Line Of Best Fit Definition How It Works And Calculation
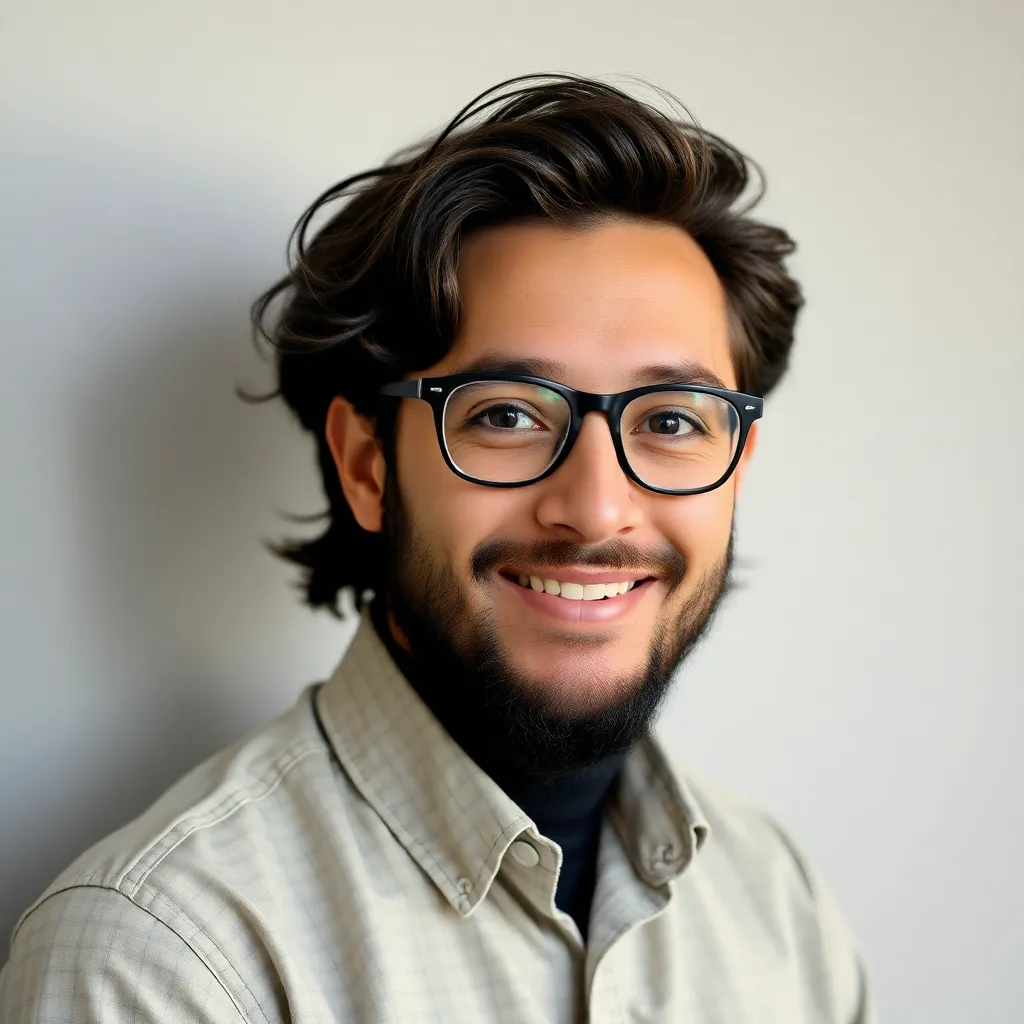
adminse
Apr 20, 2025 · 9 min read

Table of Contents
Unveiling the Line of Best Fit: Definition, Mechanics, and Calculation
What if predicting future trends and understanding complex relationships hinged on a single, elegantly simple line? The line of best fit, a cornerstone of statistical analysis, empowers us to do just that, revealing hidden patterns within data and offering invaluable insights across diverse fields.
Editor’s Note: This comprehensive article on the line of best fit provides a detailed explanation of its definition, working mechanism, and calculation methods. We've included various examples and scenarios to illustrate its practical applications and significance in data analysis. Updated for 2024.
Why the Line of Best Fit Matters: Relevance, Practical Applications, and Industry Significance
The line of best fit, also known as the regression line, is a fundamental concept in statistics with far-reaching applications. It allows us to model the relationship between two variables, identifying trends and making predictions. This capability is invaluable across numerous disciplines:
- Business and Economics: Forecasting sales, predicting consumer behavior, and analyzing market trends.
- Science and Engineering: Modeling experimental data, identifying correlations between variables, and making predictions in various scientific fields.
- Healthcare: Analyzing patient data, predicting disease progression, and evaluating the effectiveness of treatments.
- Finance: Predicting stock prices, assessing investment risk, and managing portfolios.
Understanding and applying the line of best fit empowers decision-makers to gain a deeper understanding of complex phenomena, make informed predictions, and ultimately, improve outcomes.
Overview: What This Article Covers
This article provides a comprehensive exploration of the line of best fit. We will cover:
- Definition and Core Concepts: A clear definition of the line of best fit and the underlying principles.
- Methods of Calculation: A step-by-step guide to calculating the line of best fit using the least squares method.
- Interpreting the Results: Understanding the slope and intercept of the regression line and their implications.
- Applications and Examples: Real-world examples demonstrating the practical applications of the line of best fit.
- Limitations and Considerations: Acknowledging the limitations and potential pitfalls of using the line of best fit.
- Advanced Techniques: Briefly touching upon more advanced regression techniques.
The Research and Effort Behind the Insights
This article draws upon established statistical principles and widely accepted methodologies. The calculations and explanations provided are based on standard linear regression techniques, commonly taught in introductory statistics courses and employed across various data analysis software packages.
Key Takeaways:
- Definition: A line that best represents the relationship between two variables in a dataset.
- Calculation: Primarily uses the method of least squares to minimize the sum of squared errors.
- Interpretation: The slope and intercept reveal the nature and strength of the relationship.
- Applications: Wide-ranging applications across numerous fields for prediction and analysis.
- Limitations: Assumptions underlying linear regression need to be considered.
Smooth Transition to the Core Discussion:
Having established the importance and scope of the line of best fit, let's delve into its core aspects. We'll begin with a formal definition and then explore the mechanics of its calculation.
Exploring the Key Aspects of the Line of Best Fit
Definition and Core Concepts:
The line of best fit is a straight line that best represents the linear relationship between two variables in a dataset. The "best" fit is determined by minimizing the sum of the squared distances between the data points and the line. This method, known as the least squares method, ensures that the line is as close as possible to all the data points collectively. The equation of the line is typically represented as:
y = mx + c
where:
- y is the dependent variable (the variable we are trying to predict).
- x is the independent variable (the variable used for prediction).
- m is the slope of the line (representing the rate of change of y with respect to x).
- c is the y-intercept (the value of y when x is 0).
Calculating the Line of Best Fit (Least Squares Method):
The least squares method involves finding the values of 'm' and 'c' that minimize the sum of the squared vertical distances between each data point and the line. The formulas for calculating 'm' and 'c' are derived from calculus and involve calculating sums of products and squares of the x and y values. Let's break down the process:
-
Calculate the means: Find the mean of the x values (x̄) and the mean of the y values (ȳ).
-
Calculate the deviations: For each data point, calculate the deviations from the means: (xᵢ - x̄) and (yᵢ - ȳ).
-
Calculate the sum of products: Calculate the sum of the products of the deviations: Σ[(xᵢ - x̄)(yᵢ - ȳ)].
-
Calculate the sum of squared deviations for x: Calculate the sum of the squared deviations for x: Σ(xᵢ - x̄)².
-
Calculate the slope (m): The slope is calculated using the following formula:
m = Σ[(xᵢ - x̄)(yᵢ - ȳ)] / Σ(xᵢ - x̄)²
-
Calculate the y-intercept (c): The y-intercept is calculated using the following formula:
c = ȳ - m * x̄
Interpreting the Results:
Once the slope (m) and y-intercept (c) are calculated, the equation of the line of best fit can be written. The slope indicates the change in y for every unit change in x. A positive slope indicates a positive correlation (as x increases, y increases), while a negative slope indicates a negative correlation (as x increases, y decreases). The y-intercept represents the value of y when x is 0.
Applications and Examples:
Consider a scenario where a company wants to predict its sales based on advertising expenditure. By plotting advertising expenditure (x) against sales (y), a line of best fit can be calculated. The slope would indicate the increase in sales for every dollar increase in advertising, and the y-intercept would represent the sales if no advertising were done.
Limitations and Considerations:
The line of best fit assumes a linear relationship between the variables. If the relationship is non-linear, using a linear regression will not accurately represent the data. Outliers (data points significantly far from the other data points) can heavily influence the line of best fit. Furthermore, correlation does not imply causation. Just because two variables are correlated does not mean that one causes the other.
Advanced Techniques:
While this article focuses on simple linear regression, more advanced techniques like multiple linear regression (involving multiple independent variables) and non-linear regression (for non-linear relationships) exist.
Exploring the Connection Between Correlation and the Line of Best Fit
The correlation coefficient (r) measures the strength and direction of the linear relationship between two variables. It ranges from -1 to +1. A value of +1 indicates a perfect positive correlation, -1 indicates a perfect negative correlation, and 0 indicates no linear correlation. The correlation coefficient is closely related to the line of best fit. A higher absolute value of r indicates a better fit of the line to the data.
Key Factors to Consider:
- Roles and Real-World Examples: The correlation coefficient provides a quantitative measure of the linear association, helping to assess the reliability of the line of best fit for prediction. For instance, a strong positive correlation between study hours and exam scores would justify using a line of best fit to predict exam scores based on study hours.
- Risks and Mitigations: A low correlation coefficient indicates a weak linear relationship, suggesting that the line of best fit may not be a reliable predictor. In such cases, considering non-linear models or investigating other factors might be necessary.
- Impact and Implications: Understanding the correlation coefficient helps to interpret the results of the line of best fit, providing a measure of confidence in the predictions made.
Conclusion: Reinforcing the Connection
The relationship between correlation and the line of best fit is fundamental in data analysis. A strong correlation supports the use of a linear regression model, while a weak correlation suggests limitations in using the line of best fit for prediction.
Further Analysis: Examining Correlation in Greater Detail
The correlation coefficient is calculated using the following formula:
r = Σ[(xᵢ - x̄)(yᵢ - ȳ)] / √[Σ(xᵢ - x̄)² * Σ(yᵢ - ȳ)²]
Understanding the calculation and interpretation of the correlation coefficient is crucial for assessing the reliability and validity of the line of best fit.
FAQ Section: Answering Common Questions About the Line of Best Fit
Q: What is the line of best fit used for?
A: It's used to model the relationship between two variables, make predictions, and identify trends in data.
Q: What if my data doesn't show a linear relationship?
A: In that case, a linear regression (and the line of best fit) is not appropriate. Consider using non-linear regression techniques.
Q: How do I know if my line of best fit is a good fit?
A: Examine the correlation coefficient (r). A value close to +1 or -1 indicates a strong fit. Visual inspection of the scatter plot and the line can also be helpful.
Q: Can I use the line of best fit to predict values outside the range of my data?
A: Extrapolation (predicting outside the data range) can be risky and unreliable. It's generally best to limit predictions to the range of the observed data.
Practical Tips: Maximizing the Benefits of the Line of Best Fit
-
Visualize your data: Create a scatter plot to visually assess the relationship between your variables before calculating the line of best fit.
-
Check for outliers: Identify and address outliers that may significantly influence the results.
-
Consider the correlation coefficient: Use the correlation coefficient to assess the strength and direction of the linear relationship.
-
Use appropriate software: Statistical software packages can simplify the calculation and interpretation of the line of best fit.
-
Understand the limitations: Be aware of the assumptions and limitations of linear regression before drawing conclusions.
Final Conclusion: Wrapping Up with Lasting Insights
The line of best fit is a powerful tool for analyzing data and making predictions. By understanding its definition, calculation, interpretation, and limitations, one can effectively utilize this statistical method across various fields to gain valuable insights from data. However, always remember that the line of best fit represents a model, an approximation of reality, and its validity depends on the underlying assumptions and the nature of the data. Careful consideration of these factors is crucial for drawing meaningful conclusions and avoiding misinterpretations.
Latest Posts
Latest Posts
-
Second World Definition
Apr 30, 2025
-
Second Surplus Definition
Apr 30, 2025
-
Second Chance Loan Definition
Apr 30, 2025
-
Second Life Economy Definition
Apr 30, 2025
-
Sec Rw Filing Definition
Apr 30, 2025
Related Post
Thank you for visiting our website which covers about Line Of Best Fit Definition How It Works And Calculation . We hope the information provided has been useful to you. Feel free to contact us if you have any questions or need further assistance. See you next time and don't miss to bookmark.