One Tailed Test Explained Definition And Example
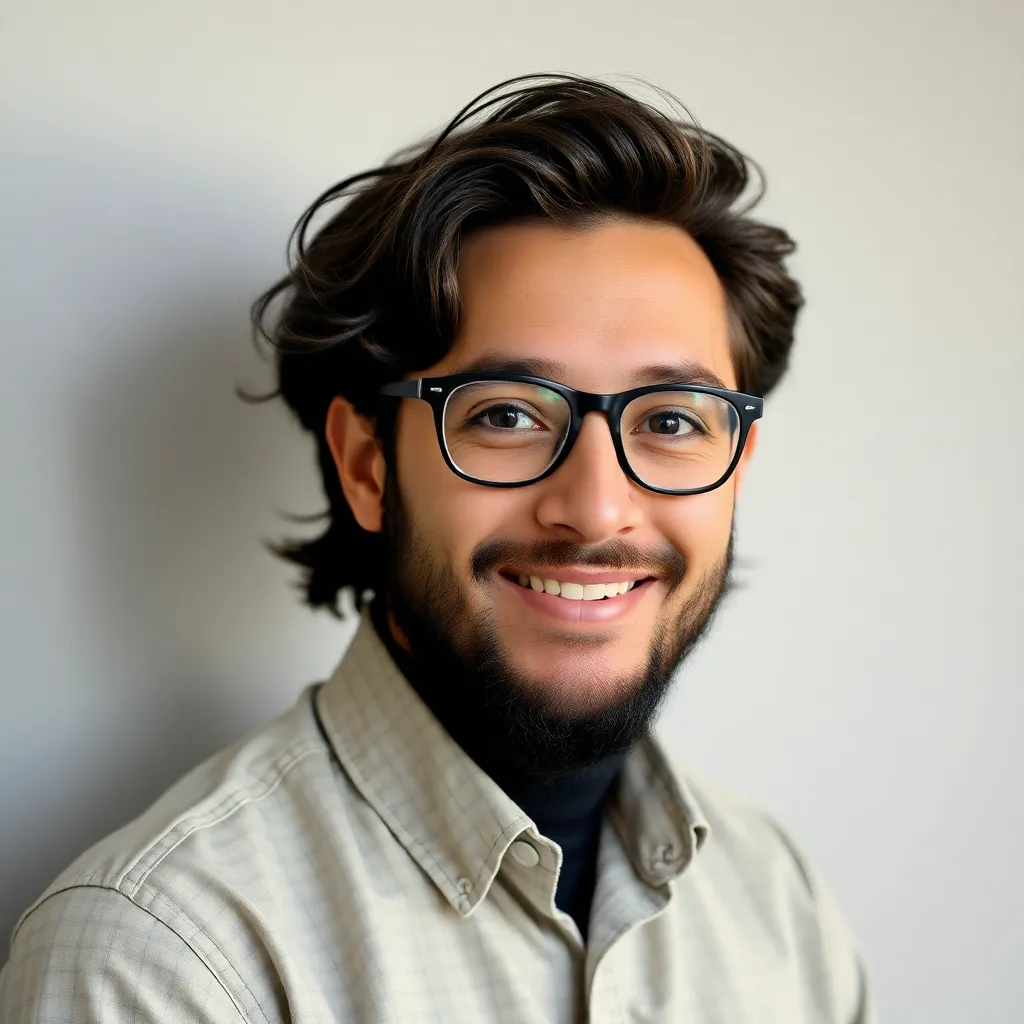
adminse
Mar 09, 2025 · 8 min read

Table of Contents
Understanding One-Tailed Tests: Definition, Examples, and Applications
What if your hypothesis only predicts a change in one direction? A one-tailed test provides the statistical power to examine such specific predictions.
Editor's Note: This article on one-tailed tests provides a comprehensive explanation of this statistical concept, including its definition, applications, and practical examples. It aims to equip readers with the knowledge to understand and apply one-tailed tests effectively in various contexts.
Why One-Tailed Tests Matter:
One-tailed tests, also known as directional tests, are a crucial statistical tool for analyzing data when researchers have a specific, directional hypothesis about the relationship between variables. Unlike two-tailed tests, which examine changes in both directions (positive and negative), one-tailed tests focus solely on a single direction of change. This focused approach can be more powerful when the researcher has strong prior evidence or theoretical justification for anticipating a specific effect. Understanding when to use a one-tailed test is essential for drawing accurate conclusions from statistical analyses, avoiding misleading results, and enhancing the efficiency of research. Misapplication can lead to overlooking potentially significant results or drawing incorrect inferences from the data.
Overview: What This Article Covers:
This article will provide a thorough understanding of one-tailed tests by exploring the following aspects:
- Definition and Core Concepts: A detailed explanation of one-tailed tests, their underlying principles, and how they differ from two-tailed tests.
- Applications Across Industries: Examples of how one-tailed tests are applied in various fields, such as medicine, engineering, and business.
- Challenges and Solutions: Potential pitfalls in using one-tailed tests and strategies to mitigate these challenges.
- The Relationship between Type I and Type II Errors and One-Tailed Tests: How the choice of a one-tailed test influences the probabilities of these errors.
- Step-by-Step Example using a t-test: A detailed walk-through of performing a one-tailed t-test.
- Comparison with Two-Tailed Tests: A clear comparison highlighting the differences and when each test is appropriate.
- Frequently Asked Questions: Addressing common questions and concerns regarding the use of one-tailed tests.
The Research and Effort Behind the Insights:
This article draws upon established statistical literature, including textbooks and peer-reviewed research papers, to ensure accuracy and credibility. The examples provided are illustrative of real-world applications, although specific datasets have been simplified for clarity. The aim is to provide readers with a clear and accessible understanding of one-tailed tests, empowering them to use this important statistical tool effectively.
Key Takeaways:
- Definition: A one-tailed test is a statistical test in which the critical region of a distribution is one-sided so that only deviations in a specific direction from a null hypothesis are considered statistically significant.
- Applications: One-tailed tests are used when researchers have a strong directional hypothesis, such as expecting an increase or decrease in a specific variable.
- Type I and Type II Errors: One-tailed tests alter the balance between the probability of these errors.
- Interpretation: The p-value from a one-tailed test is interpreted differently than that from a two-tailed test.
Smooth Transition to the Core Discussion:
Having established the importance of one-tailed tests, let’s delve into their core concepts and explore how they differ from their two-tailed counterparts.
Exploring the Key Aspects of One-Tailed Tests:
1. Definition and Core Concepts:
A one-tailed test is a statistical hypothesis test in which the critical area of a distribution is one-sided, meaning that it only considers deviations in one direction from the null hypothesis. This contrasts with a two-tailed test, which considers deviations in both directions. The choice between a one-tailed and two-tailed test depends entirely on the research question and the researcher's prior knowledge or hypothesis. If a researcher has a strong prior reason to believe that an effect will be in one specific direction (e.g., an increase, not a decrease), then a one-tailed test is appropriate.
2. Applications Across Industries:
One-tailed tests find applications in various fields:
- Medicine: Testing the effectiveness of a new drug in reducing blood pressure. The hypothesis would be that the drug reduces blood pressure, not that it changes it in either direction.
- Engineering: Assessing whether a new manufacturing process increases the strength of a material.
- Business: Determining if a new marketing campaign increases sales.
- Education: Evaluating whether a new teaching method improves student test scores.
3. Challenges and Solutions:
The primary challenge with one-tailed tests lies in the potential for overlooking significant results if the effect is in the opposite direction to that hypothesized. If the true effect is opposite to the direction specified in the one-tailed test, the result might not be deemed statistically significant even if it is practically meaningful. Careful consideration of the research question and justification for the directional hypothesis are crucial before employing a one-tailed test.
4. Impact on Innovation:
The use of one-tailed tests enables researchers to focus their resources and analyses on effects of interest, potentially leading to more efficient and targeted research. By reducing the degrees of freedom and focusing on a single direction, the statistical power to detect an effect in that direction increases. This is particularly important in situations with limited resources or when the cost of missing a significant effect in one direction is substantially higher than the cost of missing an effect in the other.
Exploring the Connection Between Type I and Type II Errors and One-Tailed Tests:
A Type I error (false positive) occurs when a researcher rejects the null hypothesis when it is actually true. A Type II error (false negative) occurs when a researcher fails to reject the null hypothesis when it is actually false. One-tailed tests affect the balance between these two types of errors. By focusing on one direction, a one-tailed test increases the power to detect an effect in that direction (reducing the probability of a Type II error), but simultaneously increases the probability of a Type I error in the opposite direction. This trade-off is crucial for consideration when selecting a one-tailed versus a two-tailed approach.
Step-by-Step Example using a t-test:
Let's say a researcher wants to test if a new fertilizer increases the yield of a particular crop. The null hypothesis (H0) is that the fertilizer has no effect (mean yield is the same). The alternative hypothesis (H1) is that the fertilizer increases the yield (mean yield is greater). This is a one-tailed test because we only care about an increase in yield.
-
State the hypotheses:
- H0: μ_fertilizer ≤ μ_control
- H1: μ_fertilizer > μ_control
-
Choose a significance level (alpha): Often set at 0.05.
-
Collect data: Measure the yield from plots treated with the fertilizer and control plots.
-
Perform a one-tailed t-test: This involves calculating the t-statistic and comparing it to the critical t-value from the t-distribution with the appropriate degrees of freedom and one-tailed alpha level. Statistical software packages readily perform these calculations.
-
Interpret the results: If the calculated t-statistic exceeds the critical t-value, the null hypothesis is rejected, indicating statistically significant evidence that the fertilizer increases yield.
Comparison with Two-Tailed Tests:
A two-tailed test considers deviations from the null hypothesis in both positive and negative directions. A one-tailed test is more powerful when the researcher has strong reason to believe the effect will only be in one direction. However, if the effect is in the unexpected direction, a one-tailed test will fail to detect it, while a two-tailed test might. The choice depends on the context and the cost of making each type of error.
Frequently Asked Questions:
- Q: When should I use a one-tailed test? A: Use a one-tailed test when you have a strong directional hypothesis supported by prior evidence or theory.
- Q: What are the limitations of one-tailed tests? A: The main limitation is the risk of missing a significant effect if it's in the opposite direction to your hypothesis.
- Q: How do I interpret the p-value from a one-tailed test? A: The p-value represents the probability of observing the obtained results (or more extreme results) if the null hypothesis were true, assuming the effect is in the direction specified.
- Q: Can I change from a two-tailed to a one-tailed test after seeing the data? A: No, this is considered a form of p-hacking and invalidates the results. The test should be chosen before collecting data.
Practical Tips: Maximizing the Benefits of One-Tailed Tests:
- Strong Justification: Ensure you have a strong theoretical or empirical basis for your directional hypothesis.
- Clear Hypotheses: Clearly define your null and alternative hypotheses before data collection.
- Appropriate Test: Select the correct statistical test based on your data and research question.
- Software Use: Utilize statistical software for accurate calculation and interpretation.
Final Conclusion: Wrapping Up with Lasting Insights:
One-tailed tests are valuable statistical tools when appropriately applied. They offer increased power to detect effects in a specified direction, making them efficient for focused research questions. However, the potential for missing significant effects in the opposite direction necessitates careful consideration of the research context and justification for the directional hypothesis. By understanding the strengths and limitations of one-tailed tests, researchers can enhance the rigor and efficiency of their analyses. Remember, the decision of whether to use a one-tailed or two-tailed test should be made before collecting the data to maintain the integrity of the statistical analysis.
Latest Posts
Latest Posts
-
Scarcity Principle Definition Importance And Example
Apr 29, 2025
-
Scalping Definition In Trading How Strategy Is Used And Example
Apr 29, 2025
-
Pension Funds Are Prohibited From Using Which Investment Strategy
Apr 29, 2025
-
How Do Pension Funds Invest In Infrastructure
Apr 29, 2025
-
Which Pension Funds Are At Risk
Apr 29, 2025
Related Post
Thank you for visiting our website which covers about One Tailed Test Explained Definition And Example . We hope the information provided has been useful to you. Feel free to contact us if you have any questions or need further assistance. See you next time and don't miss to bookmark.