What Is A Mean Definition In Math And Formula For Calculation
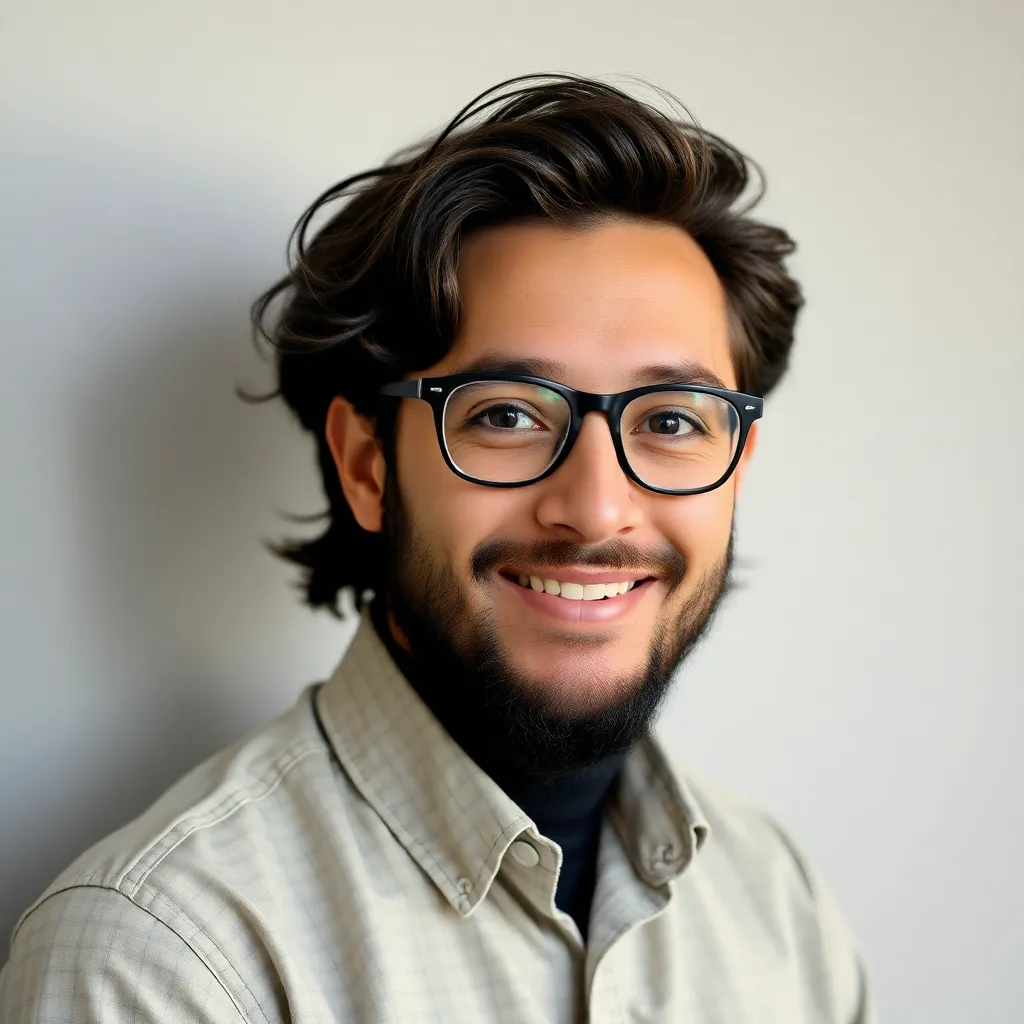
adminse
Apr 21, 2025 · 8 min read

Table of Contents
Decoding the Mean: A Comprehensive Guide to Definition, Calculation, and Applications
What if our understanding of central tendency hinges on truly grasping the concept of the mean? This fundamental statistical measure underpins countless applications, from analyzing financial markets to predicting weather patterns.
Editor’s Note: This article provides a comprehensive exploration of the mean in mathematics, covering its various forms, calculation methods, and practical applications. Updated for accuracy and relevance, it serves as a valuable resource for students, researchers, and anyone seeking a deeper understanding of this crucial statistical concept.
Why the Mean Matters: A Foundation of Data Analysis
The mean, often referred to as the average, is a cornerstone of descriptive statistics. It represents the central tendency of a dataset, providing a single value that summarizes the overall distribution of the data points. Understanding the mean is crucial for various reasons:
- Data Summarization: The mean provides a concise representation of a large dataset, making it easier to interpret and compare different datasets.
- Decision-Making: In numerous fields, the mean serves as a key input for decision-making processes, ranging from business strategies to scientific research.
- Predictive Modeling: The mean is frequently used in predictive models to estimate future outcomes based on past data trends.
- Identifying Outliers: By comparing individual data points to the mean, one can identify outliers – data points that significantly deviate from the average.
- Comparing Groups: The mean allows for efficient comparison of different groups or populations, facilitating meaningful insights.
Overview: What This Article Covers
This article will systematically explore the different types of means, their respective formulas, and appropriate applications. We will delve into the arithmetic mean, geometric mean, harmonic mean, and weighted mean, providing clear explanations and illustrative examples. Furthermore, the article will address the limitations of the mean and discuss situations where other measures of central tendency might be more appropriate. Finally, practical applications across diverse fields will be examined.
The Research and Effort Behind the Insights
This article draws upon established mathematical principles and statistical concepts, referencing standard textbooks and authoritative sources. The explanations are meticulously crafted to ensure clarity and accuracy, while the examples are designed to enhance understanding and illustrate practical application.
Key Takeaways:
- Definition and Calculation of Various Means: A clear understanding of the arithmetic, geometric, harmonic, and weighted means, including their respective formulas.
- Applications Across Disciplines: Real-world examples showcasing the use of means in finance, science, engineering, and other fields.
- Limitations and Alternatives: Awareness of situations where the mean might not be the most appropriate measure of central tendency and exploration of suitable alternatives.
- Interpreting Results: Guidance on effectively interpreting and utilizing mean values in different contexts.
Smooth Transition to the Core Discussion
Now that we understand the significance of the mean, let’s delve into the specifics of its different forms and calculation methods.
Exploring the Key Aspects of the Mean
The term "mean" encompasses several variations, each suited to different data types and applications. Let's examine the most common types:
1. Arithmetic Mean:
This is the most commonly understood and used type of mean. It's simply the sum of all values in a dataset divided by the number of values.
-
Formula: Arithmetic Mean (AM) = Σx / n
Where:
- Σx represents the sum of all data points (x₁, x₂, x₃... xₙ)
- n represents the total number of data points.
-
Example: Consider the dataset: {2, 4, 6, 8, 10}. AM = (2 + 4 + 6 + 8 + 10) / 5 = 6
-
Applications: The arithmetic mean is widely used in various fields like calculating average scores, average income, average temperature, etc.
2. Geometric Mean:
The geometric mean is particularly useful for data representing rates of change or multiplicative factors. It's calculated by multiplying all values in a dataset and then taking the nth root, where n is the number of values.
-
Formula: Geometric Mean (GM) = (x₁ * x₂ * x₃... * xₙ)^(1/n)
-
Example: Consider the dataset: {2, 4, 8}. GM = (2 * 4 * 8)^(1/3) = 4
-
Applications: The geometric mean finds application in finance (calculating average investment returns), demographics (calculating average population growth rates), and other areas involving multiplicative data.
3. Harmonic Mean:
The harmonic mean is best suited for data representing rates or ratios, especially when dealing with inversely proportional relationships. It's the reciprocal of the arithmetic mean of the reciprocals of the data points.
-
Formula: Harmonic Mean (HM) = n / (Σ(1/x))
-
Example: Consider the dataset: {2, 4, 8}. HM = 3 / (1/2 + 1/4 + 1/8) ≈ 3.43
-
Applications: The harmonic mean is commonly used in calculating average speeds, average resistances in parallel circuits, and other scenarios where reciprocals are relevant.
4. Weighted Mean:
The weighted mean assigns different weights to different data points based on their relative importance. This is useful when some data points are more significant than others.
-
Formula: Weighted Mean (WM) = Σ(wᵢ * xᵢ) / Σwᵢ
Where:
- wᵢ represents the weight assigned to the ith data point (xᵢ).
-
Example: Consider a student's grades: 80 (weight 2), 90 (weight 3), 70 (weight 1). WM = (280 + 390 + 1*70) / (2 + 3 + 1) = 83.33
-
Applications: Weighted means are widely used in academic grading systems, portfolio management (weighted average of asset returns), and other areas where data points have varying levels of importance.
Closing Insights: Summarizing the Core Discussion
The mean, in its various forms, is a powerful tool for summarizing and analyzing data. Understanding the nuances of each type—arithmetic, geometric, harmonic, and weighted—is crucial for choosing the appropriate method based on the nature of the dataset and the specific objectives of the analysis.
Exploring the Connection Between Standard Deviation and the Mean
The standard deviation and the mean are intrinsically linked. While the mean provides the central tendency, the standard deviation quantifies the dispersion or spread of the data around the mean. A high standard deviation indicates a wide spread of data points, whereas a low standard deviation suggests that data points cluster closely around the mean. Together, the mean and standard deviation provide a comprehensive picture of the data distribution.
Key Factors to Consider:
-
Roles and Real-World Examples: Standard deviation measures how much individual data points deviate from the mean. For example, in finance, high standard deviation in stock prices signifies greater risk.
-
Risks and Mitigations: A large standard deviation can make the mean a less reliable measure of central tendency, as it might be heavily influenced by outliers. Robust statistical methods can mitigate this.
-
Impact and Implications: The interplay between mean and standard deviation is critical for understanding data variability and making informed decisions based on this understanding. A high standard deviation warns against over-reliance on the mean alone.
Conclusion: Reinforcing the Connection
The relationship between standard deviation and mean is crucial in statistical analysis. While the mean indicates the center, the standard deviation reveals the data's spread, providing a more complete understanding of the data distribution and its reliability.
Further Analysis: Examining Standard Deviation in Greater Detail
Standard deviation is calculated by first finding the variance (the average of the squared differences from the mean) and then taking its square root. This process ensures that all deviations are positive and emphasizes larger deviations. Understanding the calculation and interpretation of standard deviation is fundamental for proper data analysis.
FAQ Section: Answering Common Questions About the Mean
-
What is the best type of mean to use? The optimal choice depends on the data type and the research question. The arithmetic mean is most common, but geometric and harmonic means are better suited for specific situations (multiplicative or rate data respectively).
-
How are outliers handled when calculating the mean? Outliers can significantly skew the arithmetic mean. Robust statistical methods like the median or trimmed mean can be used as alternatives.
-
Can the mean be used with categorical data? No, the mean is only applicable to numerical data. For categorical data, other measures of central tendency like the mode are more suitable.
-
What if the dataset has missing values? Missing data needs to be addressed before calculating the mean. Methods like imputation (estimating missing values) can be used, but this requires caution.
Practical Tips: Maximizing the Benefits of Using the Mean
-
Visualize your data: Before calculating the mean, create a histogram or box plot to visually inspect the data distribution and identify potential outliers.
-
Consider the context: The choice of mean depends heavily on the context. Understanding the nature of your data and research question is paramount.
-
Report both the mean and standard deviation: This provides a more complete picture of the data distribution.
-
Be aware of potential biases: Outliers can significantly affect the mean. Investigate potential reasons for outliers and consider appropriate handling strategies.
Final Conclusion: Wrapping Up with Lasting Insights
The mean, in its various forms, is an essential statistical concept with widespread applications across numerous fields. By understanding the different types of means, their calculation methods, limitations, and appropriate use cases, individuals and organizations can harness this powerful tool for data summarization, analysis, and informed decision-making. The mean isn't just a number; it's a key to unlocking insights hidden within data.
Latest Posts
Latest Posts
-
Merton Model Definition History Formula What It Tells You
Apr 22, 2025
-
What Are The Advantages And Disadvantages Of Issuing New Equity In The Capital Structure
Apr 22, 2025
-
Why Companies Do Not Have Conservative Capital Structure
Apr 22, 2025
-
Merger Definition How It Works With Types And Examples
Apr 22, 2025
-
Merger Securities Definition
Apr 22, 2025
Related Post
Thank you for visiting our website which covers about What Is A Mean Definition In Math And Formula For Calculation . We hope the information provided has been useful to you. Feel free to contact us if you have any questions or need further assistance. See you next time and don't miss to bookmark.