What Is Big Data Definition How It Works And Uses
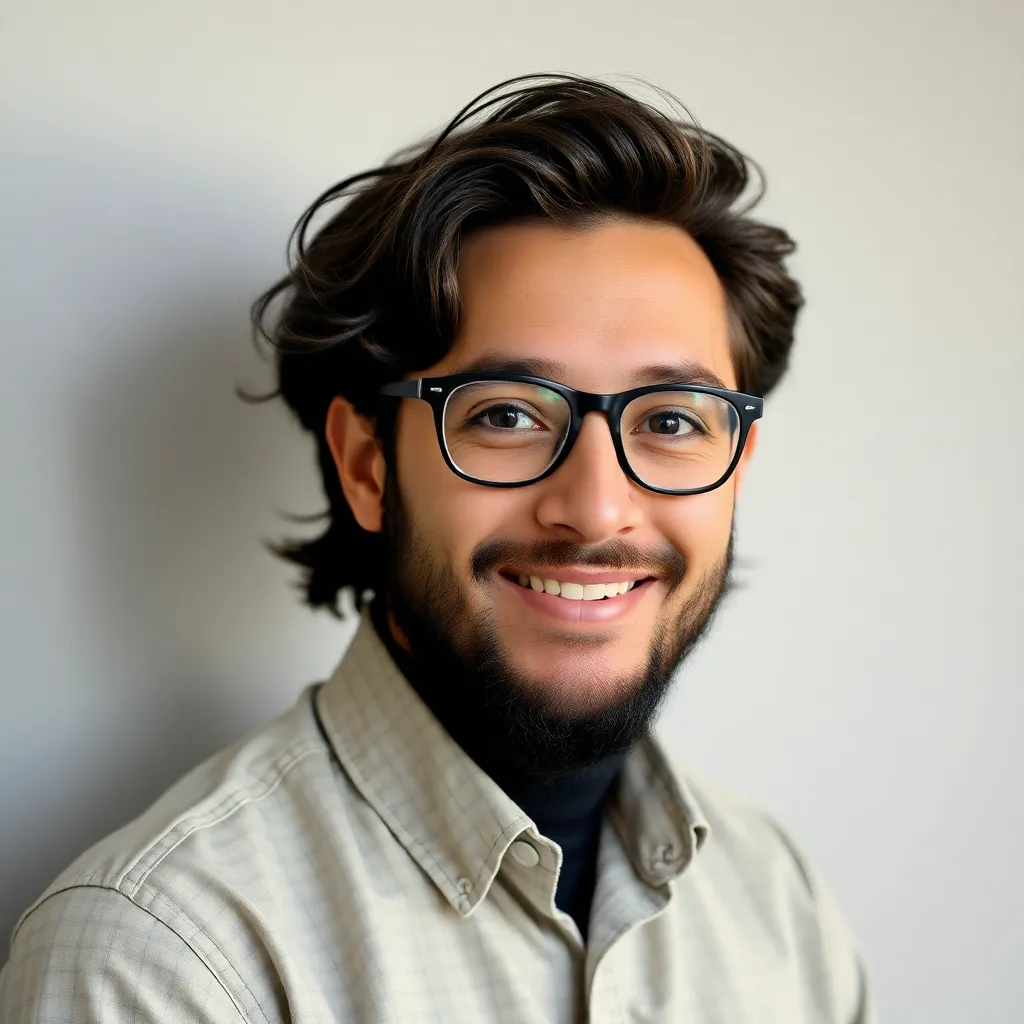
adminse
Apr 18, 2025 · 9 min read

Table of Contents
Unlocking the Power of Big Data: Definition, Mechanisms, and Applications
What if the future of decision-making hinges on our ability to harness the power of big data? This transformative resource is already revolutionizing industries and empowering organizations with unprecedented insights.
Editor’s Note: This article on big data provides a comprehensive overview of its definition, workings, and diverse applications. Published today, it offers up-to-date insights into this rapidly evolving field.
Why Big Data Matters:
Big data is no longer a futuristic concept; it's a present-day reality shaping businesses, governments, and our daily lives. Its relevance stems from its potential to uncover hidden patterns, predict future trends, and optimize processes across numerous sectors. From personalized recommendations on e-commerce platforms to sophisticated fraud detection systems in finance, big data's practical applications are vast and transformative. Its impact on industries ranges from improved healthcare outcomes through predictive diagnostics to enhanced customer experiences through targeted marketing campaigns. The ability to analyze massive datasets enables more informed decision-making, leading to increased efficiency, cost savings, and competitive advantages.
Overview: What This Article Covers:
This article will delve into the core aspects of big data, exploring its precise definition, the underlying mechanisms that enable its processing and analysis, and a wide range of its practical applications across diverse industries. Readers will gain a comprehensive understanding of big data's significance, potential challenges, and future implications, supported by data-driven research and real-world examples.
The Research and Effort Behind the Insights:
This article is the culmination of extensive research, drawing upon insights from leading industry experts, academic publications, and credible data sources. The information presented is rigorously vetted to ensure accuracy and reliability, providing readers with trustworthy and actionable insights.
Key Takeaways:
- Definition and Core Concepts: A clear understanding of big data's characteristics and its defining features (volume, velocity, variety, veracity, and value).
- Data Processing and Analysis: An overview of the technologies and techniques employed to handle and analyze big data effectively.
- Applications Across Industries: Exploration of how big data is being implemented across various sectors, such as healthcare, finance, retail, and marketing.
- Challenges and Solutions: Identification of the key obstacles associated with big data and strategies to overcome them.
- Future Implications: An examination of the potential long-term impact of big data on innovation, business strategies, and societal trends.
Smooth Transition to the Core Discussion:
Having established the importance of big data, let's now delve deeper into its intricacies, exploring its definition, the mechanisms behind its processing, and its widespread applications.
Exploring the Key Aspects of Big Data:
1. Definition and Core Concepts:
Big data is often defined by its five Vs:
- Volume: The sheer size of the data involved, often exceeding the capacity of traditional data processing tools. We're talking terabytes, petabytes, and even exabytes of information.
- Velocity: The speed at which data is generated and processed. Data streams in from various sources at an incredible rate, demanding real-time or near real-time analysis.
- Variety: The diverse range of data formats, including structured data (like databases), semi-structured data (like XML files), and unstructured data (like text, images, and videos).
- Veracity: The trustworthiness and quality of the data. Inaccurate or incomplete data can lead to flawed insights, highlighting the importance of data validation and cleaning.
- Value: The ultimate goal—extracting meaningful insights and actionable intelligence from the data to drive better decision-making.
These five Vs represent the fundamental characteristics that distinguish big data from traditional data sets. The challenge lies not just in storing this massive volume of data but also in processing it quickly enough to extract value.
2. Data Processing and Analysis:
Handling big data requires specialized technologies and techniques. Key components include:
- Hadoop: An open-source framework for storing and processing large datasets across clusters of computers. It provides scalability and fault tolerance, crucial for handling big data's volume and velocity.
- Spark: A fast and general-purpose cluster computing system, often used in conjunction with Hadoop for faster data processing. It improves performance significantly compared to traditional map-reduce approaches.
- NoSQL Databases: These databases are designed to handle large volumes of unstructured or semi-structured data more efficiently than traditional relational databases. Examples include MongoDB, Cassandra, and Redis.
- Cloud Computing: Cloud platforms like AWS, Azure, and Google Cloud provide the infrastructure and resources needed to store and process big data cost-effectively.
- Data Mining and Machine Learning: These advanced analytical techniques are used to extract patterns, predictions, and insights from the vast datasets. Algorithms such as regression, classification, and clustering are commonly employed.
3. Applications Across Industries:
Big data's applications are far-reaching and continuously expanding:
- Healthcare: Predictive analytics can identify patients at high risk of developing certain diseases, enabling proactive interventions. Big data also assists in drug discovery, personalized medicine, and improving operational efficiency in hospitals.
- Finance: Fraud detection systems rely heavily on big data to identify suspicious transactions in real-time. Risk management, algorithmic trading, and customer profiling are other key applications.
- Retail: Personalized recommendations, targeted advertising, inventory management, and supply chain optimization are all enhanced by analyzing customer purchase history and market trends.
- Marketing: Big data enables companies to understand customer behavior better, leading to more effective marketing campaigns and improved customer engagement.
- Transportation: Optimizing traffic flow, predicting delays, improving public transportation efficiency, and developing self-driving car technology all leverage big data analytics.
- Manufacturing: Predictive maintenance, quality control, and supply chain optimization are significantly improved through the analysis of sensor data and production records.
4. Challenges and Solutions:
Despite its potential, big data presents several challenges:
- Data Security and Privacy: Protecting sensitive data from unauthorized access and breaches is paramount. Robust security measures and compliance with regulations like GDPR are essential.
- Data Quality and Cleaning: Inaccurate or incomplete data can lead to flawed insights. Data cleaning and validation are crucial steps in the process.
- Data Integration: Combining data from multiple sources can be complex, requiring specialized tools and techniques.
- Scalability and Cost: Processing and storing massive datasets can be expensive and require significant computational resources. Cloud computing can help mitigate these costs.
- Skills Gap: There's a growing demand for professionals with the skills to work with big data, leading to a skills gap in the industry.
5. Future Implications:
Big data's influence will continue to grow, impacting various aspects of our lives:
- Artificial Intelligence (AI) and Machine Learning (ML): Big data fuels the development and advancement of AI and ML, enabling more sophisticated algorithms and applications.
- Internet of Things (IoT): The proliferation of connected devices generates enormous amounts of data, further expanding the scope of big data analytics.
- Automation and Robotics: Big data plays a critical role in automating processes and improving the efficiency of robotic systems.
- Personalized Experiences: Big data enables the creation of personalized experiences across various sectors, from entertainment to healthcare.
- Predictive Modeling and Forecasting: Improved predictive models based on big data can help organizations anticipate future trends and make more informed decisions.
Exploring the Connection Between Data Governance and Big Data:
The relationship between data governance and big data is critical. Effective data governance ensures the quality, security, and compliance of the vast datasets used in big data analytics. Without proper governance, organizations risk making decisions based on flawed or unreliable data, leading to inaccurate predictions and potentially harmful outcomes.
Key Factors to Consider:
- Roles and Real-World Examples: Data governance frameworks define roles and responsibilities for data management, ensuring data quality and security. For example, data stewards ensure data quality, while data owners are responsible for the accuracy and completeness of their data.
- Risks and Mitigations: Poor data governance increases the risk of data breaches, regulatory fines, and reputational damage. Implementing robust data governance policies, regular audits, and employee training can mitigate these risks.
- Impact and Implications: Effective data governance fosters trust in data-driven decisions, improving business outcomes and enhancing organizational performance. Conversely, poor data governance can erode trust and undermine the credibility of big data analytics.
Conclusion: Reinforcing the Connection:
The interplay between data governance and big data is essential for successful big data initiatives. By establishing robust data governance frameworks, organizations can maximize the value of their data while mitigating risks and ensuring compliance.
Further Analysis: Examining Data Security in Greater Detail:
Data security is a paramount concern when dealing with big data. The sheer volume and sensitivity of the data make it a prime target for cyberattacks. Effective security measures include encryption, access controls, intrusion detection systems, and regular security audits. Compliance with relevant data protection regulations is also crucial. Organizations must invest in robust security infrastructure and training to protect their big data assets.
FAQ Section: Answering Common Questions About Big Data:
-
What is big data? Big data refers to extremely large and complex datasets that are difficult to process using traditional data processing tools. It's characterized by the five Vs: volume, velocity, variety, veracity, and value.
-
How is big data used in different industries? Big data's applications are vast and vary by industry. Examples include fraud detection in finance, personalized medicine in healthcare, targeted advertising in marketing, and predictive maintenance in manufacturing.
-
What are the challenges of working with big data? Challenges include data security, data quality, data integration, scalability, cost, and the skills gap.
-
What technologies are used to process big data? Hadoop, Spark, NoSQL databases, and cloud computing platforms are commonly used.
Practical Tips: Maximizing the Benefits of Big Data:
- Define clear business objectives: Identify specific business problems that big data can help solve.
- Invest in the right infrastructure and technology: Choose appropriate tools and platforms based on your needs and resources.
- Ensure data quality and governance: Implement robust data quality and governance policies to ensure data accuracy and security.
- Develop the necessary skills and expertise: Train your workforce to effectively utilize big data technologies and techniques.
- Start small and scale gradually: Begin with a pilot project to test and refine your approach before scaling to larger datasets.
Final Conclusion: Wrapping Up with Lasting Insights:
Big data is a transformative force with the potential to revolutionize industries and improve decision-making across various sectors. By understanding its definition, processing mechanisms, and diverse applications, organizations can leverage its power to gain a competitive edge and achieve significant business outcomes. However, addressing the associated challenges, including data security, quality, and governance, is crucial for realizing the full potential of big data while ensuring responsible and ethical use. The future of informed decision-making undoubtedly lies in effectively harnessing the power of big data.
Latest Posts
Related Post
Thank you for visiting our website which covers about What Is Big Data Definition How It Works And Uses . We hope the information provided has been useful to you. Feel free to contact us if you have any questions or need further assistance. See you next time and don't miss to bookmark.