Log Normal Distribution Definition Uses And How To Calculate
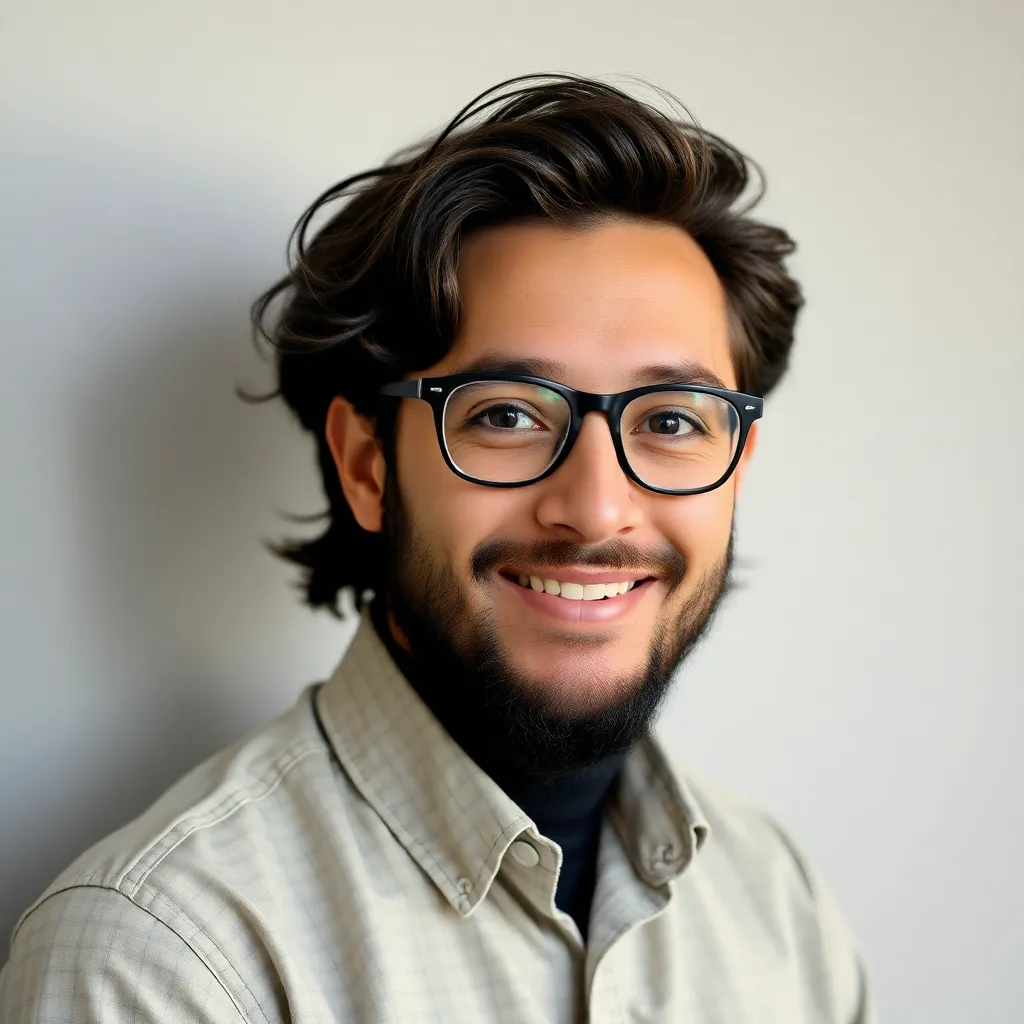
adminse
Apr 20, 2025 · 8 min read

Table of Contents
Unveiling the Log-Normal Distribution: Definition, Uses, and Calculation
What if understanding the log-normal distribution unlocks hidden patterns in seemingly disparate datasets? This powerful statistical tool offers invaluable insights across diverse fields, from finance to engineering.
Editor’s Note: This comprehensive article on the log-normal distribution provides a detailed explanation of its definition, applications, and calculation methods. It's designed to equip readers with a practical understanding of this vital statistical concept. Updated [Date of Publication].
Why the Log-Normal Distribution Matters: Relevance, Practical Applications, and Industry Significance
The log-normal distribution, while perhaps less familiar than the normal (Gaussian) distribution, holds immense practical significance across numerous disciplines. It arises naturally when the logarithm of a random variable follows a normal distribution. This seemingly simple characteristic has profound implications, making it invaluable for modeling phenomena exhibiting positive skewness and multiplicative effects. Its applications range from financial modeling, predicting the size of particles in a suspension, and analyzing reliability data to understanding the spread of infectious diseases and even the growth of populations. Understanding this distribution allows for accurate risk assessment, improved forecasting, and more effective decision-making in various contexts.
Overview: What This Article Covers
This article provides a thorough exploration of the log-normal distribution. We will delve into its precise definition, examine its key properties, explore its diverse applications across various fields, and provide step-by-step guidance on calculating key parameters and probabilities. We will also look at how to fit a log-normal distribution to real-world data. Readers will gain a comprehensive understanding of this powerful statistical tool and its practical applications.
The Research and Effort Behind the Insights
This article is based on extensive research, drawing upon established statistical literature, peer-reviewed publications, and practical applications across numerous fields. The explanations provided are supported by mathematical derivations and illustrative examples to ensure clarity and accuracy. The goal is to provide readers with a robust and reliable understanding of the log-normal distribution.
Key Takeaways:
- Definition and Core Concepts: A clear definition of the log-normal distribution and its relationship to the normal distribution.
- Practical Applications: Real-world examples showcasing the log-normal distribution's diverse uses across various industries.
- Calculation Methods: Step-by-step instructions on calculating key parameters like the mean, median, and standard deviation, as well as probabilities.
- Data Fitting and Interpretation: Guidance on fitting a log-normal distribution to a dataset and interpreting the results.
Smooth Transition to the Core Discussion
Having established the importance and scope of our discussion, let's now delve into the core aspects of the log-normal distribution, starting with its precise definition and fundamental characteristics.
Exploring the Key Aspects of the Log-Normal Distribution
1. Definition and Core Concepts:
A random variable X is said to follow a log-normal distribution if its natural logarithm, ln(X), follows a normal distribution. In other words, if Y = ln(X) ~ N(μ, σ²), where μ is the mean and σ² is the variance of the underlying normal distribution, then X follows a log-normal distribution, denoted as X ~ LogN(μ, σ²). The parameters μ and σ² are not the mean and variance of X itself, but rather the mean and variance of the logarithm of X. This subtle but crucial distinction is fundamental to understanding the log-normal distribution.
2. Applications Across Industries:
The log-normal distribution's versatility stems from its ability to model positive, right-skewed data. This makes it particularly useful in:
- Finance: Modeling asset prices (stock prices, exchange rates), risk assessment, option pricing (Black-Scholes model relies on log-normal assumptions).
- Engineering: Analyzing the lifetime of components, predicting the size of particles in a suspension, modeling the strength of materials.
- Environmental Science: Studying pollutant concentrations, analyzing rainfall data, modeling the distribution of species in an ecosystem.
- Healthcare: Modeling disease prevalence, analyzing survival times, characterizing physiological measurements.
- Insurance: Modeling claim amounts, assessing risk profiles.
3. Challenges and Solutions:
While highly useful, working with the log-normal distribution presents some challenges:
- Parameter Estimation: Estimating μ and σ² requires careful consideration, often involving maximum likelihood estimation (MLE) or the method of moments.
- Interpretation: The parameters μ and σ² are not directly interpretable as the mean and standard deviation of X. Specific formulas are needed to calculate the mean, median, and standard deviation of the log-normal distribution itself.
- Data Transformation: In many applications, data transformation (taking logarithms) is crucial before applying standard statistical techniques.
4. Impact on Innovation:
The log-normal distribution's continued use drives innovation by providing a robust framework for modeling complex phenomena across various fields. Its application leads to better predictive models, more effective risk management, and improved decision-making, furthering advancements in several sectors.
Closing Insights: Summarizing the Core Discussion
The log-normal distribution is a powerful statistical tool with widespread applicability. Its ability to model positively skewed data, coupled with its mathematical tractability, makes it invaluable for analyzing various real-world phenomena. Understanding its properties and limitations is crucial for its effective application in diverse fields.
Exploring the Connection Between the Underlying Normal Distribution and the Log-Normal Distribution
The relationship between the underlying normal distribution (of ln(X)) and the resulting log-normal distribution (of X) is fundamental. The parameters of the normal distribution (μ and σ²) directly influence the shape, central tendency, and spread of the log-normal distribution.
Key Factors to Consider:
-
Roles and Real-World Examples: The mean (μ) of the underlying normal distribution determines the location (central tendency) of the log-normal distribution. The standard deviation (σ) affects the spread and skewness. For instance, a larger σ leads to a more pronounced right skew.
-
Risks and Mitigations: Misinterpreting the parameters of the underlying normal distribution as the mean and standard deviation of the log-normal distribution is a common mistake. It's crucial to use the correct formulas to calculate the mean, median, and standard deviation of the log-normal distribution.
-
Impact and Implications: The log-normal distribution's positive skewness implies a greater probability of extreme values on the higher end, which has crucial implications for risk assessment in finance and reliability engineering.
Conclusion: Reinforcing the Connection
The connection between the underlying normal distribution and the log-normal distribution is paramount. A thorough understanding of this link allows for correct parameter estimation, accurate probability calculations, and a proper interpretation of results in various applications.
Further Analysis: Examining the Mean, Median, and Standard Deviation of the Log-Normal Distribution in Greater Detail
While μ and σ² are the parameters of the underlying normal distribution, the mean, median, and standard deviation of the log-normal distribution itself are calculated as follows:
- Mean (E[X]): E[X] = exp(μ + σ²/2)
- Median (Mdn[X]): Mdn[X] = exp(μ)
- Standard Deviation (SD[X]): SD[X] = sqrt[ (exp(σ²) - 1) * exp(2μ + σ²) ]
These formulas highlight the non-linear relationship between the parameters of the underlying normal distribution and the moments of the log-normal distribution.
How to Calculate Probabilities and Quantiles
Calculating probabilities and quantiles for the log-normal distribution involves transforming the problem to the underlying normal distribution. If X ~ LogN(μ, σ²), and you want to find P(X ≤ x), you would first transform x to the normal scale using: z = (ln(x) - μ) / σ. Then, you can use standard normal distribution tables or software to find P(Z ≤ z), which is equal to P(X ≤ x).
FAQ Section: Answering Common Questions About the Log-Normal Distribution
-
What is a log-normal distribution? A log-normal distribution is a probability distribution of a random variable whose logarithm is normally distributed.
-
How is the log-normal distribution different from the normal distribution? The log-normal distribution is always positive and right-skewed, unlike the normal distribution which is symmetric and can take on negative values.
-
When should I use a log-normal distribution? Use a log-normal distribution when dealing with positive, right-skewed data, particularly in situations involving multiplicative effects or where the logarithm of the variable is normally distributed.
-
How do I fit a log-normal distribution to my data? Use maximum likelihood estimation or the method of moments to estimate the parameters (μ and σ²) from your data. Statistical software packages readily provide these functionalities.
-
What are the limitations of the log-normal distribution? It may not be appropriate for data with significant left skewness or data containing zero or negative values.
Practical Tips: Maximizing the Benefits of Using the Log-Normal Distribution
-
Data Transformation: Always consider transforming your data (taking natural logarithms) before applying statistical analysis.
-
Parameter Estimation: Utilize statistical software (R, Python, SPSS) to efficiently estimate the parameters of the underlying normal distribution.
-
Visual Inspection: Plot your data and a fitted log-normal distribution to visually assess the goodness of fit.
-
Consider Alternatives: If the log-normal distribution doesn't fit well, explore alternative distributions like the Weibull or Gamma distribution.
-
Understand Limitations: Remember the log-normal distribution's assumptions and limitations to ensure appropriate application.
Final Conclusion: Wrapping Up with Lasting Insights
The log-normal distribution, though seemingly specialized, is a remarkably versatile tool for modeling real-world phenomena across a multitude of disciplines. Its ability to handle positive, skewed data, coupled with the ease of calculation using standard statistical software, makes it an indispensable asset for researchers, engineers, and financial analysts. By understanding its properties, applications, and limitations, one can unlock valuable insights and build robust models to analyze and predict complex systems. This powerful distribution continues to be a cornerstone of statistical analysis, pushing the boundaries of our understanding and shaping advancements in numerous fields.
Latest Posts
Latest Posts
-
Segment Definition Business Benefits Examples
Apr 30, 2025
-
Seed Stock Definition
Apr 30, 2025
-
Security Market Line Sml Definition And Characteristics
Apr 30, 2025
-
Security Market Indicator Series Smis Definition
Apr 30, 2025
-
What Is Security Interest Definition And Legal Requirements
Apr 30, 2025
Related Post
Thank you for visiting our website which covers about Log Normal Distribution Definition Uses And How To Calculate . We hope the information provided has been useful to you. Feel free to contact us if you have any questions or need further assistance. See you next time and don't miss to bookmark.