Wilcoxon Test Definition In Statistics Types And Calculation
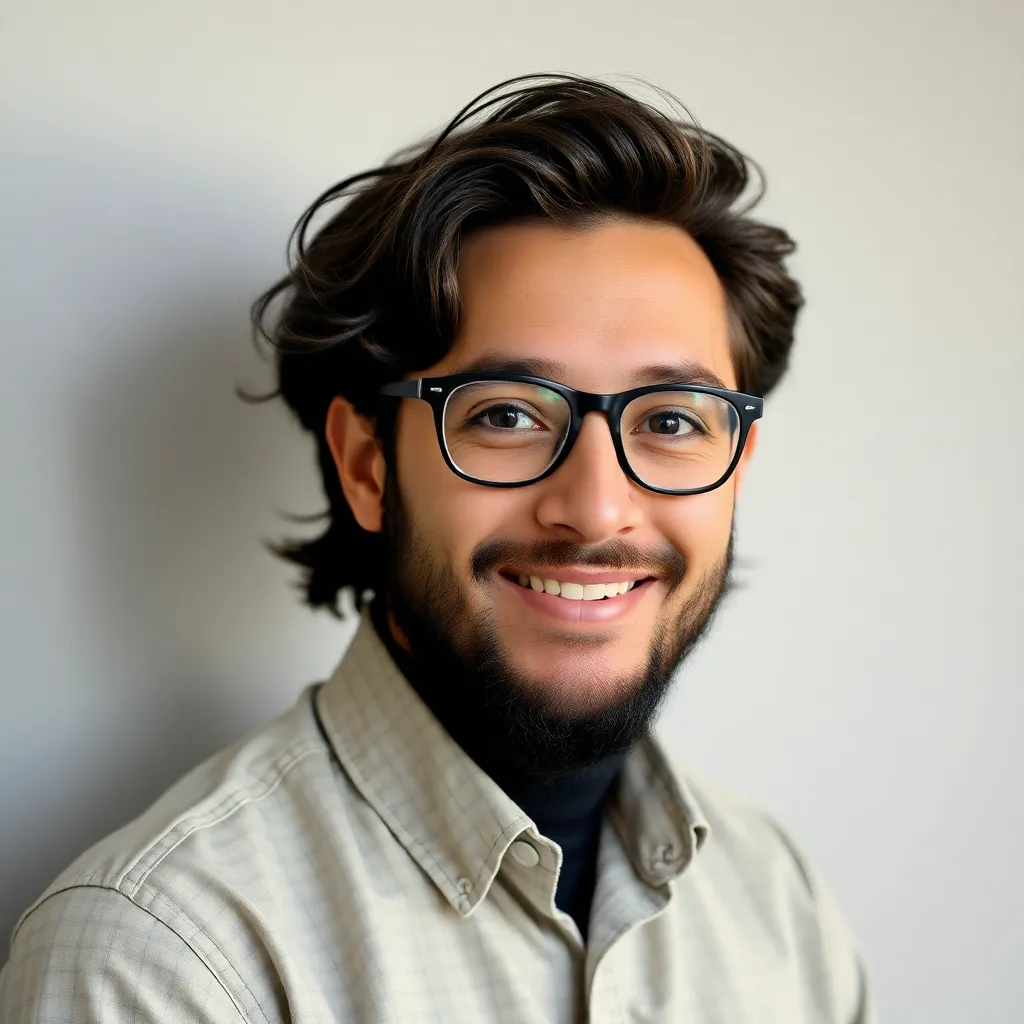
adminse
Mar 21, 2025 · 10 min read

Table of Contents
Unlocking the Power of the Wilcoxon Test: Definitions, Types, and Calculations
What if a powerful non-parametric test could unlock insights from data that traditional methods miss? The Wilcoxon test, a robust statistical tool, offers precisely that capability, providing valuable insights even when assumptions of normality are violated.
Editor’s Note: This article on the Wilcoxon test provides a comprehensive overview of its definition, types, and calculation methods. Updated with the latest statistical best practices, this guide equips readers with the knowledge to confidently apply this valuable statistical technique.
Why the Wilcoxon Test Matters:
The Wilcoxon test stands as a cornerstone of non-parametric statistics. Unlike parametric tests (like the t-test), it doesn't assume that the data is normally distributed. This makes it incredibly versatile and applicable in situations where parametric assumptions are untenable. Its relevance spans numerous fields, including medicine, psychology, social sciences, and engineering, wherever researchers grapple with data that may not meet the stringent requirements of parametric tests. The ability to analyze ordinal data, ranked data, and data with outliers effectively broadens its practical applications significantly.
Overview: What This Article Covers:
This article will thoroughly explore the Wilcoxon test, covering its fundamental definitions, its two main types (Wilcoxon signed-rank test and Wilcoxon rank-sum test), step-by-step calculation procedures, and interpretations of the results. Readers will gain a practical understanding of when to use each type and how to interpret the resulting p-values to draw meaningful conclusions. Furthermore, the article will highlight the strengths and limitations of the Wilcoxon test and offer guidance on appropriate software usage.
The Research and Effort Behind the Insights:
This article draws upon established statistical literature, including seminal works on non-parametric statistics and numerous peer-reviewed publications showcasing applications of the Wilcoxon test across various disciplines. The calculation procedures outlined are based on standard statistical methods, and examples are provided for clarity. All information is presented in a clear, accessible manner, minimizing technical jargon to ensure readability for a broad audience.
Key Takeaways:
- Definition and Core Concepts: A precise definition of the Wilcoxon test and its underlying principles.
- Wilcoxon Signed-Rank Test: Detailed explanation, calculation steps, and interpretation for paired samples.
- Wilcoxon Rank-Sum Test (Mann-Whitney U Test): Detailed explanation, calculation steps, and interpretation for independent samples.
- Interpreting Results: Understanding p-values and making informed statistical decisions.
- Software Applications: Guidance on utilizing statistical software for Wilcoxon test calculations.
Smooth Transition to the Core Discussion:
Having established the importance and scope of the Wilcoxon test, let's delve into its core aspects, beginning with a clear definition and differentiating its two primary forms.
Exploring the Key Aspects of the Wilcoxon Test:
1. Definition and Core Concepts:
The Wilcoxon test is a non-parametric statistical test used to compare two groups of data. Unlike parametric tests that rely on assumptions about the data's distribution (e.g., normality), the Wilcoxon test works with ranked data. This means it considers the relative order of the data points rather than their exact numerical values. This makes it robust to outliers and suitable for data that violates the assumptions of normality. The test assesses whether there's a statistically significant difference in the ranks between the two groups.
2. Wilcoxon Signed-Rank Test (for Paired Samples):
The Wilcoxon signed-rank test is designed for paired data, such as before-and-after measurements on the same subjects or matched pairs of subjects. It assesses whether the median difference between the paired observations is significantly different from zero. The steps for conducting the Wilcoxon signed-rank test are as follows:
- Step 1: Calculate the differences: Subtract one measurement from the other for each pair.
- Step 2: Rank the absolute differences: Rank the absolute values of the differences from smallest to largest, ignoring the signs. Assign average ranks in case of ties.
- Step 3: Sum the ranks of positive differences (W+): Add the ranks corresponding to the positive differences.
- Step 4: Sum the ranks of negative differences (W-): Add the ranks corresponding to the negative differences.
- Step 5: Determine the test statistic: The test statistic (W) is the smaller of W+ and W-.
- Step 6: Determine the critical value: Consult a Wilcoxon signed-rank test table based on the sample size (n) and the chosen significance level (alpha, typically 0.05).
- Step 7: Compare the test statistic to the critical value: If the test statistic (W) is less than or equal to the critical value, reject the null hypothesis (that there is no difference between the paired observations).
Example:
Consider a study investigating the effectiveness of a new drug to lower blood pressure. Blood pressure is measured before and after administering the drug to 10 patients. The differences (after - before) are calculated, ranked, and the sums of positive and negative ranks are determined. Based on the smaller of W+ and W-, a decision is made regarding the null hypothesis.
3. Wilcoxon Rank-Sum Test (Mann-Whitney U Test) (for Independent Samples):
The Wilcoxon rank-sum test, also known as the Mann-Whitney U test, is used to compare two independent groups. It determines whether the distributions of the two groups differ significantly. The steps are:
-
Step 1: Combine and Rank Data: Combine the data from both groups and rank all observations from smallest to largest. Assign average ranks for ties.
-
Step 2: Calculate the sum of ranks for each group (R1 and R2): Sum the ranks for each of the two groups separately.
-
Step 3: Calculate the Mann-Whitney U statistic:
U1 = n1n2 + (n1(n1+1))/2 - R1 U2 = n1n2 + (n2(n2+1))/2 - R2
Where n1 and n2 are the sample sizes of group 1 and group 2 respectively, and R1 and R2 are the sum of ranks for group 1 and group 2. The U statistic is the smaller of U1 and U2.
-
Step 4: Determine the critical value: Consult a Wilcoxon rank-sum test table using the sample sizes (n1 and n2) and the significance level (alpha).
-
Step 5: Compare the U statistic to the critical value: If the calculated U statistic is less than or equal to the critical value, reject the null hypothesis (that there's no difference between the groups).
Example:
Suppose researchers want to compare the effectiveness of two different teaching methods. Two independent groups of students are taught using each method, and their final exam scores are compared using the Wilcoxon rank-sum test.
4. Interpreting Results:
The p-value obtained from the Wilcoxon test indicates the probability of observing the obtained results (or more extreme results) if there were no real difference between the groups (null hypothesis). A p-value less than the chosen significance level (usually 0.05) leads to the rejection of the null hypothesis, suggesting a statistically significant difference between the groups.
5. Software Applications:
Statistical software packages like R, SPSS, SAS, and Python (with libraries like SciPy) readily perform Wilcoxon tests. These packages automate the calculations and provide p-values, making the analysis efficient and accurate.
Exploring the Connection Between Effect Size and the Wilcoxon Test:
While the p-value indicates statistical significance, it doesn't reveal the magnitude of the difference between the groups. Effect size measures quantify this magnitude. For the Wilcoxon tests, several effect size measures exist, including:
- r (correlation coefficient): A common measure that ranges from -1 to +1. A larger absolute value indicates a stronger effect.
- Cliff's delta: Another popular effect size measure that represents the probability that a randomly selected observation from one group will be greater than a randomly selected observation from the other group.
Understanding effect sizes is crucial for interpreting the practical significance of the Wilcoxon test results.
Key Factors to Consider:
- Tied Ranks: The presence of tied ranks in the data requires adjustments to the calculation formulas. Most statistical software handles tied ranks automatically.
- Large Sample Sizes: For large samples, the Wilcoxon test statistic can be approximated using a normal distribution, simplifying calculations.
- Assumptions: While the Wilcoxon test doesn't assume normality, it still assumes that the data are at least ordinal (meaning they can be ranked).
Risks and Mitigations:
- Misinterpretation of p-values: It's crucial to understand that a statistically significant result doesn't automatically imply practical significance. Consider the effect size along with the p-value.
- Inappropriate use: The Wilcoxon test should only be applied when the assumptions of the test are met. Applying it to inappropriate data can lead to misleading conclusions.
Impact and Implications:
The Wilcoxon test plays a vital role in ensuring the robustness of statistical analysis, particularly when dealing with non-normal data. Its widespread application across various disciplines reflects its value in providing reliable insights from diverse datasets.
Conclusion: Reinforcing the Connection:
The Wilcoxon test provides a powerful and flexible non-parametric alternative to parametric tests. Understanding its two forms (signed-rank and rank-sum) and the steps involved in their calculation, along with proper interpretation of results, enables researchers to draw accurate and meaningful conclusions from their data, even when normality assumptions are violated.
Further Analysis: Examining Effect Size Measures in Greater Detail:
Various effect size measures complement the p-value from Wilcoxon tests. Researchers should choose the most appropriate measure based on the specific research question and data characteristics. Each measure offers a unique perspective on the magnitude of the effect, enhancing the interpretability of the findings.
FAQ Section: Answering Common Questions About the Wilcoxon Test:
-
Q: What is the difference between the Wilcoxon signed-rank test and the Wilcoxon rank-sum test?
- A: The signed-rank test is for paired samples, while the rank-sum test (Mann-Whitney U) is for independent samples.
-
Q: Can the Wilcoxon test be used with interval or ratio data?
- A: Yes, but it utilizes the ranks of the data, not the actual values. This makes it robust to outliers and non-normality.
-
Q: What happens if I have many ties in my data?
- A: Most statistical software accounts for ties in the calculations. However, a very large number of ties might slightly affect the accuracy of the results.
-
Q: How do I choose between a Wilcoxon test and a t-test?
- A: If your data is normally distributed, a t-test is generally preferred. However, if normality is violated, or if your data is ordinal, the Wilcoxon test is more appropriate.
Practical Tips: Maximizing the Benefits of the Wilcoxon Test:
-
Check data assumptions: Before running the test, ensure your data meets the basic assumptions of ordinality and independence (for the rank-sum test).
-
Choose the correct test: Select the signed-rank test for paired samples and the rank-sum test for independent samples.
-
Report effect size: Don't rely solely on the p-value. Report an appropriate effect size to convey the magnitude of the difference.
-
Use statistical software: Utilize statistical software to avoid manual calculation errors and obtain accurate p-values and effect sizes.
Final Conclusion: Wrapping Up with Lasting Insights:
The Wilcoxon test remains an invaluable tool in the statistician's arsenal. Its ability to handle non-normal data and provide robust results makes it essential for researchers across diverse fields. By understanding its nuances, limitations, and appropriate application, researchers can leverage its power to uncover valuable insights hidden within their datasets. Proper interpretation, encompassing both statistical significance and effect size, is key to drawing meaningful conclusions and contributing to a deeper understanding of the phenomena under investigation.
Latest Posts
Related Post
Thank you for visiting our website which covers about Wilcoxon Test Definition In Statistics Types And Calculation . We hope the information provided has been useful to you. Feel free to contact us if you have any questions or need further assistance. See you next time and don't miss to bookmark.