Multiple Discriminant Analysis Mda Definition How Its Used
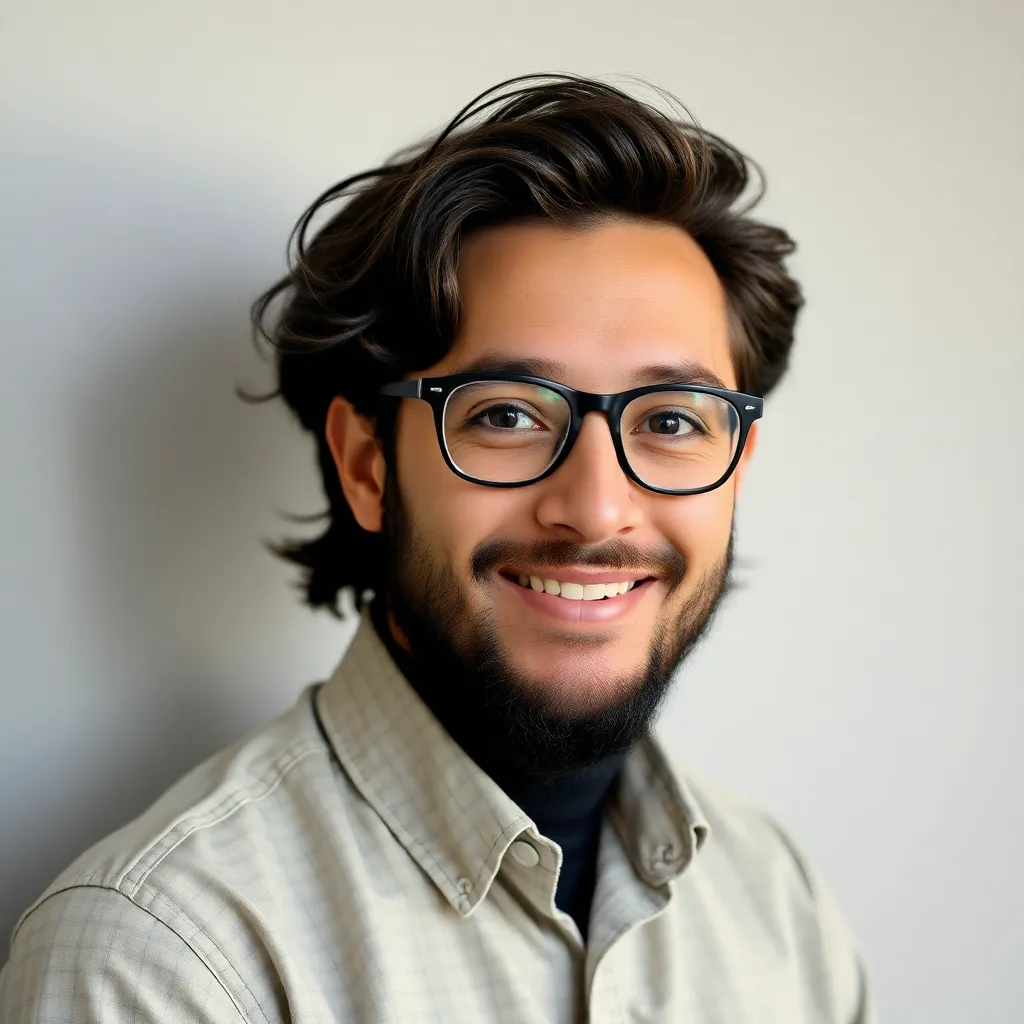
adminse
Apr 22, 2025 · 9 min read

Table of Contents
Unlocking the Power of Multiple Discriminant Analysis (MDA): Definition, Applications, and Insights
What if a single analytical technique could simultaneously classify observations into multiple groups and identify the variables driving those classifications? Multiple Discriminant Analysis (MDA) is that powerful tool, offering profound insights into complex datasets and informing crucial decisions across various fields.
Editor’s Note: This article on Multiple Discriminant Analysis (MDA) provides a comprehensive overview of its definition, applications, and interpretations. It’s designed to be accessible to a broad audience, offering both conceptual understanding and practical examples.
Why Multiple Discriminant Analysis Matters:
Multiple Discriminant Analysis (MDA) is a multivariate statistical technique used to model the relationship between a set of predictor (independent) variables and a categorical response variable with more than two groups (levels). Unlike techniques like linear discriminant analysis (LDA) which focuses on only two groups, MDA excels in situations where categorization involves three or more distinct classes. Its power lies in its ability to not only classify observations into their respective groups but also to identify which predictor variables contribute most significantly to these classifications. This makes MDA invaluable for understanding the underlying structure of data and making informed predictions. MDA finds applications in diverse fields, including marketing (customer segmentation), finance (credit risk assessment), medicine (disease diagnosis), and social sciences (behavioral analysis).
Overview: What This Article Covers:
This article provides a detailed exploration of MDA, covering its fundamental principles, assumptions, practical applications, and potential limitations. We'll delve into the mathematical underpinnings, illustrate its use with practical examples, and discuss its strengths and weaknesses compared to other classification methods. Readers will gain a robust understanding of how to apply and interpret MDA results, enabling them to leverage its power for data-driven decision-making.
The Research and Effort Behind the Insights:
This article synthesizes information from leading statistical textbooks, research articles, and online resources dedicated to multivariate analysis. The explanations are grounded in established statistical theory, and the examples are chosen to represent a range of real-world applications. The aim is to provide a clear, accurate, and accessible guide to understanding and utilizing MDA effectively.
Key Takeaways:
- Definition and Core Concepts: A precise definition of MDA and its core principles.
- Practical Applications: Real-world examples of MDA across various industries.
- Assumptions and Limitations: A critical assessment of the conditions under which MDA is most appropriate.
- Interpretation of Results: A step-by-step guide to understanding MDA output and drawing meaningful conclusions.
- Comparison with Other Techniques: How MDA compares to other multivariate techniques such as logistic regression and cluster analysis.
Smooth Transition to the Core Discussion:
Having established the importance and scope of MDA, let's now delve into its core aspects, exploring its mathematical foundation, practical applications, and interpretational nuances.
Exploring the Key Aspects of Multiple Discriminant Analysis:
1. Definition and Core Concepts:
MDA aims to find linear combinations of predictor variables that best separate the groups defined by the categorical response variable. These linear combinations are called discriminant functions. Each discriminant function maximizes the variance between groups while minimizing the variance within groups. The number of discriminant functions is typically limited to the minimum of (k-1) and p, where k is the number of groups and p is the number of predictor variables. This is because additional functions will not contribute significantly to separating the groups. Each function is uncorrelated with the others, providing a set of orthogonal dimensions for separating the groups.
2. Applications Across Industries:
The versatility of MDA makes it applicable in a broad range of fields:
- Marketing: Segmenting customers based on demographics, purchasing behavior, and psychographics to tailor marketing campaigns.
- Finance: Predicting credit risk by classifying borrowers into different risk categories based on financial indicators.
- Medicine: Diagnosing diseases by differentiating patients into different disease groups based on clinical variables.
- Social Sciences: Analyzing the factors that influence voting patterns or consumer preferences.
- Environmental Science: Classifying different environmental zones based on various ecological factors.
3. Assumptions and Limitations:
MDA relies on several key assumptions:
- Multivariate Normality: The predictor variables should follow a multivariate normal distribution within each group. While minor deviations may not severely impact the results, substantial departures can lead to inaccurate conclusions.
- Homogeneity of Variance-Covariance Matrices: The variance-covariance matrix of the predictor variables should be the same across all groups. Violation of this assumption can reduce the accuracy of classification.
- Linearity: The relationship between the predictor variables and the group membership should be linear. Nonlinear relationships may require transformations of the predictor variables or the use of alternative nonlinear methods.
- Multicollinearity: High correlation between predictor variables can negatively affect the stability and interpretability of the discriminant functions. Techniques like Principal Component Analysis (PCA) can be used to address this issue.
4. Interpretation of Results:
The output of MDA typically includes:
- Discriminant functions: Linear combinations of predictor variables that maximize group separation.
- Canonical correlation: A measure of the association between the discriminant functions and the group membership.
- Eigenvalues: Measures of the variance explained by each discriminant function.
- Classification functions: Used to predict group membership for new observations.
- Classification accuracy: The percentage of correctly classified observations in the validation sample.
5. Comparison with Other Techniques:
MDA shares similarities with other classification techniques but also possesses unique strengths:
- Logistic Regression: While logistic regression can handle multiple groups, it models the probability of belonging to each group separately. MDA provides a more holistic view by identifying linear combinations of predictors that best separate all groups simultaneously.
- Cluster Analysis: Cluster analysis groups observations based on similarity, without explicitly defining predefined groups. MDA requires pre-defined groups and aims to find the variables that best distinguish between them.
Exploring the Connection Between Data Preprocessing and MDA:
Data preprocessing plays a crucial role in the success of MDA. Understanding how preprocessing techniques influence the results is vital for accurate and reliable analysis.
Roles and Real-World Examples:
-
Standardization: Standardizing predictor variables (centering and scaling) is crucial because variables with larger scales can disproportionately influence the discriminant functions. This ensures that all variables contribute equally to the analysis, regardless of their units of measurement. For example, in a study analyzing customer segmentation based on income and age, standardizing both variables prevents income from dominating the analysis simply because it's expressed in larger numerical values.
-
Outlier Detection and Handling: Outliers can significantly distort the results of MDA. Robust methods for outlier detection and handling, such as using trimmed means or Winsorizing, should be employed. For instance, in a medical study using MDA to classify patients based on various biomarkers, outliers representing unusual biological responses should be carefully examined and treated appropriately to avoid misinterpreting the discriminant functions.
-
Missing Data Imputation: Dealing with missing data is essential. Various imputation techniques, such as mean imputation, k-nearest neighbors imputation, or multiple imputation, can be used. In a marketing study classifying customer segments based on survey responses, missing data imputation ensures that the analysis utilizes as much available information as possible.
Risks and Mitigations:
-
Violation of Assumptions: Failure to meet the assumptions of multivariate normality and homogeneity of variance-covariance matrices can lead to biased results. Transformations of the data, use of robust methods, or alternative techniques might be necessary.
-
Overfitting: With a large number of predictor variables relative to the sample size, the model may overfit the training data, leading to poor generalization to new data. Techniques like cross-validation or regularization can mitigate this risk.
-
Interpretability Issues: Interpreting the discriminant functions can be challenging, particularly with a large number of variables. Techniques like variable importance plots or stepwise selection can improve interpretability.
Impact and Implications:
Effective data preprocessing ensures that the MDA results are reliable, accurate, and generalizable. This impacts the validity of conclusions drawn from the analysis, influencing decisions made in various applications like marketing campaigns, financial risk assessments, or medical diagnoses. Careful attention to preprocessing safeguards the integrity of the MDA results and increases their impact.
Conclusion: Reinforcing the Connection:
The interplay between data preprocessing and MDA underscores the importance of data quality and proper methodological choices. By addressing challenges and leveraging opportunities, researchers and practitioners can harness the full power of MDA to obtain valid and insightful results.
Further Analysis: Examining Dimensionality Reduction Techniques in MDA:
Dimensionality reduction techniques, like Principal Component Analysis (PCA), can be employed before applying MDA to address multicollinearity and improve the interpretability of the results. PCA transforms the original predictor variables into a smaller set of uncorrelated principal components, retaining most of the variance in the data. This can simplify the MDA model, making it easier to interpret the discriminant functions and improve its computational efficiency. In applications with high-dimensional data, such as genomic studies, PCA is often used to reduce the number of variables before applying MDA for classification.
FAQ Section: Answering Common Questions About MDA:
Q: What is the difference between MDA and LDA?
A: MDA extends LDA to handle more than two groups in the categorical response variable. LDA is a special case of MDA limited to only two groups.
Q: How do I interpret the discriminant functions?
A: Discriminant functions are linear combinations of predictor variables. The coefficients associated with each variable indicate its contribution to the separation of groups. Larger absolute coefficients indicate stronger contributions.
Q: What is the significance of canonical correlations?
A: Canonical correlations measure the correlation between the discriminant functions and the group membership. Higher values indicate stronger association and better group separation.
Q: What should I do if my data violates the assumptions of MDA?
A: Consider data transformations, robust methods, or alternative techniques like logistic regression or classification trees.
Q: How can I assess the accuracy of my MDA model?
A: Use cross-validation techniques to evaluate the model's performance on unseen data.
Practical Tips: Maximizing the Benefits of MDA:
- Start with Exploratory Data Analysis: Examine your data thoroughly before applying MDA to identify outliers, missing data, and potential violations of assumptions.
- Use appropriate preprocessing techniques: Standardize your data and handle missing data appropriately.
- Consider dimensionality reduction: Use PCA or other dimensionality reduction techniques to simplify the analysis and improve interpretability.
- Validate your model: Use cross-validation or other validation methods to assess the model's generalization ability.
- Interpret the results cautiously: Be mindful of the assumptions of MDA and consider potential limitations when interpreting the results.
Final Conclusion: Wrapping Up with Lasting Insights:
Multiple Discriminant Analysis is a valuable tool for classifying observations into multiple groups and identifying the variables that drive those classifications. By understanding its principles, assumptions, and limitations, researchers and practitioners can leverage its power to gain insights from complex datasets and make informed decisions in a variety of fields. While it requires careful consideration of data preprocessing and assumption checks, the potential for significant insights makes MDA a powerful addition to any data analyst's toolkit. Its ability to simultaneously classify and identify key differentiating factors makes it an invaluable asset for navigating the complexities of multivariate data.
Latest Posts
Latest Posts
-
National Issuers Definition
Apr 22, 2025
-
National Diamond Definition
Apr 22, 2025
-
National Credit Union Administration Ncua Definition
Apr 22, 2025
-
National Bank Surveillance System Nbss Definition
Apr 22, 2025
-
National Association Of State Boards Of Accountancy Nasba Definition
Apr 22, 2025
Related Post
Thank you for visiting our website which covers about Multiple Discriminant Analysis Mda Definition How Its Used . We hope the information provided has been useful to you. Feel free to contact us if you have any questions or need further assistance. See you next time and don't miss to bookmark.