Kurtosis Definition Types And Importance
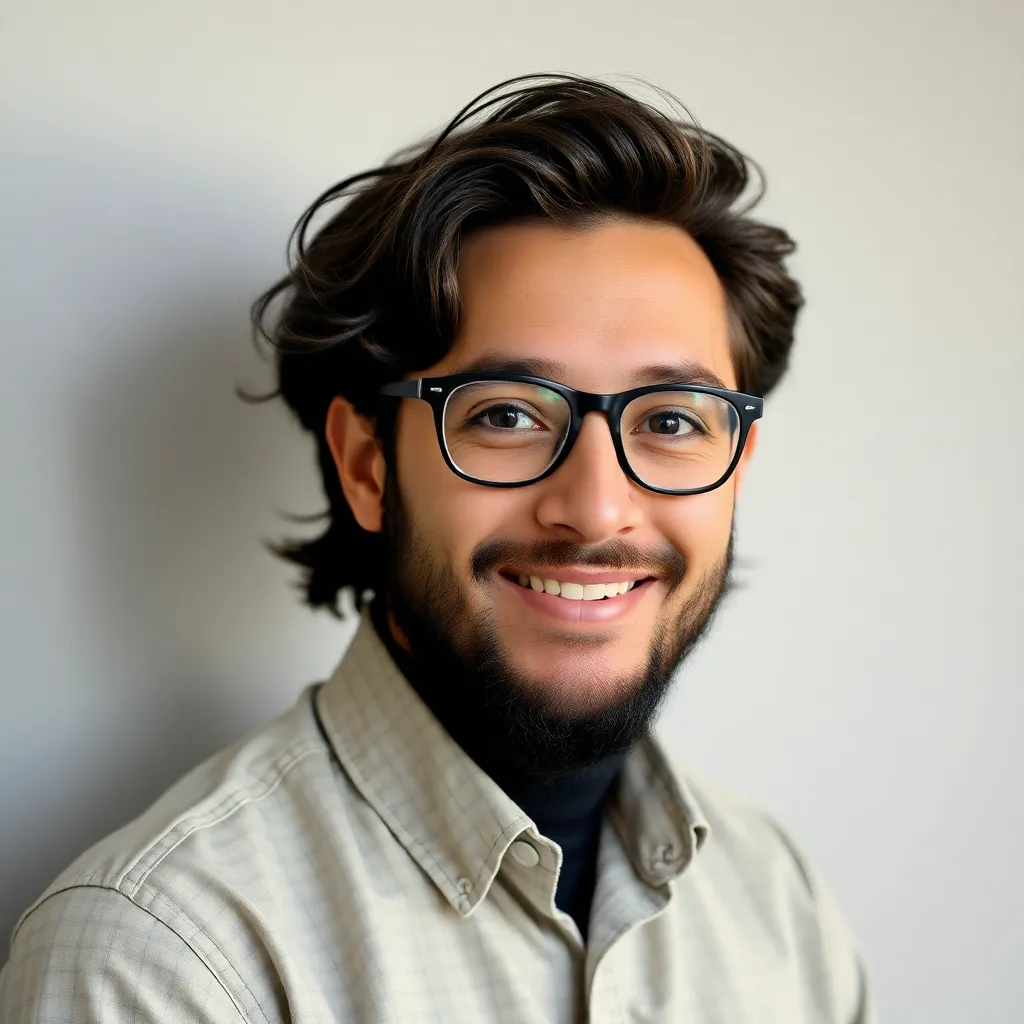
adminse
Apr 20, 2025 · 9 min read

Table of Contents
Unveiling Kurtosis: Definition, Types, and Importance
What if the seemingly simple measure of kurtosis holds the key to unlocking deeper insights into data distribution and predictive modeling? This crucial statistical concept offers invaluable information beyond the typical mean and standard deviation, providing a more complete picture of data variability.
Editor's Note: This article on kurtosis provides a comprehensive overview of its definition, types, and importance in various fields. The information presented is current and based on established statistical principles.
Why Kurtosis Matters: Relevance, Practical Applications, and Industry Significance
Kurtosis, a measure of the "tailedness" and "peakedness" of a probability distribution, plays a significant role in various fields, from finance and risk management to quality control and machine learning. Understanding kurtosis allows for a more nuanced interpretation of data, revealing patterns that might be missed by simply considering the mean and standard deviation. Its applications range from identifying outliers and assessing risk to optimizing investment strategies and improving model accuracy. In essence, kurtosis provides a crucial layer of information for a more complete understanding of data variability. It helps in identifying distributions with unusually heavy tails or sharp peaks, which can significantly impact decision-making processes.
Overview: What This Article Covers
This article will delve into the core aspects of kurtosis, starting with its precise definition and exploring the different types of kurtosis (mesokurtic, leptokurtic, and platykurtic). We will then examine the practical applications of kurtosis across various disciplines, emphasizing its importance in statistical modeling, risk assessment, and anomaly detection. Furthermore, the article will address potential challenges in interpreting kurtosis and offer strategies to mitigate misinterpretations. Finally, we’ll explore the relationship between kurtosis and other statistical measures, highlighting its synergistic value when used in conjunction with other descriptive statistics.
The Research and Effort Behind the Insights
This article is the result of extensive research, drawing upon established statistical textbooks, peer-reviewed publications, and authoritative online resources. The information presented is based on well-established statistical principles and aims to provide readers with an accurate and comprehensive understanding of kurtosis. Every concept is explained clearly and supported by illustrative examples to enhance comprehension.
Key Takeaways:
- Definition and Core Concepts: A clear and concise definition of kurtosis and its foundational principles.
- Types of Kurtosis: A detailed explanation of mesokurtic, leptokurtic, and platykurtic distributions and their characteristics.
- Practical Applications: Examples of how kurtosis is used in different fields, such as finance, engineering, and data science.
- Interpretational Challenges: Discussion of potential pitfalls and strategies for effective interpretation.
- Relationship with other Statistical Measures: Understanding kurtosis in the broader context of descriptive statistics.
Smooth Transition to the Core Discussion:
Now that the foundational importance of kurtosis has been established, let’s delve deeper into its core aspects, starting with a precise definition and exploration of its various types.
Exploring the Key Aspects of Kurtosis
Definition and Core Concepts:
Kurtosis is a statistical measure that describes the "tailedness" and "peakedness" of a probability distribution. It quantifies the concentration of data around the mean and the presence of outliers in the tails of the distribution. A higher kurtosis indicates a sharper peak and heavier tails (more outliers), while a lower kurtosis suggests a flatter peak and lighter tails. The most common measure of kurtosis is the excess kurtosis, calculated as the fourth standardized moment minus 3. Subtracting 3 allows for a convenient comparison with the normal distribution, which has an excess kurtosis of 0.
Types of Kurtosis:
Based on the value of excess kurtosis, probability distributions are classified into three categories:
-
Mesokurtic: A mesokurtic distribution has an excess kurtosis of approximately 0. This indicates a distribution similar in shape to a normal distribution. The data points are relatively evenly distributed around the mean, with neither exceptionally high peaks nor heavy tails.
-
Leptokurtic: A leptokurtic distribution has a positive excess kurtosis (greater than 0). This indicates a distribution with a sharper peak and heavier tails than a normal distribution. Leptokurtic distributions have a higher concentration of data points near the mean and a greater number of outliers in the tails. This suggests that extreme values are more likely to occur than in a normal distribution. Examples include the Laplace distribution and Student's t-distribution.
-
Platykurtic: A platykurtic distribution has a negative excess kurtosis (less than 0). This signifies a distribution with a flatter peak and lighter tails than a normal distribution. Platykurtic distributions have less data concentrated around the mean and fewer outliers in the tails. This suggests that extreme values are less likely to occur than in a normal distribution. The uniform distribution is an example of a platykurtic distribution.
Applications Across Industries:
Kurtosis finds application in a wide range of fields:
-
Finance: In finance, kurtosis is crucial for risk management. Leptokurtic distributions of asset returns indicate a higher probability of extreme events (large gains or losses), requiring more robust risk management strategies. This is particularly relevant for portfolio optimization and assessing market risk.
-
Engineering: In engineering, kurtosis is used to assess the robustness and reliability of systems. A leptokurtic distribution of component failure rates might indicate the need for more stringent quality control measures.
-
Data Science: In data science, kurtosis helps identify outliers and improve the accuracy of predictive models. Understanding the kurtosis of the data can inform the choice of appropriate statistical methods and potentially improve model performance. For example, in machine learning, kurtosis can help detect anomalies in datasets.
Challenges and Solutions:
While kurtosis provides valuable insights, there are challenges in its interpretation:
-
Sensitivity to Outliers: Kurtosis is highly sensitive to outliers. Even a few extreme values can significantly inflate the kurtosis value, potentially misleading the interpretation. Robust measures of kurtosis, less sensitive to outliers, might be more appropriate in such cases.
-
Misinterpretation: High kurtosis doesn't automatically imply the presence of important information; it simply indicates a deviation from normality. The context of the data and other statistical measures should be considered alongside kurtosis.
Impact on Innovation:
The understanding and application of kurtosis contribute to innovation across different fields:
-
Improved Risk Management: A deeper understanding of kurtosis in financial modeling leads to more accurate risk assessments and better risk mitigation strategies.
-
Enhanced Quality Control: In manufacturing, understanding kurtosis can guide improvements in quality control processes, resulting in higher reliability and fewer failures.
-
More Accurate Predictive Models: In data science, incorporating kurtosis into model development can lead to more accurate and robust predictions.
Exploring the Connection Between Sample Size and Kurtosis
The reliability of kurtosis estimations significantly depends on the sample size. Small sample sizes can lead to unstable and unreliable kurtosis estimates, potentially resulting in misinterpretations. Larger sample sizes generally produce more stable and accurate estimations. This is because, with larger samples, the distribution of the sample kurtosis converges more closely to the true population kurtosis. Therefore, it's crucial to consider the sample size when interpreting kurtosis, particularly in cases where the sample size is small.
Key Factors to Consider:
-
Roles and Real-World Examples: Small sample sizes can lead to significant variability in kurtosis estimations, potentially misrepresenting the true distribution. For instance, in a small survey on customer satisfaction, a few extreme responses can drastically alter the calculated kurtosis, leading to inaccurate conclusions.
-
Risks and Mitigations: The risk of misinterpreting kurtosis increases with smaller sample sizes. To mitigate this, researchers often employ larger sample sizes or use robust measures of kurtosis less sensitive to outliers. Non-parametric methods may also be preferred in cases with small sample sizes.
-
Impact and Implications: Inaccurate kurtosis estimation can lead to flawed conclusions, especially in hypothesis testing and model building. It can affect the selection of appropriate statistical methods and ultimately impact decision-making based on the analysis.
Conclusion: Reinforcing the Connection:
The connection between sample size and kurtosis is paramount. Researchers must carefully consider sample size when interpreting kurtosis to avoid misinterpretations and ensure accurate conclusions. The use of robust techniques and a critical assessment of the data are vital when dealing with smaller sample sizes.
Further Analysis: Examining Sample Size in Greater Detail
The impact of sample size on kurtosis estimation can be further explored using simulation studies. By generating numerous samples of different sizes from a known distribution, the variability of the estimated kurtosis can be assessed. This allows for a quantitative understanding of the relationship between sample size and kurtosis reliability. Such simulations can also inform the selection of an appropriate sample size for achieving a desired level of accuracy in kurtosis estimation.
FAQ Section: Answering Common Questions About Kurtosis
What is kurtosis?
Kurtosis is a statistical measure that describes the degree of peakedness and tail heaviness of a probability distribution. It quantifies how much the distribution's data is concentrated around the mean and the likelihood of extreme values.
How is kurtosis calculated?
The most common measure is excess kurtosis, calculated as the fourth standardized moment minus 3. The formula involves the sum of the fourth powers of the deviations from the mean, divided by the fourth power of the standard deviation.
What are the different types of kurtosis?
Distributions are categorized as mesokurtic (excess kurtosis ≈ 0), leptokurtic (excess kurtosis > 0), and platykurtic (excess kurtosis < 0).
Why is kurtosis important?
Kurtosis provides crucial information about data variability beyond the mean and standard deviation, impacting risk assessment, model building, and outlier detection.
How can I interpret kurtosis values?
Interpret kurtosis in context, considering the sample size, the presence of outliers, and other descriptive statistics. High kurtosis doesn't always mean significant information; it signifies deviation from normality.
Practical Tips: Maximizing the Benefits of Kurtosis Analysis
- Consider Sample Size: Ensure sufficient sample size for reliable kurtosis estimation.
- Assess Outliers: Check for and address potential outliers impacting kurtosis.
- Use Robust Measures: Employ robust kurtosis measures if outliers are a concern.
- Interpret in Context: Don’t rely solely on kurtosis; consider other statistical measures.
- Visualize the Data: Use histograms and other visualizations to understand the distribution.
Final Conclusion: Wrapping Up with Lasting Insights
Kurtosis, while often overlooked, is a fundamental statistical measure offering invaluable insights into data variability. By carefully considering its definition, types, and practical applications, while acknowledging the challenges in its interpretation, researchers and analysts can gain a more comprehensive understanding of their data. Its proper application can lead to improved risk management, enhanced quality control, and more accurate predictive modeling across diverse disciplines. Understanding and correctly interpreting kurtosis significantly improves the depth and accuracy of data analysis, contributing to more informed and effective decision-making.
Latest Posts
Latest Posts
-
Secondary Liquidity Definition
Apr 30, 2025
-
Secondary Liability Definition
Apr 30, 2025
-
Secondary Business Definition And Overview
Apr 30, 2025
-
Second Lien Debt Definition Risks Example
Apr 30, 2025
-
Second World Definition
Apr 30, 2025
Related Post
Thank you for visiting our website which covers about Kurtosis Definition Types And Importance . We hope the information provided has been useful to you. Feel free to contact us if you have any questions or need further assistance. See you next time and don't miss to bookmark.