What Is Supply Chain Analytics
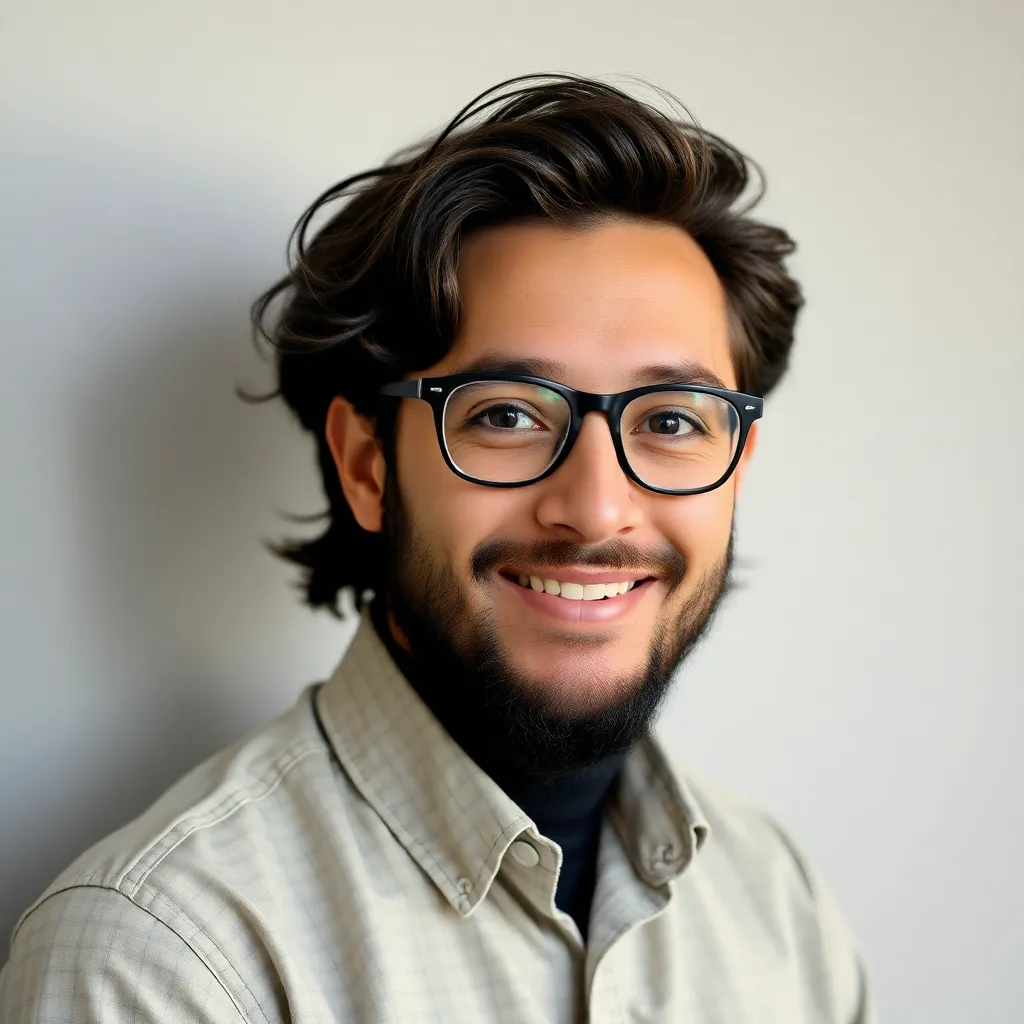
adminse
Mar 22, 2025 · 10 min read

Table of Contents
Unlocking the Power of Prediction: A Deep Dive into Supply Chain Analytics
What if businesses could anticipate disruptions before they strike, optimizing their operations for unparalleled efficiency and profitability? Supply chain analytics is the key, transforming reactive strategies into proactive, data-driven decision-making.
Editor’s Note: This article on supply chain analytics was published today, providing readers with the latest insights and best practices in this rapidly evolving field. The information presented here is based on extensive research, combining academic studies, industry reports, and practical case studies to offer a comprehensive understanding of the subject.
Why Supply Chain Analytics Matters:
In today's interconnected global economy, supply chains are the lifeblood of businesses. They encompass the intricate network of activities involved in sourcing, producing, and delivering goods and services to end consumers. However, these networks are complex, vulnerable to a multitude of disruptions, and often operate with limited visibility into key performance indicators (KPIs). This is where supply chain analytics steps in, offering a transformative approach to optimizing these complex systems. Its relevance extends far beyond simple efficiency gains; it's about building resilience, enhancing agility, and ultimately, driving sustainable competitive advantage. From predicting demand fluctuations to mitigating risk and improving customer satisfaction, supply chain analytics offers actionable insights that directly impact the bottom line. Understanding and implementing these techniques is no longer a luxury but a necessity for organizations aiming to thrive in the modern business landscape.
Overview: What This Article Covers:
This article provides a comprehensive exploration of supply chain analytics, covering its core concepts, practical applications, and the challenges involved in its implementation. Readers will gain a clear understanding of the various analytical techniques employed, the types of data utilized, and the potential impact on various industry sectors. We will delve into specific examples and case studies, highlighting best practices and demonstrating the tangible benefits of embracing this powerful approach.
The Research and Effort Behind the Insights:
This article is the result of extensive research, drawing upon a wide range of sources, including peer-reviewed academic journals, industry reports from reputable firms like Gartner and McKinsey, and case studies from leading companies that have successfully implemented supply chain analytics. The information presented is carefully vetted to ensure accuracy and objectivity, providing readers with reliable and actionable insights.
Key Takeaways:
- Definition and Core Concepts: A clear explanation of supply chain analytics, its fundamental principles, and its relationship to other analytical disciplines.
- Data Sources and Types: An overview of the various data sources utilized in supply chain analytics, including transactional data, sensor data, and external market data.
- Analytical Techniques: An exploration of the key analytical methods, including descriptive, predictive, and prescriptive analytics.
- Applications Across Industries: Real-world examples of how supply chain analytics is transforming operations across various sectors, including retail, manufacturing, and logistics.
- Challenges and Solutions: Identification of common challenges and the strategies to overcome them, emphasizing data quality, integration, and talent acquisition.
- Future Implications: Discussion of the emerging trends and the potential impact of advanced analytics techniques, such as artificial intelligence and machine learning.
Smooth Transition to the Core Discussion:
Having established the importance and scope of supply chain analytics, let's delve into the specifics of this powerful tool, examining its core components and its transformative impact on modern business operations.
Exploring the Key Aspects of Supply Chain Analytics:
1. Definition and Core Concepts:
Supply chain analytics is the application of advanced analytical techniques to supply chain data to gain insights that improve decision-making and optimize performance. It goes beyond basic reporting; it leverages data-driven insights to predict future trends, identify potential problems, and proactively implement solutions to enhance efficiency, reduce costs, and increase profitability. The core objective is to move from a reactive, fire-fighting approach to a proactive, data-informed strategy. This involves utilizing various analytical techniques to understand past performance, predict future outcomes, and prescribe optimal actions.
2. Data Sources and Types:
Effective supply chain analytics relies on access to a wide variety of data sources. These can be broadly categorized as:
- Internal Data: This includes transactional data from Enterprise Resource Planning (ERP) systems, Customer Relationship Management (CRM) systems, and other internal databases. Examples include sales orders, inventory levels, production schedules, transportation data, and supplier performance metrics.
- External Data: This encompasses data from external sources, such as market research reports, economic indicators, weather data, geopolitical events, and social media sentiment analysis. This data provides context and allows for a more holistic view of the supply chain.
- Sensor Data: The rise of the Internet of Things (IoT) has led to an explosion of sensor data from various points within the supply chain. This includes data from GPS trackers on vehicles, sensors monitoring temperature and humidity in warehouses, and machine sensors tracking equipment performance.
The types of data used include:
- Structured Data: This refers to data organized in a predefined format, such as databases and spreadsheets.
- Semi-structured Data: This includes data that doesn't conform to a rigid structure but contains some organization, such as XML or JSON files.
- Unstructured Data: This is data without a predefined format, such as text documents, emails, and images. Advanced techniques are required to extract meaningful insights from unstructured data.
3. Analytical Techniques:
Supply chain analytics employs a range of analytical techniques, including:
- Descriptive Analytics: This involves analyzing historical data to understand past performance and identify trends. Examples include calculating key performance indicators (KPIs) like inventory turnover, on-time delivery rate, and order fulfillment cycle time.
- Predictive Analytics: This uses historical data and statistical modeling to forecast future outcomes, such as demand forecasting, supply disruptions, and potential bottlenecks. Techniques like time series analysis, regression analysis, and machine learning algorithms are commonly employed.
- Prescriptive Analytics: This goes beyond prediction and involves recommending optimal actions to improve outcomes. This often involves optimization models and simulation techniques to determine the best course of action in various scenarios.
4. Applications Across Industries:
Supply chain analytics has widespread applications across various industries:
- Retail: Optimizing inventory levels, predicting demand fluctuations, personalizing customer experiences, and improving supply chain resilience.
- Manufacturing: Optimizing production schedules, improving resource allocation, reducing waste, and enhancing quality control.
- Logistics: Optimizing transportation routes, improving delivery times, managing warehousing effectively, and reducing transportation costs.
- Healthcare: Managing pharmaceutical supply chains, optimizing inventory levels of medical supplies, improving patient care, and ensuring timely delivery of essential medications.
5. Challenges and Solutions:
Implementing effective supply chain analytics faces several challenges:
- Data Quality: Inaccurate or incomplete data can lead to flawed insights. Data cleansing and validation are crucial.
- Data Integration: Integrating data from various sources can be complex and time-consuming. Robust data integration platforms are essential.
- Talent Acquisition: Finding and retaining skilled professionals with the necessary analytical expertise is a significant challenge. Investing in training and development is key.
- Technology Investment: Implementing advanced analytical tools and technologies requires significant investment. A phased approach can be effective.
Solutions include investing in robust data management systems, employing data integration tools, developing internal expertise through training and recruitment, and adopting a phased approach to technology implementation.
6. Future Implications:
The future of supply chain analytics is closely tied to advancements in technology:
- Artificial Intelligence (AI) and Machine Learning (ML): AI and ML are transforming supply chain analytics by enabling more accurate forecasting, automated decision-making, and proactive risk management.
- Blockchain Technology: Blockchain can improve transparency and traceability within the supply chain, enhancing security and accountability.
- Digital Twins: Digital twins create virtual representations of physical supply chains, allowing for simulation and optimization before real-world implementation.
Exploring the Connection Between Data Visualization and Supply Chain Analytics:
Data visualization plays a crucial role in supply chain analytics. It transforms complex data sets into easily understandable charts, graphs, and dashboards, making insights readily accessible to stakeholders at all levels of the organization. Effective data visualization enables better communication of findings, facilitates quicker decision-making, and improves collaboration across different departments.
Key Factors to Consider:
- Roles and Real-World Examples: Data visualization tools, such as Tableau and Power BI, are commonly used to create interactive dashboards that display key supply chain KPIs. This allows managers to monitor performance, identify trends, and react quickly to potential problems. For example, a retailer might use a dashboard to monitor inventory levels, sales data, and shipping times to identify potential stockouts or delays.
- Risks and Mitigations: Poorly designed visualizations can lead to misinterpretations and incorrect decisions. It's crucial to ensure visualizations are clear, accurate, and easy to understand. Using appropriate chart types for the type of data being presented is essential.
- Impact and Implications: Effective data visualization can significantly improve communication, collaboration, and decision-making within the supply chain. It allows stakeholders to quickly grasp complex information and make data-driven decisions, leading to improved efficiency, cost savings, and enhanced customer satisfaction.
Conclusion: Reinforcing the Connection:
The connection between data visualization and supply chain analytics is inseparable. Data visualization is the critical bridge that connects complex data analysis with actionable insights and effective communication within the organization. By leveraging effective visualization techniques, organizations can unlock the full potential of their supply chain data, driving significant improvements in efficiency, resilience, and profitability.
Further Analysis: Examining Data Visualization Tools in Greater Detail:
Various data visualization tools cater to different needs and budgets. Some popular choices include Tableau, Power BI, Qlik Sense, and Google Data Studio. The selection of a suitable tool depends on factors such as the size of the organization, the complexity of the data, and the technical expertise of the users. Each tool offers unique features and capabilities, ranging from basic charting to advanced analytics and predictive modeling.
FAQ Section: Answering Common Questions About Supply Chain Analytics:
- What is the difference between supply chain management and supply chain analytics? Supply chain management is the overall process of managing the flow of goods and services, while supply chain analytics is the application of data analysis techniques to improve decision-making within that process.
- How much does implementing supply chain analytics cost? The cost varies significantly depending on the size and complexity of the organization, the scope of the implementation, and the technology chosen.
- What are the key benefits of using supply chain analytics? Benefits include improved forecasting accuracy, reduced inventory costs, enhanced supply chain visibility, optimized logistics, and increased profitability.
- What skills are needed for a career in supply chain analytics? Skills include strong analytical abilities, programming skills (e.g., Python, R), knowledge of statistical modeling, and experience with data visualization tools.
Practical Tips: Maximizing the Benefits of Supply Chain Analytics:
- Start with a clear objective: Define the specific business problems you want to solve using supply chain analytics.
- Assess your data: Evaluate the quality, completeness, and accessibility of your data.
- Choose the right tools: Select appropriate analytical tools and technologies based on your needs and budget.
- Develop internal expertise: Invest in training and development to build internal expertise in supply chain analytics.
- Start small and iterate: Begin with a pilot project to test and refine your approach before scaling up.
Final Conclusion: Wrapping Up with Lasting Insights:
Supply chain analytics is no longer a futuristic concept; it's a critical capability for organizations striving for operational excellence and sustainable competitive advantage. By embracing data-driven decision-making, businesses can anticipate disruptions, optimize their operations, and enhance their resilience in an increasingly volatile global marketplace. The journey requires a strategic approach, careful planning, and a commitment to continuous improvement, but the rewards—in terms of efficiency, profitability, and enhanced customer satisfaction—are substantial.
Latest Posts
Latest Posts
-
Advance Refunding Definition
Apr 30, 2025
-
Advance Premium Fund Definition
Apr 30, 2025
-
Advance Premium Definition
Apr 30, 2025
-
Advance Funded Pension Plan Definition
Apr 30, 2025
-
Advance Commitment Definition
Apr 30, 2025
Related Post
Thank you for visiting our website which covers about What Is Supply Chain Analytics . We hope the information provided has been useful to you. Feel free to contact us if you have any questions or need further assistance. See you next time and don't miss to bookmark.