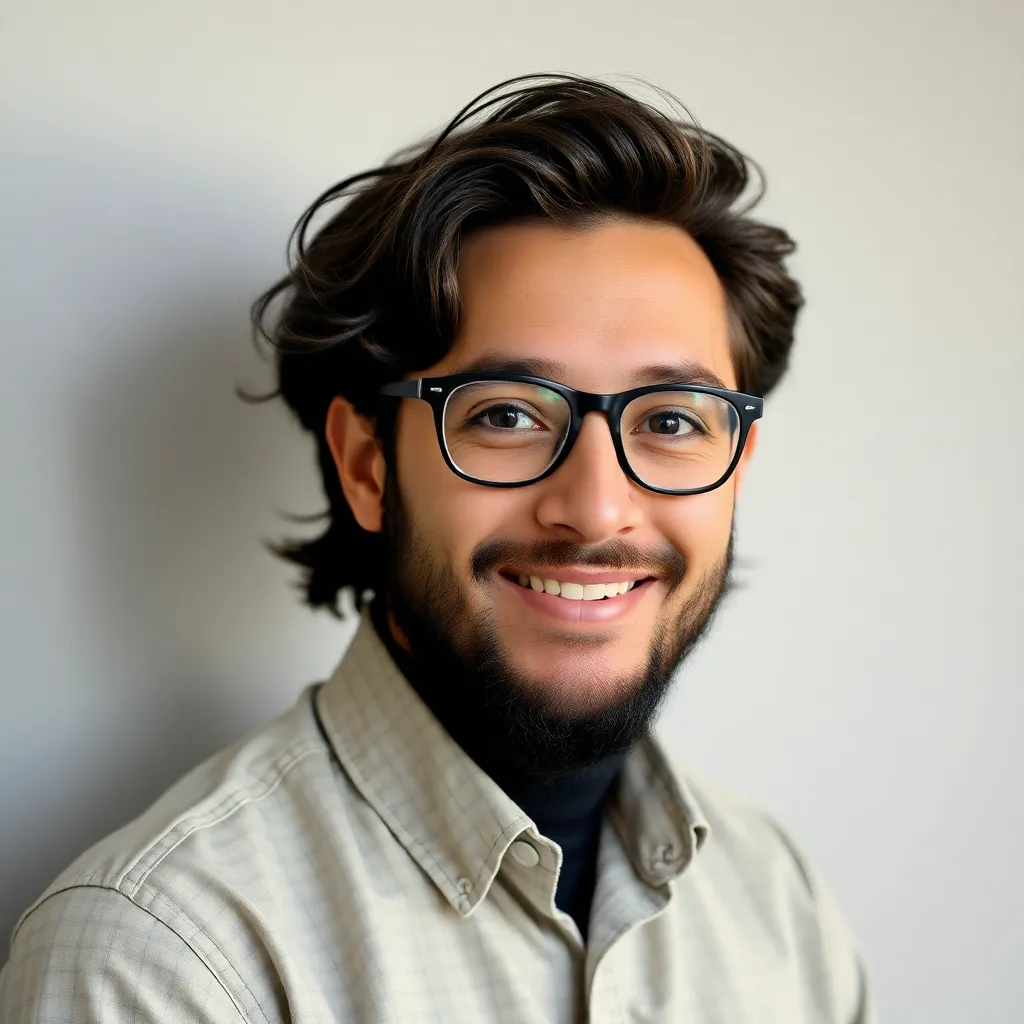
adminse
Mar 25, 2025 · 8 min read
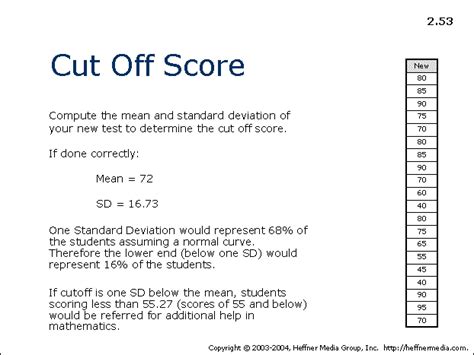
Table of Contents
Decoding the Cut-Off Score: What It Means to Consumers
What if your access to essential services, financial products, or even opportunities hinged on a single number? This seemingly simple metric, the cut-off score, wields significant power in shaping consumer experiences and access to various resources.
Editor’s Note: This article on cut-off scores provides a comprehensive overview of their definition, implications for consumers, and the ethical considerations surrounding their use. It's designed to empower consumers with the knowledge to navigate situations where cut-off scores are applied.
Why Cut-Off Scores Matter: Relevance, Practical Applications, and Industry Significance
Cut-off scores are numerical thresholds used across numerous sectors to filter or categorize individuals based on predefined criteria. These scores determine eligibility for loans, insurance policies, credit cards, employment opportunities, and even access to social services in some instances. Their seemingly objective nature often masks the complex calculations and potential biases embedded within their methodology. Understanding cut-off scores is crucial for consumers because they directly impact financial access, social mobility, and overall well-being. The implications extend beyond individual experiences, influencing market competition, financial inclusion, and societal equity.
Overview: What This Article Covers
This article provides a detailed examination of cut-off scores, exploring their definition, diverse applications, ethical concerns, and the impact on consumers. We will delve into the methodologies used, the potential for bias, and strategies consumers can employ to understand and navigate situations involving cut-off scores. The article also explores specific examples across various sectors, offering practical insights and recommendations.
The Research and Effort Behind the Insights
This article draws upon extensive research encompassing academic literature, industry reports, government data, and analysis of consumer experiences. Information is sourced from reputable organizations, government agencies, and financial institutions. The analysis strives for neutrality and objectivity, aiming to present a balanced perspective on the complexities surrounding cut-off scores.
Key Takeaways:
- Definition and Core Concepts: A clear explanation of cut-off scores and their foundational principles.
- Applications Across Industries: Examples of how cut-off scores are used across various sectors, including finance, insurance, and employment.
- Challenges and Solutions: Identification of potential biases and strategies to mitigate their impact on consumers.
- Ethical Considerations: An exploration of the ethical dilemmas associated with the use of cut-off scores and their implications for fairness and equality.
- Consumer Empowerment: Practical tips and strategies for consumers to understand and navigate situations involving cut-off scores.
Smooth Transition to the Core Discussion
Having established the importance of cut-off scores, let's delve into their specific definition and the various contexts in which they are applied.
Exploring the Key Aspects of Cut-Off Scores
Definition and Core Concepts:
A cut-off score is a predetermined numerical value that serves as a threshold for selection or classification. It represents the minimum score required to meet a specific criterion. These scores are often derived from complex algorithms, scoring models, or statistical analyses applied to various data points. The specific data points and algorithms vary greatly depending on the context. For instance, a credit score cut-off for a mortgage might be 680, while a cut-off for a particular job applicant screening process could be based on a composite score of skills tests, experience, and educational qualifications.
Applications Across Industries:
-
Finance: Cut-off scores are extensively used in the financial sector. Credit scores, for example, are fundamental in determining eligibility for loans, credit cards, and mortgages. Insurance companies also utilize scoring models to assess risk and set premiums. These scores consider factors like credit history, income, debt-to-income ratio, and other relevant financial data. A low cut-off score can result in denial of credit or higher interest rates, significantly impacting financial access.
-
Insurance: Insurance companies use cut-off scores to assess risk and determine premiums. Factors such as driving history, health status, and claims history contribute to these scores. Individuals with scores below the cut-off might face higher premiums or denial of coverage. This can create disparities in access to essential insurance products based on factors that may not fully reflect individual risk.
-
Employment: Some employers use cut-off scores in their hiring processes. These scores can be based on standardized tests, background checks, or assessments of skills and experience. While potentially streamlining the hiring process, such scores can inadvertently perpetuate biases, leading to exclusion of qualified candidates from diverse backgrounds.
-
Social Services: In certain instances, eligibility for social services might be determined by a cut-off score based on factors like income, household size, and disability status. These scores, intended to allocate resources effectively, can inadvertently create barriers for those who fall just below the threshold, exacerbating existing inequalities.
Challenges and Solutions:
One significant challenge lies in the potential for bias within the scoring models themselves. Algorithms trained on historical data might inadvertently perpetuate existing societal inequalities. For example, if historical lending data reflects discriminatory practices, a credit scoring model trained on that data could disproportionately disadvantage certain demographics.
Another challenge is the lack of transparency in many scoring systems. Consumers often lack clear understanding of how their scores are calculated, making it difficult to challenge inaccuracies or biases.
To address these challenges, increased transparency in scoring methodologies is crucial. Regular audits of scoring systems to identify and mitigate biases are necessary. Furthermore, providing consumers with clear explanations of their scores and the factors influencing them can enhance fairness and accountability.
Impact on Innovation:
Ironically, the drive for efficiency and innovation in data analytics can sometimes inadvertently lead to inequitable outcomes. The increasing reliance on complex algorithms and automated decision-making systems necessitates careful consideration of potential biases and their impact on vulnerable populations. Developing scoring systems that are both efficient and equitable requires ongoing refinement and ethical oversight.
Closing Insights: Summarizing the Core Discussion
Cut-off scores represent a double-edged sword. While they can streamline processes and improve efficiency, their use necessitates a constant vigilance against bias and a commitment to transparency and fairness. The potential for exclusion and inequitable outcomes underscores the need for careful consideration and ongoing refinement of these scoring systems.
Exploring the Connection Between Algorithmic Bias and Cut-Off Scores
Algorithmic bias plays a crucial role in shaping the outcomes of cut-off score systems. Bias can creep into the system at various stages: from the data used to train the algorithms to the design of the algorithms themselves. The consequences can be far-reaching, affecting access to credit, insurance, employment, and essential services.
Key Factors to Consider:
-
Roles and Real-World Examples: Algorithmic bias manifests in various ways. For instance, if a loan application scoring system is trained on data reflecting historical discrimination against a particular demographic, the algorithm might unfairly deny loans to applicants from that group, even if their creditworthiness is comparable to others.
-
Risks and Mitigations: The risks associated with algorithmic bias include perpetuating and amplifying existing inequalities. Mitigation strategies include rigorous testing of algorithms for bias, using diverse and representative datasets, and incorporating human oversight in the decision-making process.
-
Impact and Implications: The long-term impact of biased cut-off scores can be significant, leading to limited economic opportunities, reduced social mobility, and increased inequality.
Conclusion: Reinforcing the Connection
The connection between algorithmic bias and cut-off scores underscores the need for careful scrutiny and ethical considerations in the design and implementation of scoring systems. Addressing algorithmic bias requires a multi-pronged approach, encompassing data quality, algorithm design, and ongoing monitoring and evaluation.
Further Analysis: Examining Algorithmic Bias in Greater Detail
Algorithmic bias is a complex phenomenon stemming from various sources. One key aspect is the inherent biases present in the data used to train the algorithms. Historical data often reflects existing societal biases, which can be inadvertently replicated and amplified by the algorithms. Another source of bias lies in the design of the algorithms themselves. Subtle biases in the algorithm's logic can lead to discriminatory outcomes.
FAQ Section: Answering Common Questions About Cut-Off Scores
-
What is a cut-off score? A cut-off score is a predetermined numerical threshold used to categorize or select individuals based on a set of criteria.
-
How are cut-off scores determined? The methods vary depending on the context, often involving statistical analysis, scoring models, and complex algorithms.
-
Are cut-off scores always fair and unbiased? No, cut-off scores can perpetuate biases present in the underlying data or the algorithms used to calculate them.
-
What can consumers do if they believe their cut-off score is unfair? Consumers can investigate the basis of their score, seek explanations from the relevant organization, and explore available recourse mechanisms.
Practical Tips: Maximizing the Benefits of Understanding Cut-Off Scores
-
Understand the Basics: Learn about the different types of cut-off scores used in various sectors.
-
Request Explanations: If you are denied a service or opportunity based on a cut-off score, request a detailed explanation of the factors contributing to your score.
-
Monitor Your Scores: Regularly monitor your credit score, insurance score, or other relevant scores to identify potential inaccuracies or areas for improvement.
-
Advocate for Fairness: Support initiatives promoting transparency and accountability in the use of cut-off scores.
Final Conclusion: Wrapping Up with Lasting Insights
Cut-off scores are integral to many decision-making processes, but their impact on consumers necessitates ongoing scrutiny and ethical considerations. By understanding the complexities of cut-off scores, consumers can become more informed participants in systems that significantly affect their lives. Advocating for transparency, fairness, and mitigation of biases is crucial to ensuring that these systems promote equity and opportunity for all.
Latest Posts
Latest Posts
-
Chemo Xrt Definition
Apr 02, 2025
-
Palliative Xrt Definition
Apr 02, 2025
-
Xrt Definition Medical Terms
Apr 02, 2025
-
Xenocurrency Meaning
Apr 02, 2025
-
Xenocurrency Adalah
Apr 02, 2025
Related Post
Thank you for visiting our website which covers about Cut Off Score Definition And What It Means To Consumers . We hope the information provided has been useful to you. Feel free to contact us if you have any questions or need further assistance. See you next time and don't miss to bookmark.