Measure Of Skewness Definition
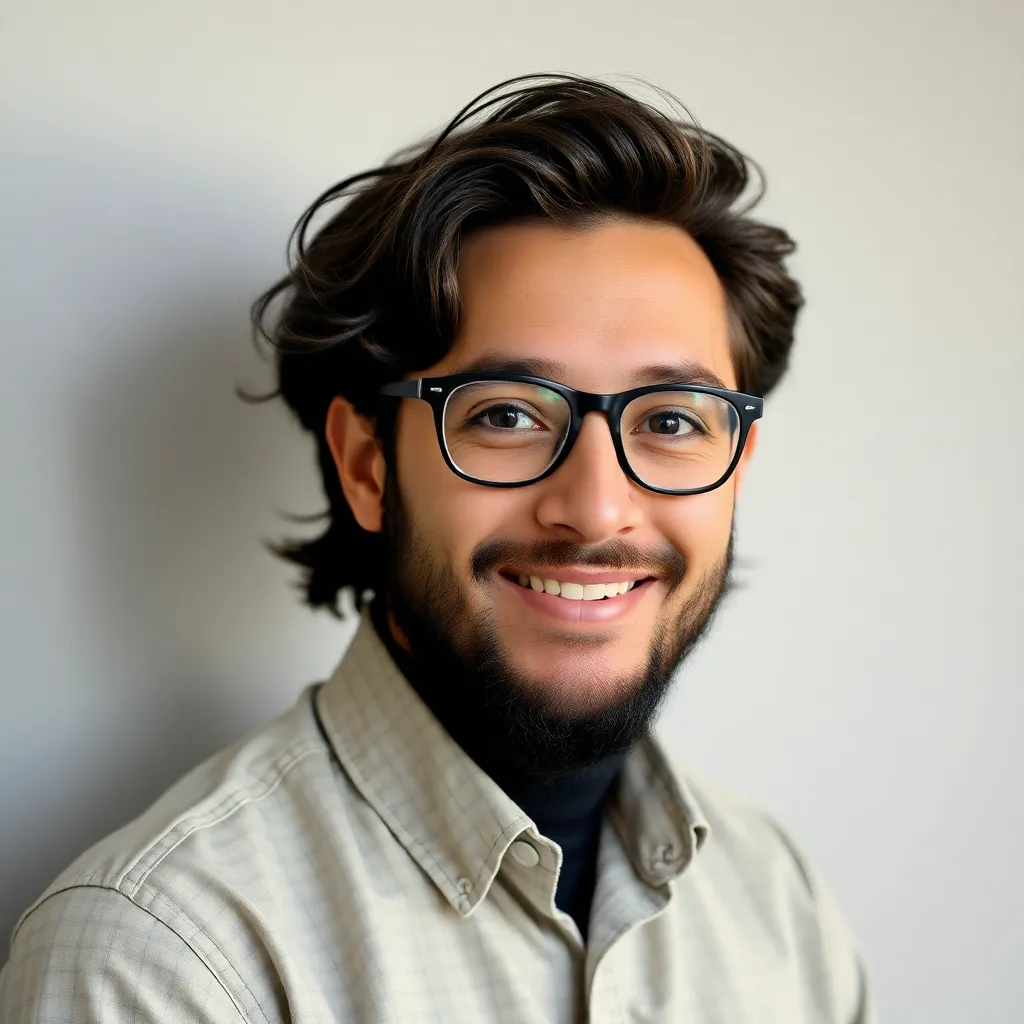
adminse
Mar 28, 2025 · 8 min read

Table of Contents
Unveiling the Secrets of Skewness: A Comprehensive Guide to its Measurement
What if the future of data analysis hinges on a deeper understanding of skewness? This fundamental statistical concept is crucial for interpreting data accurately and making informed decisions across diverse fields.
Editor’s Note: This article on the measurement of skewness provides a comprehensive overview of this crucial statistical concept. It explores various methods for quantifying skewness, their applications, and limitations, ensuring readers gain a thorough understanding of the topic.
Why Skewness Matters: Relevance, Practical Applications, and Industry Significance
Skewness, a measure of the asymmetry of a probability distribution, is far from a mere statistical curiosity. It plays a vital role in numerous fields, offering invaluable insights into the nature of data. Understanding skewness is crucial for:
- Accurate Data Interpretation: Symmetrical distributions (like the normal distribution) are easy to interpret; however, skewed distributions require careful consideration of their asymmetry to avoid misleading conclusions.
- Risk Assessment: In finance, skewed distributions are common (e.g., investment returns). Recognizing skewness helps assess risk more accurately, particularly the potential for extreme events (tail risk).
- Predictive Modeling: Many statistical models assume normality. Highly skewed data can violate these assumptions, leading to inaccurate predictions. Understanding skewness allows for data transformations or the selection of appropriate, robust models.
- Business Decision-Making: Skewed data frequently appears in business contexts (e.g., customer spending, sales figures). Understanding skewness can improve strategic planning, resource allocation, and marketing efforts.
- Scientific Research: Across scientific disciplines, recognizing skewness is crucial for valid interpretations of experimental results and developing accurate models of natural phenomena.
Overview: What This Article Covers
This article provides a detailed exploration of skewness measurement. We'll examine various methods, including Pearson's moment coefficient of skewness, the quartile coefficient of skewness, and the Bowley's coefficient of skewness. We will compare their strengths and weaknesses, discuss their applications, and illustrate their usage with practical examples. Further, the article delves into the implications of different types of skewness (positive and negative) and discusses how to handle skewed data in statistical analysis.
The Research and Effort Behind the Insights
This article synthesizes information from numerous sources, including leading statistical textbooks, peer-reviewed research papers, and reputable online resources. The explanations are grounded in established statistical principles, and the examples are designed to illustrate concepts clearly and practically.
Key Takeaways:
- Definition of Skewness: A clear understanding of what skewness represents and its significance in data analysis.
- Methods of Measuring Skewness: Detailed explanations of Pearson's moment coefficient, quartile coefficient, and Bowley's coefficient, including their formulas and calculations.
- Interpreting Skewness Measures: Guidance on how to interpret the numerical values obtained from skewness calculations.
- Types of Skewness: Distinguishing between positive and negative skewness and their implications.
- Handling Skewed Data: Strategies for addressing skewed data in statistical analysis.
Smooth Transition to the Core Discussion:
With a firm grasp on the importance of skewness, let's delve into the specifics of its measurement. We will begin by defining skewness and then explore the most common methods used to quantify this important statistical property.
Exploring the Key Aspects of Skewness Measurement
1. Definition and Core Concepts:
Skewness quantifies the asymmetry of a probability distribution. A symmetrical distribution, such as the normal distribution, has a skewness of zero. In a positively skewed distribution, the tail extends to the right (higher values), indicating a concentration of data points at lower values and a few extreme high values. Conversely, a negatively skewed distribution has a tail extending to the left (lower values), suggesting a concentration of data points at higher values with a few extreme low values.
2. Pearson's Moment Coefficient of Skewness:
This is the most widely used method for measuring skewness. It's based on the relationship between the mean, median, and standard deviation. The formula is:
Skewness = 3 * (Mean - Median) / Standard Deviation
A positive value indicates positive skewness, a negative value indicates negative skewness, and a value close to zero suggests a relatively symmetrical distribution. This method is sensitive to outliers, which can significantly influence the mean and standard deviation.
3. Quartile Coefficient of Skewness:
This method uses quartiles (Q1, Q2, Q3) to measure skewness, making it less sensitive to extreme values than Pearson's moment coefficient. The formula is:
Skewness = (Q3 - 2Q2 + Q1) / (Q3 - Q1)
Similar to Pearson's method, a positive value indicates positive skewness, a negative value indicates negative skewness, and a value near zero suggests symmetry.
4. Bowley's Coefficient of Skewness:
This is another quartile-based measure of skewness, defined as:
Skewness = (Q3 + Q1 - 2Q2) / (Q3 - Q1)
Again, a positive value indicates positive skewness, a negative value indicates negative skewness, and a value around zero suggests symmetry. This measure is robust to outliers and provides a measure of skewness that is less affected by extreme values.
5. Applications Across Industries:
The applications of skewness measurement are vast and span diverse fields:
- Finance: Assessing investment risk, modeling asset returns, and managing portfolio risk.
- Healthcare: Analyzing health outcomes, identifying risk factors for diseases, and understanding the distribution of patient characteristics.
- Engineering: Analyzing material properties, characterizing manufacturing defects, and assessing system reliability.
- Marketing: Understanding customer behavior, segmenting markets, and improving marketing strategies.
6. Challenges and Solutions:
While several methods exist for measuring skewness, each has limitations:
- Sensitivity to Outliers: Pearson's moment coefficient is highly sensitive to outliers. Robust alternatives like the quartile-based methods are preferable when dealing with data containing extreme values.
- Sample Size: Skewness estimates can be unreliable with small sample sizes. Larger samples generally provide more accurate estimations.
- Data Transformation: Highly skewed data may require transformation (e.g., logarithmic transformation) to stabilize variance and improve the performance of statistical models that assume normality.
7. Impact on Innovation:
Understanding skewness leads to innovations in various fields. For example, the development of robust statistical methods for handling skewed data has enhanced the accuracy of predictive models and improved decision-making in many applications.
Closing Insights: Summarizing the Core Discussion
The measurement of skewness is a fundamental aspect of statistical analysis. Understanding the different methods, their strengths and weaknesses, and their applications allows for more accurate data interpretation and informed decision-making across various domains. Choosing the appropriate method depends on the specific data characteristics and the research goals.
Exploring the Connection Between Data Transformation and Skewness Measurement
The relationship between data transformation and skewness measurement is crucial. Highly skewed data often violates the assumptions of many statistical models that assume normality. Data transformations aim to reduce skewness and make the data more closely resemble a normal distribution.
Key Factors to Consider:
- Roles and Real-World Examples: Logarithmic transformations are commonly used to reduce positive skewness in data with positive values, while Box-Cox transformations offer more flexibility. For example, income data, often positively skewed, can benefit from a logarithmic transformation.
- Risks and Mitigations: Incorrectly applying transformations can introduce bias or distort the underlying data structure. Careful consideration of the data and the transformation's impact is necessary.
- Impact and Implications: Transforming data can improve the accuracy of statistical inferences and model predictions, but it also changes the scale of the data, requiring careful interpretation of the results.
Conclusion: Reinforcing the Connection
Data transformation plays a critical role in managing skewness. By carefully selecting and applying appropriate transformations, researchers can improve the accuracy and reliability of their analyses, leading to more informed conclusions and better decision-making.
Further Analysis: Examining Data Transformation in Greater Detail
Several transformations exist, each with specific properties and applications. The choice of transformation depends on the type and degree of skewness, the presence of outliers, and the nature of the data. Understanding these nuances is critical for effective data analysis.
FAQ Section: Answering Common Questions About Skewness Measurement
- What is skewness? Skewness is a measure of the asymmetry of a probability distribution. It indicates whether the data is concentrated more on one side of the mean than the other.
- How is skewness measured? Several methods exist, including Pearson's moment coefficient, quartile coefficient, and Bowley's coefficient. The choice of method depends on the data characteristics and the research goals.
- What does positive skewness mean? Positive skewness indicates that the tail of the distribution extends to the right (higher values), with a concentration of data points at lower values and a few extreme high values.
- What does negative skewness mean? Negative skewness indicates that the tail of the distribution extends to the left (lower values), with a concentration of data points at higher values and a few extreme low values.
- How do I handle skewed data? Methods include data transformation (e.g., logarithmic transformation), using robust statistical methods, or employing non-parametric statistical tests.
Practical Tips: Maximizing the Benefits of Skewness Analysis
- Visualize your data: Histograms and box plots can provide a visual indication of skewness before performing any calculations.
- Choose an appropriate method: Select a skewness measure that is suitable for your data (e.g., robust methods for data with outliers).
- Interpret the results carefully: Consider the context of the data and the limitations of the chosen method when interpreting skewness values.
- Consider data transformation: If the data is highly skewed, consider applying a suitable transformation to improve the performance of statistical models.
Final Conclusion: Wrapping Up with Lasting Insights
Understanding and measuring skewness is an essential skill for anyone working with data. By grasping the concepts, methods, and implications discussed in this article, individuals can enhance their ability to interpret data accurately, build robust models, and make more informed decisions. The careful consideration of skewness is critical for drawing valid conclusions from data analysis and contributes significantly to responsible and effective decision-making across diverse fields.
Latest Posts
Latest Posts
-
International Fisher Effect Ife Definition Example Formula
Apr 24, 2025
-
International Finance Corporation Ifc Definition And Example
Apr 24, 2025
-
International Etf Definition
Apr 24, 2025
-
International Depository Receipt Idr Definition And Uses
Apr 24, 2025
-
International Commodities Clearing House Icch Definition
Apr 24, 2025
Related Post
Thank you for visiting our website which covers about Measure Of Skewness Definition . We hope the information provided has been useful to you. Feel free to contact us if you have any questions or need further assistance. See you next time and don't miss to bookmark.