Moodys Analytics Definition
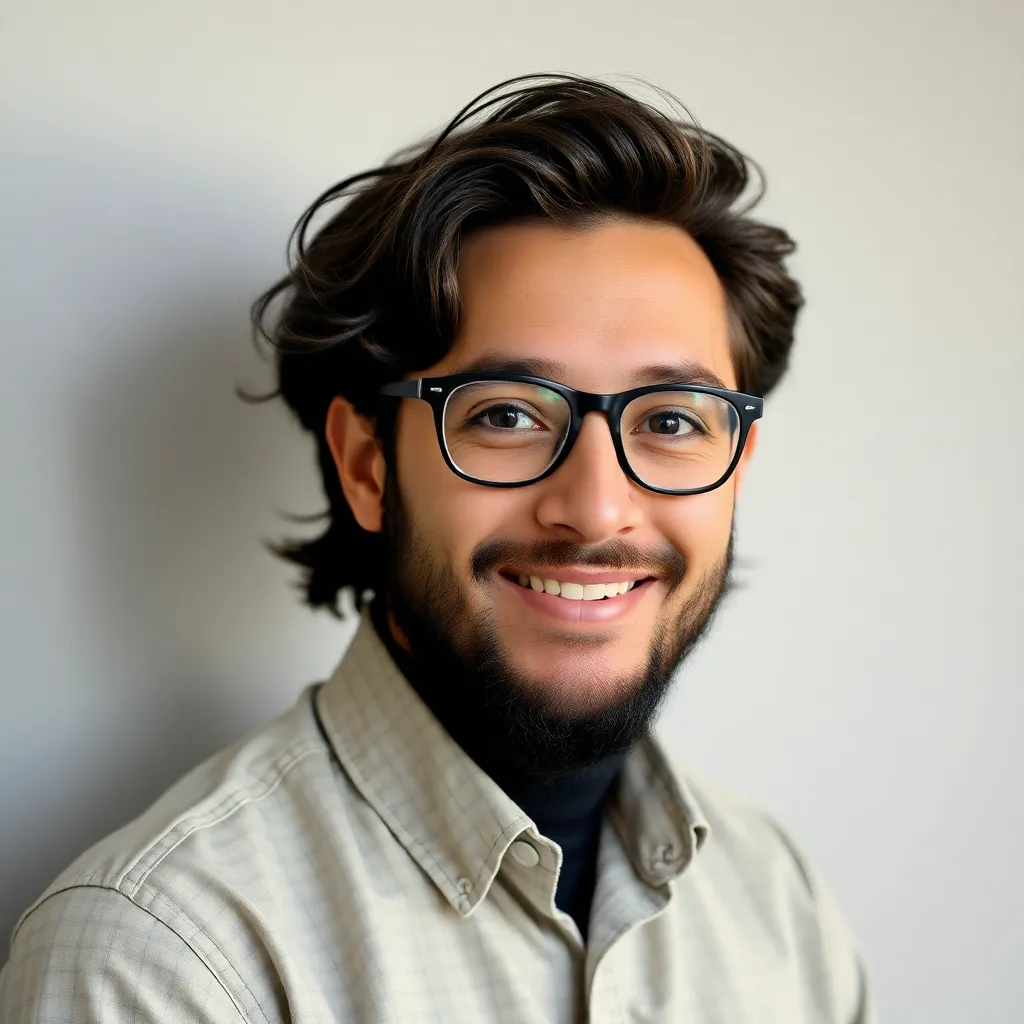
adminse
Apr 22, 2025 · 8 min read

Table of Contents
Unlocking the Power of Predictive Analytics: A Deep Dive into Moody's Analytics Definition
What if the future of financial decision-making hinges on understanding Moody's Analytics' definition of predictive analytics? This powerful methodology is transforming how organizations assess risk, optimize strategies, and navigate the complexities of the global financial landscape.
Editor’s Note: This article on Moody's Analytics' definition of predictive analytics provides a comprehensive overview of this crucial field, drawing upon Moody's publicly available resources and general industry understanding. It aims to equip readers with a clear understanding of the concepts, applications, and implications of predictive analytics within the context of Moody's expertise.
Why Moody's Analytics' Approach to Predictive Analytics Matters:
Moody's Analytics, a globally recognized leader in credit rating and financial analysis, utilizes predictive analytics to provide crucial insights into financial markets, credit risk, and economic trends. Their approach isn't merely about forecasting; it's about integrating sophisticated statistical modeling, machine learning algorithms, and vast datasets to generate actionable intelligence. This matters because accurate prediction significantly reduces uncertainties, enabling better risk management and informed strategic planning for businesses, investors, and policymakers alike. The implications span various sectors, from banking and insurance to investment management and government regulation.
Overview: What This Article Covers:
This article will delve into the core principles of predictive analytics as defined and applied by Moody's Analytics. We will explore its foundational elements, its practical applications across various industries, common challenges, future implications, and the crucial role of data quality and model validation. We'll also examine specific areas where Moody's leverages these techniques and how their definition differs from a more general understanding of the field.
The Research and Effort Behind the Insights:
This article draws upon publicly available information from Moody's Analytics website, press releases, industry reports, and academic research on predictive modeling techniques. The analysis focuses on extracting the underlying principles and methodologies that underpin Moody's application of predictive analytics, providing a comprehensive and accurate representation of their approach.
Key Takeaways:
- Definition and Core Concepts: A precise understanding of Moody's implicit definition of predictive analytics, emphasizing its focus on risk assessment and financial forecasting.
- Practical Applications: Examples of how Moody's employs predictive analytics across different financial domains, including credit risk assessment, portfolio management, and economic forecasting.
- Data Sources and Methodology: An examination of the types of data utilized by Moody's and the statistical methods employed to build robust predictive models.
- Challenges and Limitations: Addressing potential challenges like data bias, model limitations, and the ethical considerations of predictive modeling in finance.
- Future Implications: Exploring the future potential of predictive analytics within Moody's Analytics and its impact on the broader financial industry.
Smooth Transition to the Core Discussion:
Having established the importance of understanding Moody's approach, let's now delve into the key aspects of their predictive analytics methodology, analyzing its components and applications in detail.
Exploring the Key Aspects of Moody's Analytics' Predictive Analytics:
While Moody's doesn't explicitly lay out a singular, concise definition of "predictive analytics," their extensive use of the methodology reveals a distinct approach. Their framework centers around the following key aspects:
1. Data Integration and Management: Moody's possesses an extensive repository of financial data, encompassing macroeconomic indicators, credit ratings, market data, and company financials. This data is meticulously cleaned, validated, and integrated to create comprehensive datasets suitable for building predictive models. The emphasis is on both breadth (covering various data sources) and depth (incorporating historical trends and granular details).
2. Advanced Statistical Modeling and Machine Learning: Moody's utilizes a range of statistical techniques, including regression analysis, time series modeling, and machine learning algorithms (like neural networks and random forests) to build predictive models. The choice of methodology depends on the specific application and the characteristics of the data. The focus is on building models that are not only accurate but also robust and interpretable, allowing for a clear understanding of the underlying drivers of predictions.
3. Model Validation and Risk Management: A crucial element of Moody's approach is rigorous model validation. This involves testing the model's performance on unseen data, assessing its sensitivity to changes in input variables, and quantifying the uncertainty associated with its predictions. Moody's employs sophisticated risk management techniques to manage the inherent uncertainties associated with any predictive model.
4. Application Across Financial Domains: Moody's applies predictive analytics across numerous financial areas, including:
* **Credit Risk Assessment:** Predicting the probability of default for borrowers, informing credit rating decisions and loan pricing.
* **Portfolio Management:** Optimizing investment portfolios by predicting asset returns and assessing risk exposures.
* **Economic Forecasting:** Predicting macroeconomic indicators such as GDP growth, inflation, and interest rates.
* **Financial Stress Testing:** Assessing the resilience of financial institutions under various stress scenarios.
Closing Insights: Summarizing the Core Discussion:
Moody's Analytics’ implicit definition of predictive analytics is characterized by its rigorous data-driven approach, sophisticated modeling techniques, and emphasis on model validation and risk management. This comprehensive framework allows them to generate actionable insights across various financial domains, enhancing risk management, optimizing investment strategies, and informing critical economic decisions.
Exploring the Connection Between Data Quality and Moody's Analytics' Predictive Analytics:
The relationship between data quality and Moody's predictive analytics is paramount. The accuracy and reliability of any predictive model depend fundamentally on the quality of the input data. Moody's invests heavily in data sourcing, cleaning, and validation to ensure the integrity of its datasets.
Key Factors to Consider:
-
Roles and Real-World Examples: Moody's stringent data quality checks, including data cleansing, outlier detection, and consistency checks, directly impact the accuracy of credit risk models, for instance. Inaccurate data can lead to misclassifications of borrowers, potentially affecting lending decisions and overall risk assessment.
-
Risks and Mitigations: Data biases, missing data, and inconsistent data formats pose significant risks. Moody's mitigates these risks through robust data preprocessing techniques, imputation methods, and careful selection of data sources.
-
Impact and Implications: High-quality data translates to more accurate predictions, improved decision-making, and a more robust risk management framework. Conversely, poor data quality can lead to inaccurate forecasts, flawed strategies, and increased financial losses.
Conclusion: Reinforcing the Connection:
The connection between data quality and Moody's predictive analytics cannot be overstated. The accuracy, robustness, and reliability of their predictive models are directly contingent on the quality, consistency, and integrity of the underlying data. Moody's commitment to high-quality data forms a cornerstone of their success in applying predictive analytics across the financial industry.
Further Analysis: Examining Data Sources in Greater Detail:
Moody's data sources are diverse and encompass both proprietary and publicly available information. Proprietary data includes their extensive credit rating databases, internal research reports, and market data feeds. Publicly available data sources include macroeconomic statistics from government agencies, financial statements of publicly listed companies, and market data from exchanges. The integration of these diverse data sources is crucial for creating a comprehensive and nuanced view of the financial landscape. This integrated approach enables Moody's to build predictive models that capture both micro-level (individual borrower or company) and macro-level (economic trends) factors.
FAQ Section: Answering Common Questions About Moody's Analytics' Predictive Analytics:
Q: What types of predictive models does Moody's Analytics use?
A: Moody's employs a diverse range of models, including linear regression, logistic regression, time series analysis, and various machine learning algorithms like neural networks and random forests. The choice of model depends on the specific application and the nature of the data.
Q: How does Moody's ensure the accuracy of its predictive models?
A: Moody's employs rigorous model validation techniques, including backtesting, stress testing, and out-of-sample testing. They also monitor the performance of their models over time and make adjustments as needed.
Q: What are the limitations of Moody's predictive analytics?
A: Like any predictive model, Moody's models are subject to limitations. Unforeseen events, changes in market dynamics, and inherent uncertainties in data can all affect the accuracy of predictions. Moreover, model biases can exist if the training data does not fully represent the population of interest.
Q: How does Moody's address ethical concerns related to its use of predictive analytics?
A: Moody's adheres to strict ethical guidelines and regulatory requirements in the development and application of its predictive analytics models. Transparency, fairness, and accountability are prioritized to ensure the responsible use of this powerful technology.
Practical Tips: Maximizing the Benefits of Understanding Moody's Predictive Analytics:
-
Understand the Fundamentals: Begin by grasping the core principles of predictive analytics and how they relate to financial risk management.
-
Explore Moody's Resources: Familiarize yourself with publicly available information on Moody's Analytics, focusing on their methodologies and applications of predictive analytics.
-
Consider the Limitations: Recognize the inherent limitations of any predictive model and the importance of cautious interpretation of results.
Final Conclusion: Wrapping Up with Lasting Insights:
Moody's Analytics’ application of predictive analytics represents a significant advancement in financial risk management and decision-making. By integrating vast datasets, employing sophisticated modeling techniques, and adhering to stringent validation processes, Moody's generates valuable insights that inform credit ratings, investment strategies, and economic forecasts. Understanding Moody's approach offers valuable lessons for businesses and individuals seeking to leverage the power of data-driven decision-making in the dynamic world of finance. While challenges and limitations exist, the ongoing development and refinement of predictive analytics underpins the future of informed financial decision-making.
Latest Posts
Latest Posts
-
Msci Emu Index Definition
Apr 22, 2025
-
What Is Accumulated Deficit On A Balance Sheet
Apr 22, 2025
-
How To Find Total Liabilities On Balance Sheet
Apr 22, 2025
-
Msci All Country World Index Acwi Definition And Countries
Apr 22, 2025
-
Msci Bric Index Definition
Apr 22, 2025
Related Post
Thank you for visiting our website which covers about Moodys Analytics Definition . We hope the information provided has been useful to you. Feel free to contact us if you have any questions or need further assistance. See you next time and don't miss to bookmark.