Representative Sample Definition Importance And Examples
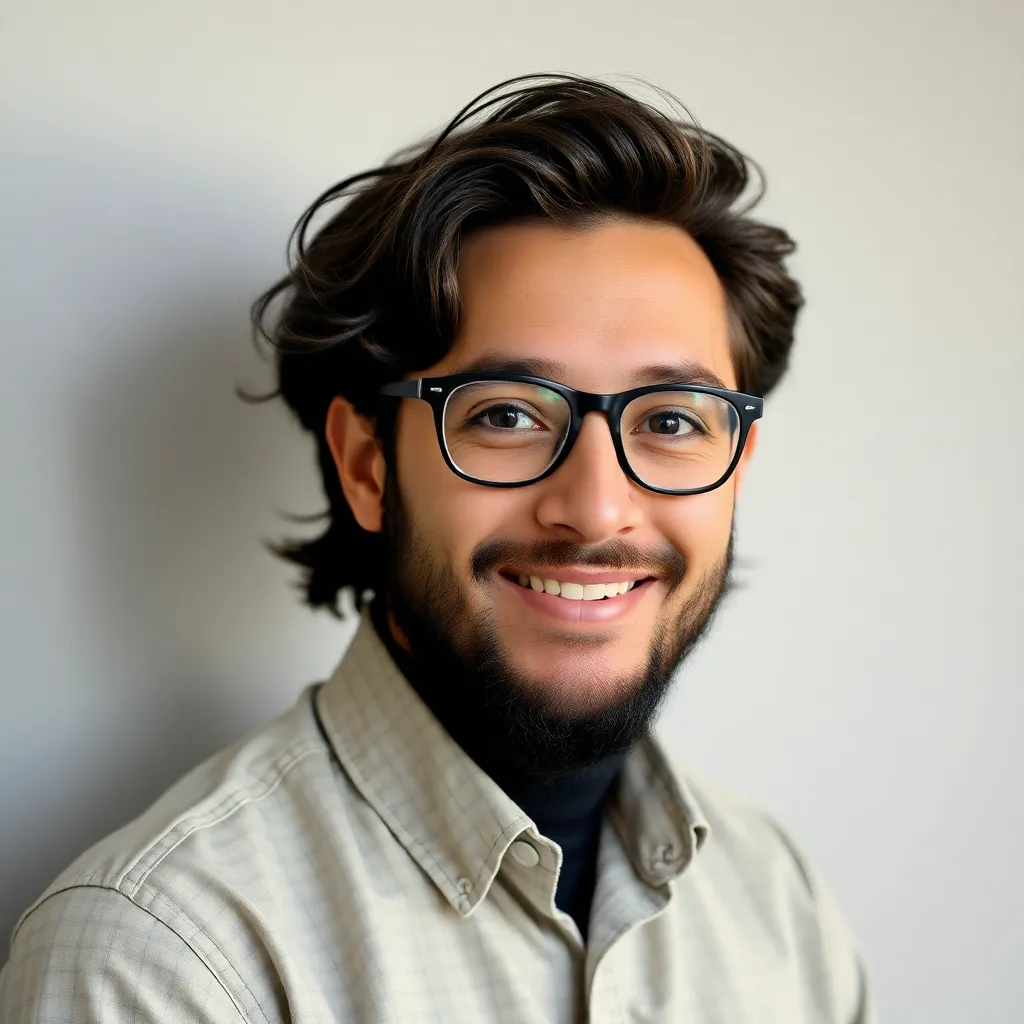
adminse
Apr 28, 2025 · 9 min read

Table of Contents
Unlocking Insights: The Power of Representative Samples
What if the accuracy of your conclusions hinges entirely on the sample you choose? Representative sampling is the cornerstone of reliable data analysis, offering invaluable insights across diverse fields.
Editor’s Note: This article on representative sample definition, importance, and examples was published today, providing readers with up-to-date information and practical applications in various fields.
Why Representative Samples Matter: Relevance, Practical Applications, and Industry Significance
Representative sampling is not just a statistical concept; it's a fundamental principle underpinning credible research and informed decision-making. Across industries, from market research and healthcare to political polling and environmental studies, the ability to extrapolate findings from a smaller group to a larger population depends entirely on the representativeness of the sample. A well-chosen sample allows researchers to draw inferences with confidence, minimizing bias and maximizing the validity of their conclusions. Failing to use a representative sample can lead to inaccurate conclusions, wasted resources, and potentially harmful decisions. The cost of an unrepresentative sample can be far greater than the effort invested in selecting a representative one.
Overview: What This Article Covers
This article provides a comprehensive exploration of representative samples. It delves into the precise definition of a representative sample, explores its crucial importance in various fields, and presents numerous real-world examples to illustrate its practical application. Furthermore, we will examine the methods used to achieve representativeness, the potential pitfalls to avoid, and the impact of sample size on the accuracy of results. Finally, we will address frequently asked questions and offer practical tips for selecting and utilizing representative samples effectively.
The Research and Effort Behind the Insights
This article draws upon extensive research from leading statistical textbooks, peer-reviewed journal articles, and reputable online resources. The information presented is based on established statistical principles and supported by real-world examples to demonstrate the practical implications of representative sampling. The aim is to provide readers with a clear, concise, and accurate understanding of this critical topic.
Key Takeaways:
- Definition and Core Concepts: A clear understanding of what constitutes a representative sample and the key principles involved.
- Practical Applications: Numerous examples illustrating the use of representative sampling across diverse fields.
- Methods for Achieving Representativeness: A detailed exploration of sampling techniques designed to ensure representativeness.
- Challenges and Limitations: An examination of potential pitfalls and biases that can compromise the representativeness of a sample.
- Impact of Sample Size: Understanding the relationship between sample size and the accuracy of results.
Smooth Transition to the Core Discussion
Having established the importance of representative sampling, let's now delve into a detailed examination of its core concepts, practical applications, and the methods employed to achieve representative samples.
Exploring the Key Aspects of Representative Samples
Definition and Core Concepts:
A representative sample is a subset of a population that accurately reflects the characteristics of the entire population from which it is drawn. In other words, the sample's composition mirrors the population's composition in terms of key variables relevant to the study. These variables could include age, gender, ethnicity, income, location, or any other factor that might influence the results. The goal is to minimize sampling error—the difference between the characteristics of the sample and the characteristics of the population. A truly representative sample allows researchers to generalize their findings from the sample to the larger population with a high degree of confidence.
Applications Across Industries:
The applications of representative sampling are incredibly broad and span numerous fields:
-
Market Research: Companies use representative samples to understand consumer preferences, predict market trends, and test the effectiveness of marketing campaigns. For example, a company launching a new product might survey a representative sample of consumers to gauge interest and identify potential improvements.
-
Political Polling: Politicians and pollsters rely on representative samples to gauge public opinion, predict election outcomes, and tailor their campaigns effectively. A poll that doesn't use a representative sample can significantly misrepresent public sentiment.
-
Healthcare: Researchers use representative samples in clinical trials to test the efficacy and safety of new drugs and treatments. The results of these trials are then used to inform medical practice and policy.
-
Environmental Studies: Scientists use representative samples to monitor environmental conditions, assess pollution levels, and study the impact of human activities on the environment. For example, water samples from different locations within a river system might be analyzed to assess water quality.
-
Social Sciences: Sociologists and other social scientists use representative samples to study social trends, attitudes, and behaviors. This allows researchers to draw conclusions about the entire population based on the data collected from the sample.
Challenges and Solutions:
Achieving a truly representative sample can be challenging. Several factors can introduce bias and compromise the representativeness of a sample:
-
Sampling Bias: This occurs when certain groups within the population are over-represented or under-represented in the sample. This can lead to inaccurate and misleading conclusions.
-
Non-response Bias: This occurs when individuals selected for the sample refuse to participate or cannot be contacted. This can skew the results if non-respondents differ significantly from respondents.
-
Selection Bias: This occurs when the selection process itself favors certain groups over others. For example, relying solely on online surveys can exclude those without internet access.
To mitigate these challenges, researchers employ various techniques:
-
Stratified Sampling: The population is divided into subgroups (strata) based on relevant characteristics, and then a random sample is selected from each stratum. This ensures representation from all subgroups.
-
Cluster Sampling: The population is divided into clusters (e.g., geographic areas), and then a random sample of clusters is selected. All individuals within the selected clusters are included in the sample.
-
Random Sampling: Every individual in the population has an equal chance of being selected for the sample. This is a fundamental technique for minimizing bias.
Impact on Innovation:
Representative sampling is a crucial driver of innovation across various fields. By providing accurate and reliable data, it enables researchers to develop new products, treatments, policies, and technologies that are more effective and relevant to the needs of the population. The use of accurate data ensures better decision-making that improves processes, products, services, and overall outcomes.
Closing Insights: Summarizing the Core Discussion
Representative sampling is not merely a statistical tool; it's a critical foundation for reliable research and evidence-based decision-making. Its applications are vast, impacting virtually every field that seeks to understand and act upon the characteristics of a population. By employing appropriate sampling techniques and addressing potential biases, researchers can extract valuable insights from smaller samples, leading to more accurate conclusions and ultimately more impactful innovations.
Exploring the Connection Between Sample Size and Representative Samples
The relationship between sample size and the representativeness of a sample is complex. While a larger sample generally increases the chances of representativeness, it's not a guarantee. A large sample can still be biased if the sampling method is flawed. However, a larger sample size reduces the margin of error, providing greater confidence in the accuracy of the results. The appropriate sample size depends on several factors, including the population size, the desired level of precision, and the variability within the population. Statistical power calculations help determine the minimum sample size needed to detect meaningful differences or effects with a specified level of confidence.
Key Factors to Consider:
-
Roles and Real-World Examples: A larger sample size reduces sampling error, leading to more precise estimations of population parameters. For example, in political polling, a larger sample size provides a more accurate prediction of election outcomes.
-
Risks and Mitigations: While a larger sample is generally beneficial, excessively large samples can be costly and inefficient. Careful planning and resource allocation are crucial to balance sample size with practical constraints.
-
Impact and Implications: The sample size directly impacts the precision and reliability of research findings. Insufficient sample sizes can lead to inaccurate conclusions and undermine the validity of research.
Conclusion: Reinforcing the Connection
The optimal sample size is not simply a matter of "bigger is better". It's a balance between achieving sufficient precision and managing practical limitations like cost and time constraints. Understanding this relationship is critical for researchers and decision-makers to obtain valid and reliable results.
Further Analysis: Examining Sampling Techniques in Greater Detail
Several sophisticated sampling techniques are designed to enhance the representativeness of samples. These include:
- Systematic Sampling: Selecting individuals at regular intervals from a ranked list of the population.
- Quota Sampling: Ensuring the sample reflects the population's proportions across specific characteristics.
- Convenience Sampling: Selecting individuals easily accessible, although this method is prone to bias and should generally be avoided for critical research.
Each technique has its strengths and weaknesses, and the choice of method depends on the specific research question, the available resources, and the characteristics of the population.
FAQ Section: Answering Common Questions About Representative Samples
Q: What is the difference between a representative sample and a random sample?
A: While all representative samples aim to mirror the population, a random sample is one method of achieving representativeness. Random sampling ensures every member of the population has an equal chance of selection. However, other techniques, like stratified sampling, can also produce representative samples even without relying solely on random selection.
Q: How can I ensure my sample is truly representative?
A: Careful planning is crucial. Define the target population precisely. Choose an appropriate sampling method to address potential biases. Consider the sample size requirements based on statistical power calculations. And finally, rigorously analyze the data to identify any potential biases or anomalies.
Q: What happens if my sample isn't representative?
A: The results will be biased and potentially misleading. The conclusions drawn may not accurately reflect the characteristics of the population, leading to flawed decisions and wasted resources.
Practical Tips: Maximizing the Benefits of Representative Sampling
- Clearly define your target population: Be specific about who you're trying to represent.
- Select an appropriate sampling method: Choose the method that best suits your research question and resources.
- Determine the required sample size: Use statistical power calculations to ensure sufficient precision.
- Monitor for potential biases: Be vigilant for any factors that might skew your results.
- Analyze your data thoroughly: Look for outliers and patterns that might suggest bias.
Final Conclusion: Wrapping Up with Lasting Insights
Representative sampling is the cornerstone of reliable data analysis and evidence-based decision-making. By understanding its principles, employing appropriate techniques, and carefully managing potential biases, researchers and decision-makers can extract valuable insights from data, leading to more accurate predictions, more effective interventions, and ultimately, a more informed understanding of the world around us. The investment in achieving a representative sample is far outweighed by the cost of drawing erroneous conclusions from a biased sample. The pursuit of accurate, reliable data is fundamental to progress in all fields.
Latest Posts
Latest Posts
-
How Often Can You Refinance Your Student Loans
Apr 28, 2025
-
How To Get Student Loans Off My Credit Report
Apr 28, 2025
-
Retail Sales Definition Measurement As An Economic Indicator
Apr 28, 2025
-
Retail Repurchase Agreement Definition
Apr 28, 2025
-
I Just Paid Off My Student Loans Now What
Apr 28, 2025
Related Post
Thank you for visiting our website which covers about Representative Sample Definition Importance And Examples . We hope the information provided has been useful to you. Feel free to contact us if you have any questions or need further assistance. See you next time and don't miss to bookmark.