Linear Relationship Definition
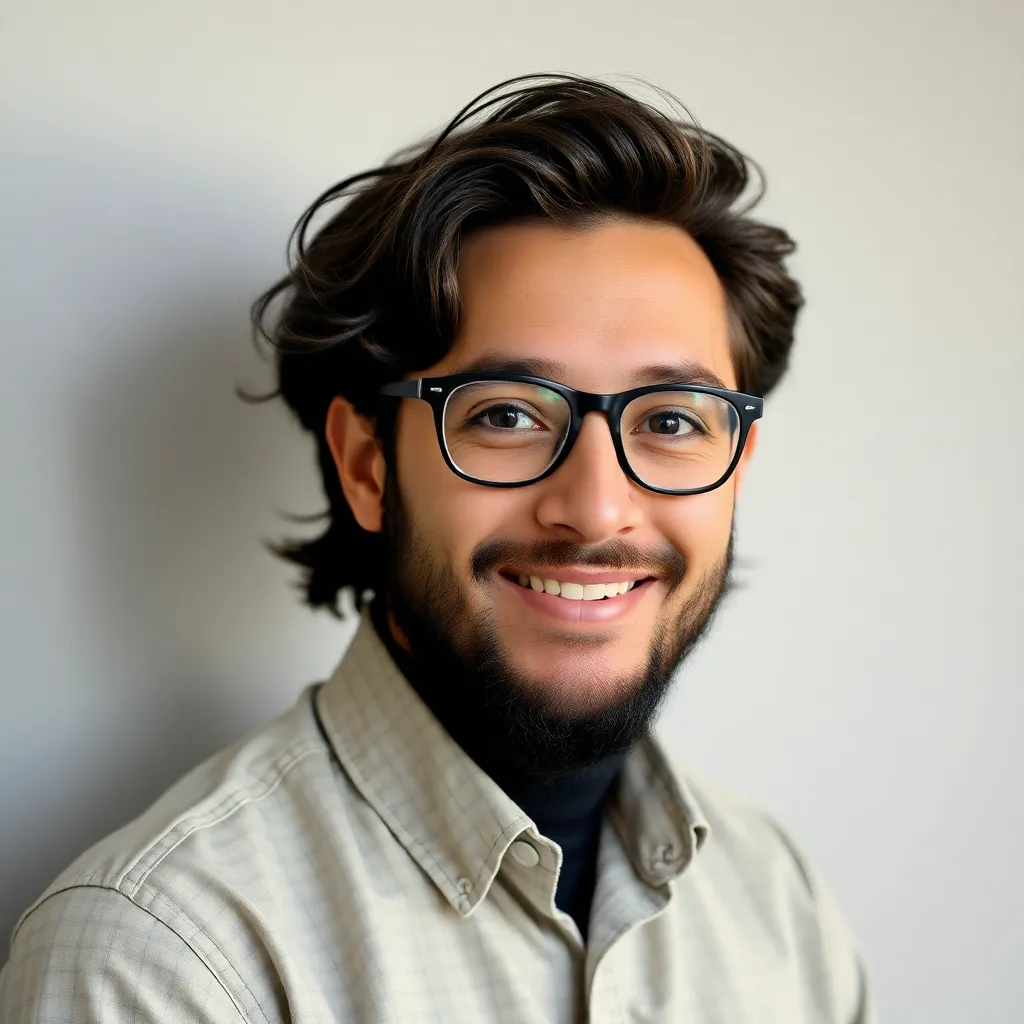
adminse
Apr 20, 2025 · 8 min read

Table of Contents
Unveiling the Linear Relationship: A Comprehensive Guide
What if the very foundation of countless predictions and analyses rests upon the understanding of linear relationships? This fundamental concept underpins a vast array of fields, from simple everyday observations to complex scientific models.
Editor’s Note: This article on linear relationships provides a comprehensive overview of this critical mathematical concept. Updated for 2024, it offers practical applications, insightful analysis, and clear explanations for readers of all backgrounds.
Why Linear Relationships Matter: Relevance, Practical Applications, and Industry Significance
Linear relationships are the bedrock of many predictive models and analytical techniques. Their simplicity belies their power; understanding them allows for the creation of straightforward yet effective tools across numerous disciplines. From predicting sales based on advertising spend in marketing to analyzing the correlation between temperature and ice cream sales in economics, linear relationships offer a readily accessible method for understanding and quantifying the connection between variables. Furthermore, they serve as a building block for more complex statistical models. In engineering, for example, understanding linear relationships is crucial for analyzing stress and strain in structures, predicting fluid flow, and designing control systems. The widespread application and foundational nature of this concept make it essential for anyone seeking a strong understanding of quantitative analysis.
Overview: What This Article Covers
This article provides a deep dive into linear relationships. We will explore the definition, core concepts, various representations, applications across diverse fields, and the limitations of this model. Readers will gain a comprehensive understanding of linear relationships, enabling them to identify, analyze, and interpret them effectively.
The Research and Effort Behind the Insights
This article synthesizes information from numerous textbooks on statistics, linear algebra, and calculus, as well as peer-reviewed research papers. Examples and illustrations are drawn from various fields to demonstrate the wide applicability of linear relationships. A structured approach ensures clarity and accessibility for a broad audience.
Key Takeaways:
- Definition and Core Concepts: A precise definition of linear relationships and their underlying principles.
- Representations: Exploring various ways to represent linear relationships (equations, graphs, tables).
- Slope and Intercept: Understanding the significance of these key parameters in describing a linear relationship.
- Applications: Examining real-world applications across diverse fields (science, engineering, economics, etc.).
- Limitations: Acknowledging the circumstances where a linear model might be inappropriate.
Smooth Transition to the Core Discussion:
Having established the significance of linear relationships, let's delve into their core definition and explore the various ways they can be represented and understood.
Exploring the Key Aspects of Linear Relationships
1. Definition and Core Concepts:
A linear relationship, in its simplest form, describes a relationship between two variables where a change in one variable is associated with a proportional change in the other. This proportionality implies a constant rate of change. Mathematically, a linear relationship can be represented by the equation y = mx + c, where:
- y: is the dependent variable (its value depends on the value of x).
- x: is the independent variable (its value is not influenced by y).
- m: is the slope, representing the rate of change of y with respect to x. A positive slope indicates a positive relationship (as x increases, y increases), while a negative slope indicates a negative relationship (as x increases, y decreases). A slope of zero indicates no relationship.
- c: is the y-intercept, representing the value of y when x is zero.
2. Representations of Linear Relationships:
Linear relationships can be represented in several ways, each offering unique insights:
- Algebraic Equation (y = mx + c): This provides a precise mathematical description of the relationship.
- Graph (Cartesian Coordinate System): Plotting the relationship on a graph visually demonstrates the linear nature, showing the slope and intercept clearly. A linear relationship will always appear as a straight line.
- Table of Values: A table listing pairs of x and y values satisfying the equation illustrates the relationship numerically.
3. Slope and Intercept:
The slope (m) and y-intercept (c) are crucial parameters that completely define a linear relationship. The slope indicates the steepness of the line and the direction of the relationship. The y-intercept indicates where the line crosses the y-axis. Understanding these parameters allows for accurate interpretation and prediction.
4. Applications Across Industries:
The applications of linear relationships are vast and span numerous fields:
- Physics: Describing motion (velocity and time), Ohm's Law (voltage and current), Hooke's Law (force and extension).
- Engineering: Analyzing stress and strain, designing control systems, predicting fluid flow.
- Economics: Modeling supply and demand, predicting sales based on advertising spend, analyzing correlations between economic variables.
- Finance: Predicting stock prices (with caution, as financial markets are rarely perfectly linear), calculating interest earned.
- Biology: Modeling population growth (under certain conditions), studying enzyme kinetics.
- Environmental Science: Modeling pollutant dispersion, predicting the relationship between carbon emissions and temperature increase.
5. Challenges and Limitations:
While linear relationships offer a simple and powerful tool for analysis, it's crucial to acknowledge their limitations:
- Non-Linear Relationships: Many real-world phenomena are not linear. Forcing a linear model onto non-linear data can lead to inaccurate predictions and misleading conclusions.
- Outliers: Extreme data points can significantly influence the slope and intercept, potentially skewing the analysis.
- Causation vs. Correlation: A linear relationship doesn't necessarily imply causation. Two variables may be linearly correlated without one causing the other. A third, unmeasured variable might be the underlying cause.
Closing Insights: Summarizing the Core Discussion
Linear relationships provide a fundamental framework for understanding and modeling the connections between variables. Their simplicity facilitates easy interpretation and application across diverse fields. However, careful consideration of their limitations and the potential for non-linearity is crucial for accurate analysis and reliable predictions.
Exploring the Connection Between Correlation and Linear Relationships
Correlation measures the strength and direction of a linear relationship between two variables. A strong positive correlation indicates a strong positive linear relationship, a strong negative correlation indicates a strong negative linear relationship, and a correlation close to zero suggests a weak or non-existent linear relationship. It's important to note that correlation does not equal causation.
Key Factors to Consider:
- Roles and Real-World Examples: Correlation coefficients (like Pearson's r) quantify the linear relationship. For example, a high positive correlation between ice cream sales and temperature indicates a strong positive linear relationship, but doesn't mean ice cream sales cause higher temperatures.
- Risks and Mitigations: Misinterpreting correlation as causation is a significant risk. Careful consideration of potential confounding variables is necessary.
- Impact and Implications: Understanding correlation helps identify potential relationships and guides further investigation, but it shouldn't be the sole basis for drawing causal conclusions.
Conclusion: Reinforcing the Connection
The connection between correlation and linear relationships is vital. Correlation provides a quantitative measure of the strength and direction of a linear relationship, guiding further analysis. However, it's crucial to avoid the fallacy of assuming causation based solely on correlation.
Further Analysis: Examining Regression Analysis in Greater Detail
Regression analysis is a statistical technique used to model the relationship between a dependent variable and one or more independent variables. Linear regression specifically models linear relationships. It allows for the estimation of the slope and intercept of the line of best fit, which minimizes the sum of squared errors between the observed data and the predicted values.
FAQ Section: Answering Common Questions About Linear Relationships
-
What is a linear relationship? A linear relationship is a relationship between two variables where a change in one variable is associated with a proportional change in the other, resulting in a straight line when graphed.
-
How do I determine if a relationship is linear? Visually inspect a scatter plot of the data. If the points roughly fall along a straight line, a linear relationship is likely. Statistical tests can also confirm linearity.
-
What if my data doesn't show a perfect linear relationship? Real-world data rarely shows perfect linearity. A linear model can still be useful as an approximation, but consider the limitations and potential for non-linearity.
-
What are the applications of linear relationships? Linear relationships are applied across countless fields, including physics, engineering, economics, finance, biology, and environmental science.
-
How do I interpret the slope and intercept of a linear relationship? The slope represents the rate of change, while the intercept represents the value of the dependent variable when the independent variable is zero.
Practical Tips: Maximizing the Benefits of Understanding Linear Relationships
-
Visualize Your Data: Always start by creating a scatter plot to visually inspect the data and assess the potential for a linear relationship.
-
Calculate Correlation: Use correlation coefficients to quantify the strength and direction of the relationship.
-
Perform Regression Analysis: Use linear regression to estimate the line of best fit and obtain the slope and intercept.
-
Consider Limitations: Acknowledge the limitations of linear models and be aware of potential non-linearity, outliers, and the difference between correlation and causation.
-
Seek Further Analysis: If your data doesn't conform to a linear model, explore other statistical methods to model the relationship.
Final Conclusion: Wrapping Up with Lasting Insights
Linear relationships represent a fundamental building block in quantitative analysis, providing a simple yet powerful framework for understanding and modeling connections between variables. By understanding their definition, representations, applications, and limitations, individuals can effectively leverage this concept across various disciplines. However, it's crucial to always critically evaluate the appropriateness of a linear model and consider the broader context of the data. The ability to identify, analyze, and interpret linear relationships is a cornerstone of effective data analysis and a valuable skill in a data-driven world.
Latest Posts
Latest Posts
-
Segment Definition Business Benefits Examples
Apr 30, 2025
-
Seed Stock Definition
Apr 30, 2025
-
Security Market Line Sml Definition And Characteristics
Apr 30, 2025
-
Security Market Indicator Series Smis Definition
Apr 30, 2025
-
What Is Security Interest Definition And Legal Requirements
Apr 30, 2025
Related Post
Thank you for visiting our website which covers about Linear Relationship Definition . We hope the information provided has been useful to you. Feel free to contact us if you have any questions or need further assistance. See you next time and don't miss to bookmark.