Leptokurtic Distributions Definition Example Vs Platykurtic
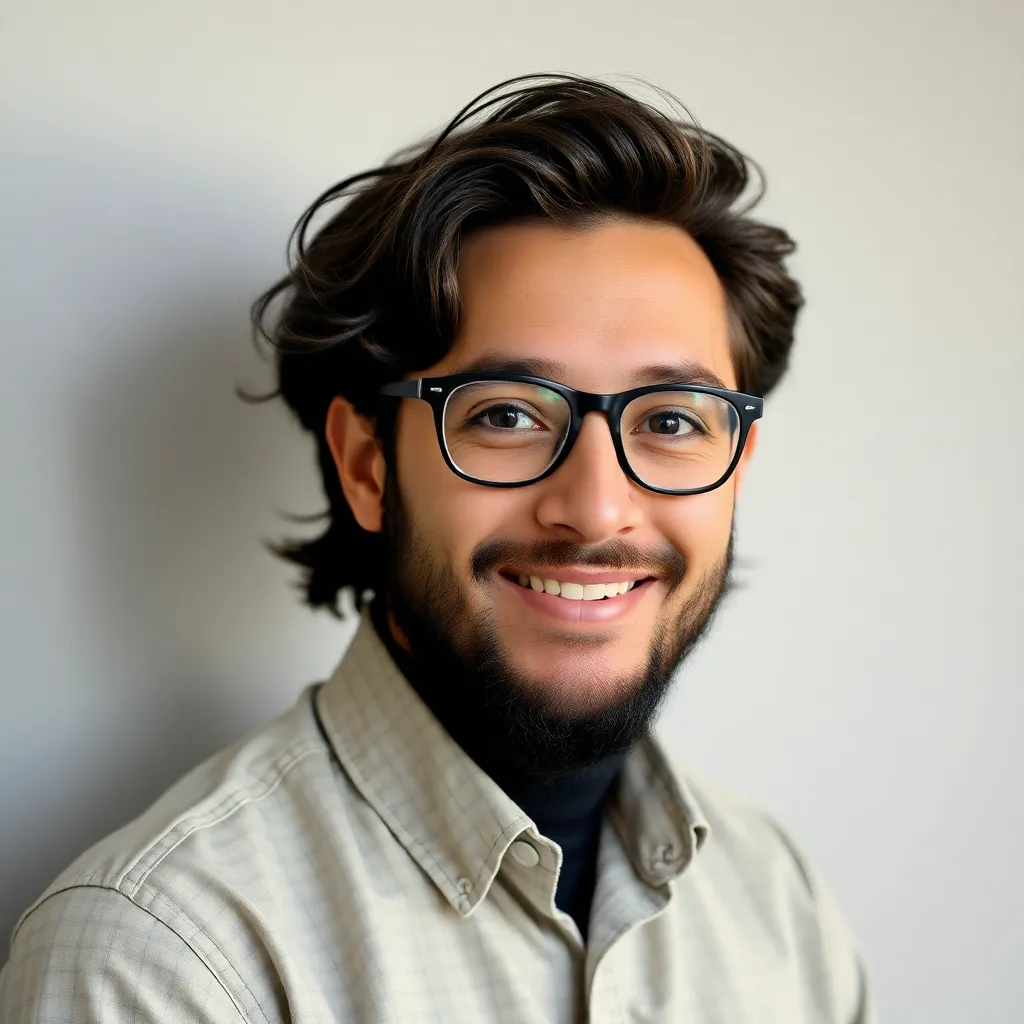
adminse
Apr 20, 2025 · 7 min read

Table of Contents
Unveiling the Secrets of Kurtosis: Leptokurtic vs. Platykurtic Distributions
What if a deeper understanding of kurtosis could unlock crucial insights into data analysis and predictive modeling? The subtle differences between leptokurtic and platykurtic distributions hold the key to more accurate interpretations and informed decisions.
Editor’s Note: This article provides a comprehensive exploration of leptokurtic and platykurtic distributions, examining their definitions, distinguishing features, real-world examples, and practical implications. The information presented is current and based on established statistical principles.
Why Kurtosis Matters: Beyond the Bell Curve
Kurtosis, a measure of the "tailedness" and "peakedness" of a probability distribution, is a critical statistical concept often overlooked. While the normal distribution serves as a benchmark, many real-world datasets deviate significantly, exhibiting either heavier tails and sharper peaks (leptokurtic) or lighter tails and flatter peaks (platykurtic) than the normal distribution. Understanding these variations is paramount for accurate data interpretation, model building, and risk assessment across diverse fields, including finance, healthcare, and engineering. This understanding allows for more robust predictions and informed decision-making. Failing to account for leptokurtic or platykurtic distributions can lead to inaccurate risk assessments and flawed models.
Overview: What This Article Covers
This in-depth analysis will meticulously define kurtosis and its subtypes, leptokurtic and platykurtic distributions. We will delve into the mathematical foundations, explore illustrative real-world examples, and contrast the properties of these distributions. Furthermore, we will discuss the practical implications of identifying leptokurtic and platykurtic distributions in statistical modeling and data analysis. The article will conclude with a comprehensive FAQ section and actionable tips for better understanding and interpreting kurtosis in your data.
The Research and Effort Behind the Insights
This article draws upon established statistical literature, peer-reviewed research papers, and widely accepted statistical methodologies. The explanations are grounded in theoretical principles and supplemented by illustrative examples. The aim is to provide readers with a clear, concise, and accurate understanding of leptokurtic and platykurtic distributions, enabling them to confidently apply this knowledge in their respective fields.
Key Takeaways:
- Definition and Core Concepts: A precise definition of kurtosis and its relationship to the shape of a distribution.
- Leptokurtic Distributions: Detailed explanation, examples, and implications.
- Platykurtic Distributions: Detailed explanation, examples, and implications.
- Mesokurtic Distributions: A brief overview for comparison.
- Practical Applications: How recognizing leptokurtic and platykurtic distributions improves data analysis and modeling.
- Limitations and Considerations: Addressing potential pitfalls and nuances.
Smooth Transition to the Core Discussion
Having established the importance of understanding kurtosis, let's now embark on a detailed exploration of leptokurtic and platykurtic distributions, beginning with a clear definition of kurtosis itself.
Exploring the Key Aspects of Kurtosis
Kurtosis is a statistical measure that quantifies the "tailedness" and "peakedness" of a probability distribution. It describes the concentration of data around the mean and in the tails of the distribution. There are different ways to measure kurtosis, but the most common is excess kurtosis, which compares the kurtosis of the distribution to the kurtosis of a normal distribution. A normal distribution has an excess kurtosis of 0.
Definition and Core Concepts:
Mathematically, kurtosis is the fourth standardized moment of a distribution. While the formula itself is complex, the interpretation is key: a higher kurtosis value indicates heavier tails and a sharper peak (or more data clustered around the mean), while a lower kurtosis indicates lighter tails and a flatter peak (more data spread out).
Leptokurtic Distributions:
A leptokurtic distribution is characterized by heavier tails and a sharper peak compared to a normal distribution. Its excess kurtosis is greater than 0. This means more data points are concentrated around the mean and in the extreme tails. These distributions are prone to outliers.
Examples of Leptokurtic Distributions:
- Financial Returns: Stock market returns often exhibit leptokurtosis, with periods of extreme volatility (heavy tails) interspersed with periods of relative calm (sharp peak). This is crucial for risk management.
- Income Distribution: In many countries, income distribution is leptokurtic, with a large concentration of individuals around the mean income and a smaller number of individuals with extremely high or low incomes.
- Heights of Adult Males: While generally following a normal distribution, including outliers like exceptionally tall or short individuals can lead to a leptokurtic distribution.
- The t-distribution: For smaller degrees of freedom, the t-distribution is leptokurtic. It's frequently used in statistical inference when the population standard deviation is unknown.
Platykurtic Distributions:
A platykurtic distribution is characterized by lighter tails and a flatter peak compared to a normal distribution. Its excess kurtosis is less than 0. This indicates that data is more evenly spread out, with fewer data points concentrated around the mean and in the extreme tails.
Examples of Platykurtic Distributions:
- Uniform Distribution: A perfect example, as the probability is constant across the entire range.
- Certain Measurement Errors: Measurement errors, after careful calibration and control, might demonstrate a platykurtic distribution due to the reduction of extreme values.
- Simulated Data: Certain types of simulated data, particularly those designed to be uniformly distributed, will exhibit platykurtosis.
Mesokurtic Distributions:
For completeness, it's crucial to mention mesokurtic distributions. These have an excess kurtosis of approximately 0 and closely resemble a normal distribution.
Closing Insights: Summarizing the Core Discussion
The distinction between leptokurtic and platykurtic distributions is not merely an academic exercise; it holds significant practical implications. Understanding the shape of a distribution allows for more accurate risk assessments, more appropriate statistical tests, and more robust model building. Ignoring the kurtosis of your data can lead to inaccurate conclusions and potentially flawed decisions.
Exploring the Connection Between Outliers and Kurtosis
Outliers play a crucial role in shaping the kurtosis of a distribution. Leptokurtic distributions are particularly sensitive to outliers because the presence of extreme values significantly increases the fourth moment, leading to a higher kurtosis value. In contrast, platykurtic distributions are less affected by outliers because the data is more evenly spread out.
Key Factors to Consider:
- Roles and Real-World Examples: Outliers in financial data (e.g., Black Swan events) drastically influence the leptokurtosis observed in stock market returns.
- Risks and Mitigations: Failure to account for outliers in a leptokurtic distribution can lead to inaccurate model predictions and risk assessments. Robust statistical methods are needed.
- Impact and Implications: The presence of outliers can mask the true underlying distribution, leading to misleading conclusions about the population.
Conclusion: Reinforcing the Connection
The connection between outliers and kurtosis is undeniable. Understanding this relationship is critical for proper data analysis and model building. Appropriate data cleaning techniques and robust statistical methods are essential when dealing with leptokurtic distributions and their susceptibility to outliers.
Further Analysis: Examining Outliers in Greater Detail
Outliers are data points that fall significantly outside the typical range of values observed in a dataset. They can arise due to various reasons, including measurement errors, data entry errors, or genuine extreme values reflecting the true nature of the underlying phenomenon. Identifying and handling outliers appropriately is a crucial step in any data analysis process. Various techniques exist, including visual inspection (box plots, scatter plots), statistical methods (Z-scores, IQR), and robust statistical methods.
FAQ Section: Answering Common Questions About Kurtosis
Q: What is the difference between kurtosis and skewness?
A: Kurtosis measures the "tailedness" and "peakedness" of a distribution, while skewness measures the asymmetry of the distribution. A distribution can be leptokurtic and symmetrical, or platykurtic and skewed.
Q: How can I calculate kurtosis?
A: Statistical software packages (R, Python, SPSS) readily provide kurtosis calculations. The formula involves the fourth moment of the data. Remember, excess kurtosis is usually reported.
Q: Why is kurtosis important in financial modeling?
A: Leptokurtosis in financial returns signifies the risk of infrequent but potentially large losses (Black Swan events), requiring specialized risk models and hedging strategies.
Practical Tips: Maximizing the Benefits of Understanding Kurtosis
- Visual Inspection: Always start with visual tools (histograms, box plots) to get a sense of the distribution's shape.
- Calculate Kurtosis: Use statistical software to obtain the excess kurtosis value.
- Consider Outliers: Investigate the presence of outliers and their potential impact on kurtosis.
- Choose Appropriate Models: Select statistical models and methods appropriate for the identified distribution type.
Final Conclusion: Wrapping Up with Lasting Insights
Understanding leptokurtic and platykurtic distributions is essential for accurate data interpretation and robust statistical modeling. By acknowledging the role of kurtosis and outliers, analysts can gain deeper insights into data, leading to more informed decisions and more accurate predictions across various fields. The key takeaway is that the simple bell curve is often an oversimplification, and understanding deviations from normality—particularly in the context of kurtosis—is crucial for sound data analysis.
Latest Posts
Latest Posts
-
Secondary Mortgage Market Definition Purpose And Example
Apr 30, 2025
-
Secondary Market Annuity Sma Definition
Apr 30, 2025
-
Secondary Liquidity Definition
Apr 30, 2025
-
Secondary Liability Definition
Apr 30, 2025
-
Secondary Business Definition And Overview
Apr 30, 2025
Related Post
Thank you for visiting our website which covers about Leptokurtic Distributions Definition Example Vs Platykurtic . We hope the information provided has been useful to you. Feel free to contact us if you have any questions or need further assistance. See you next time and don't miss to bookmark.