Positive Skewness Definition
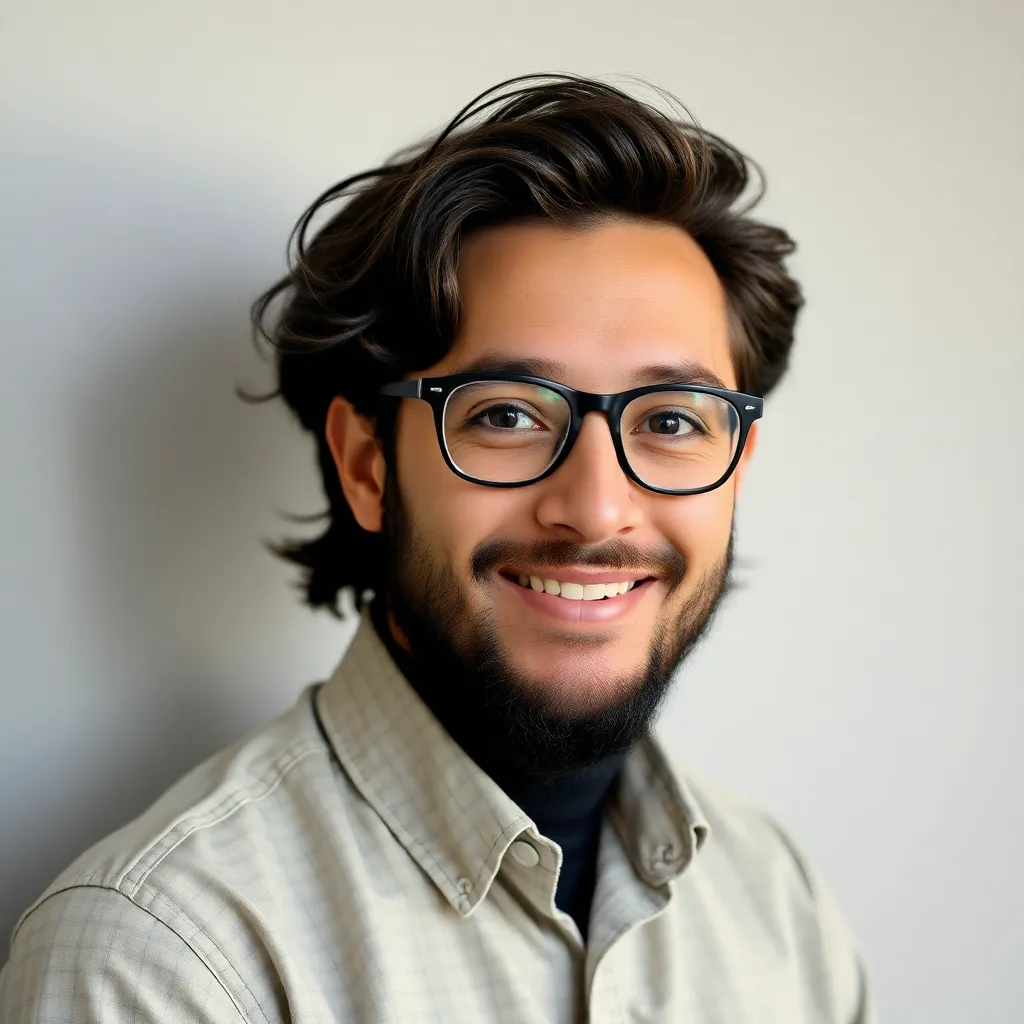
adminse
Mar 28, 2025 · 9 min read

Table of Contents
Understanding Positive Skewness: A Deep Dive into Right-Skewed Distributions
What if understanding positive skewness unlocks a deeper comprehension of data analysis and decision-making? This critical statistical concept is fundamental to interpreting data accurately and drawing meaningful conclusions.
Editor’s Note: This article on positive skewness provides a comprehensive overview of the concept, its implications, and practical applications across various fields. Updated insights and real-world examples ensure readers gain a thorough understanding of this important statistical measure.
Why Positive Skewness Matters:
Positive skewness, also known as right skewness, is a crucial concept in statistics and data analysis. It describes a distribution where the tail on the right side is longer or fatter than the tail on the left. This asymmetry implies that a significant portion of the data points cluster towards the lower end of the scale, while a smaller number of exceptionally high values extend the distribution to the right. Understanding positive skewness is essential because it significantly impacts the interpretation of central tendency measures (mean, median, mode) and the overall understanding of the data's distribution. It's relevant across numerous fields, from finance (analyzing investment returns) to healthcare (understanding patient recovery times) and even marketing (evaluating customer satisfaction scores). Ignoring positive skewness can lead to flawed conclusions and inaccurate predictions.
Overview: What This Article Covers:
This article provides a detailed exploration of positive skewness. We will define the concept, explore its graphical representation, discuss its implications for descriptive statistics, examine its causes and real-world examples, and delve into how to handle positively skewed data in statistical analysis. Readers will gain a practical understanding of how to identify, interpret, and address positive skewness in their own data analysis.
The Research and Effort Behind the Insights:
This article is the product of extensive research, drawing upon established statistical textbooks, peer-reviewed journals, and practical applications across various disciplines. The information presented is supported by established statistical principles and real-world examples to ensure accuracy and clarity.
Key Takeaways:
- Definition and Core Concepts: A clear definition of positive skewness and its underlying principles.
- Graphical Representation: Understanding how positive skewness manifests in histograms and other visual representations.
- Impact on Descriptive Statistics: How positive skewness affects the mean, median, and mode, and the implications for interpretation.
- Causes of Positive Skewness: Exploring the factors that contribute to the development of right-skewed distributions.
- Real-World Examples: Illustrative examples of positive skewness across various fields.
- Handling Positively Skewed Data: Methods for transforming data and applying appropriate statistical techniques.
Smooth Transition to the Core Discussion:
Now that we understand the importance of positive skewness, let's delve into its core aspects, starting with a precise definition and moving towards practical applications and considerations.
Exploring the Key Aspects of Positive Skewness:
1. Definition and Core Concepts:
Positive skewness refers to a distribution where the tail on the right side (the higher values) is longer or heavier than the tail on the left. This means there are more data points concentrated towards the lower end of the distribution, with a few outliers pulling the tail to the right. The distribution is asymmetrical, deviating from a perfectly symmetrical bell curve (normal distribution). The skewness is quantified using statistical measures, with positive values indicating right skewness.
2. Graphical Representation:
A histogram provides a clear visual representation of positive skewness. In a positively skewed histogram, the majority of the bars are clustered on the left, creating a peak. As you move to the right, the bars become progressively shorter, indicating a gradual decrease in frequency. The tail extends significantly to the right, indicating the presence of high-value outliers. Other visual representations, such as box plots, also clearly show positive skewness through the position of the median relative to the mean and the length of the upper whisker compared to the lower whisker.
3. Impact on Descriptive Statistics:
Positive skewness significantly affects the interpretation of descriptive statistics:
- Mean: The mean is highly sensitive to outliers. In a positively skewed distribution, the presence of high-value outliers pulls the mean towards the right, making it larger than the median.
- Median: The median, being the middle value, is less sensitive to outliers and provides a more robust measure of central tendency in skewed distributions. In a positively skewed distribution, the median is typically smaller than the mean.
- Mode: The mode represents the most frequent value. In a positively skewed distribution, the mode is usually located towards the left, at the peak of the distribution.
The relationship between the mean, median, and mode is a key indicator of skewness. In a positively skewed distribution, the typical order is Mode < Median < Mean.
4. Causes of Positive Skewness:
Several factors can contribute to positive skewness in a dataset:
- Presence of Outliers: High-value outliers significantly stretch the right tail of the distribution.
- Natural Constraints: Some variables have a natural lower bound (e.g., zero for income or age), limiting values on the left, while the upper bound is less constrained.
- Measurement Scales: The specific measurement scale used can influence skewness. For instance, logarithmic transformations can reduce positive skewness.
- Data Collection Methods: Sampling bias or data entry errors can introduce skewness.
5. Real-World Examples:
Positive skewness is commonly observed across various fields:
- Income Distribution: Income data often exhibits positive skewness, with a majority of individuals earning relatively lower incomes, and a smaller number of high-income earners pulling the tail to the right.
- House Prices: Similarly, house prices often show positive skewness, with most houses falling within a certain price range, and a few luxury properties significantly increasing the average price.
- Investment Returns: Investment returns can be positively skewed, with most returns falling within a certain range, and some exceptionally high returns stretching the distribution to the right.
- Waiting Times: Waiting times in a queue or for a service can show positive skewness, with most people waiting for a relatively short period and a few experiencing unusually long delays.
- Customer Satisfaction Scores: Customer satisfaction scores often show positive skewness, with most customers reasonably satisfied, and a few reporting extremely low satisfaction scores.
6. Handling Positively Skewed Data:
Analyzing positively skewed data requires careful consideration. Simply using the mean might be misleading. Several techniques can address this:
- Data Transformation: Transforming the data using logarithmic, square root, or other transformations can reduce skewness and improve the normality of the distribution. This allows for the application of parametric statistical tests that assume normality.
- Non-parametric Tests: If data transformation is ineffective or inappropriate, non-parametric statistical tests, which don't assume normality, can be used. Examples include the Mann-Whitney U test and the Kruskal-Wallis test.
- Robust Statistical Measures: Using robust measures of central tendency, like the median, instead of the mean, can provide a more accurate representation of the central tendency in skewed distributions.
- Winsorizing or Trimming: Winsorizing replaces extreme outliers with less extreme values, while trimming removes outliers entirely. This can reduce the influence of outliers on statistical analysis.
Exploring the Connection Between Outliers and Positive Skewness:
Outliers play a significant role in creating positive skewness. A single exceptionally high value can dramatically stretch the right tail of the distribution, resulting in a long right tail and a positively skewed shape. Understanding this connection is crucial for interpreting data accurately and employing appropriate analytical techniques.
Key Factors to Consider:
Roles and Real-World Examples:
Outliers, as illustrated in the income distribution and house price examples above, are a prime driver of positive skewness. A small number of exceptionally high values disproportionately influences the mean, potentially misrepresenting the typical value.
Risks and Mitigations:
The risk of misinterpreting data due to positive skewness is substantial. Relying solely on the mean in such cases can lead to inaccurate conclusions. Mitigation strategies involve using robust measures like the median, transforming the data, or applying non-parametric tests.
Impact and Implications:
Misinterpreting positively skewed data can have far-reaching consequences. In finance, it might lead to flawed investment decisions. In healthcare, it could misrepresent the effectiveness of a treatment. Accurate identification and handling of positive skewness are crucial for reliable decision-making.
Conclusion: Reinforcing the Connection:
The relationship between outliers and positive skewness is undeniable. Outliers significantly distort the distribution, leading to a rightward skew. By understanding this connection and employing appropriate analytical methods, analysts can avoid misleading interpretations and make informed decisions based on accurate data analysis.
Further Analysis: Examining Outliers in Greater Detail:
Identifying and handling outliers is a critical aspect of dealing with positively skewed data. Various methods exist for outlier detection, including box plots, scatter plots, and statistical measures like the z-score. Depending on the context and nature of the data, different strategies may be employed for handling outliers, including removal, transformation, or winsorization.
FAQ Section: Answering Common Questions About Positive Skewness:
Q: What is the difference between positive and negative skewness?
A: Positive skewness (right skew) has a longer tail on the right, while negative skewness (left skew) has a longer tail on the left.
Q: How can I determine if my data is positively skewed?
A: Visual inspection of a histogram or box plot is a good starting point. Calculating the skewness coefficient provides a numerical measure. The relationship between the mean, median, and mode (Mean > Median > Mode) also indicates positive skewness.
Q: What statistical tests are appropriate for positively skewed data?
A: Non-parametric tests are generally preferred. If data transformation achieves normality, parametric tests can be used.
Q: What is the best way to handle outliers in positively skewed data?
A: The optimal approach depends on the context and potential causes of the outliers. Methods include removal, transformation, or winsorization. Careful consideration of the impact on the analysis is crucial.
Practical Tips: Maximizing the Understanding of Positive Skewness:
- Visualize your data: Always start with visual representations like histograms and box plots to get a sense of the distribution's shape.
- Calculate the skewness coefficient: Use statistical software to calculate the skewness coefficient to get a quantitative measure of skewness.
- Consider data transformations: If skewness is problematic, explore appropriate transformations to improve normality.
- Choose appropriate statistical tests: Select statistical tests that are robust to non-normality if necessary.
- Document your choices: Clearly document the methods used for handling skewness and outliers to ensure transparency and reproducibility.
Final Conclusion: Wrapping Up with Lasting Insights:
Positive skewness is a common feature in many datasets, and understanding its implications is vital for accurate data interpretation and effective decision-making. By employing appropriate techniques for visualizing, analyzing, and handling positively skewed data, researchers and analysts can avoid misleading conclusions and draw more accurate insights from their data. Ignoring positive skewness can lead to flawed analyses and ultimately, poor decisions. The principles outlined in this article provide a solid foundation for navigating this common statistical challenge.
Latest Posts
Latest Posts
-
Chemo Xrt Definition
Apr 02, 2025
-
Palliative Xrt Definition
Apr 02, 2025
-
Xrt Definition Medical Terms
Apr 02, 2025
-
Xenocurrency Meaning
Apr 02, 2025
-
Xenocurrency Adalah
Apr 02, 2025
Related Post
Thank you for visiting our website which covers about Positive Skewness Definition . We hope the information provided has been useful to you. Feel free to contact us if you have any questions or need further assistance. See you next time and don't miss to bookmark.