Information Coefficient Ic Definition Example And Formula
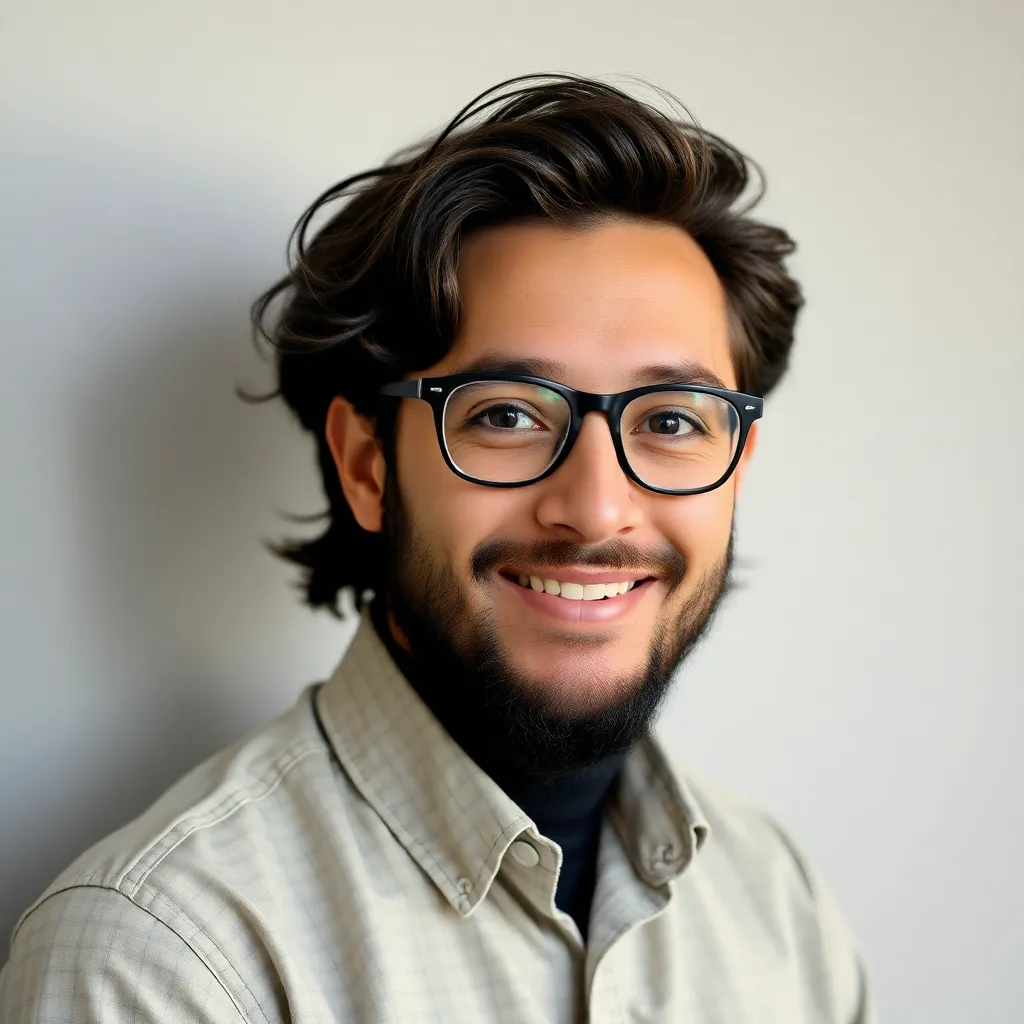
adminse
Apr 24, 2025 · 9 min read

Table of Contents
Unlocking the Power of Prediction: A Deep Dive into Information Coefficient (IC)
What if the secret to superior investment returns lies in understanding the Information Coefficient (IC)? This crucial metric offers invaluable insights into the predictive power of any investment model, driving better decision-making and improved performance.
Editor’s Note: This article on the Information Coefficient (IC) provides a comprehensive overview of its definition, calculation, interpretation, and practical applications in investment management. The information presented here is for educational purposes and should not be considered financial advice.
Why the Information Coefficient Matters
In the world of quantitative finance and investment management, accurately predicting future asset performance is the holy grail. Numerous models are employed to forecast returns, ranging from complex statistical algorithms to sophisticated machine learning techniques. However, evaluating the effectiveness of these models is critical. This is where the Information Coefficient (IC) steps in. It quantifies the predictive power of a specific factor, signal, or model in predicting asset returns. Understanding and optimizing IC is crucial for building robust investment strategies that consistently outperform the market. Its significance spans diverse applications, including portfolio construction, risk management, and algorithmic trading.
Overview: What This Article Covers
This article will provide a thorough understanding of the Information Coefficient (IC), encompassing its definition, the formula for calculating it, diverse examples illustrating its practical application, and a detailed discussion of its strengths and limitations. Readers will gain a comprehensive framework for interpreting IC values and leveraging this metric to enhance their investment strategies.
The Research and Effort Behind the Insights
This article draws upon extensive research from leading academic publications, industry reports, and practical experience in quantitative finance. The explanations and examples presented are based on established statistical methodologies and real-world applications of the IC.
Key Takeaways:
- Definition and Core Concepts: A clear explanation of the Information Coefficient and its fundamental principles.
- Formula and Calculation: A step-by-step guide on how to calculate the IC using different methods.
- Examples and Interpretations: Real-world examples showcasing the application and interpretation of IC values in various contexts.
- Strengths and Limitations: A balanced assessment of the IC’s capabilities and potential pitfalls.
- Applications in Investment Management: How the IC is used in portfolio construction, risk management, and factor-based investing.
Smooth Transition to the Core Discussion
Now that we understand the importance of the IC, let's delve into its core aspects. We'll begin with a precise definition and then explore its calculation, interpretation, and practical uses.
Exploring the Key Aspects of the Information Coefficient (IC)
1. Definition and Core Concepts:
The Information Coefficient (IC) is a statistical measure that assesses the correlation between a predictive signal (e.g., a factor score, a model's output) and the subsequent return of an asset. It represents the strength of the linear relationship between the predicted return and the actual realized return. A higher IC indicates a stronger predictive ability, while a lower IC suggests a weaker or non-existent relationship. It’s a crucial metric for evaluating the quality and effectiveness of any forecasting model or signal within an investment strategy. Importantly, the IC doesn't measure the magnitude of the return itself, but rather the accuracy of the prediction.
2. Formula and Calculation:
The IC is typically calculated using the rank correlation between the predicted and realized returns. This is because the magnitude of returns is often less important than the direction (up or down). The most common rank correlation used is Spearman's rank correlation coefficient, which is calculated as follows:
Spearman's Rank Correlation Coefficient (ρ):
ρ = 1 - (6Σd²)/(n(n²-1))
Where:
- d = the difference between the ranks of the predicted and actual returns for each asset.
- n = the number of assets.
Steps to Calculate the IC:
- Rank the predicted returns for all assets in ascending order. Assign a rank to each asset.
- Rank the actual realized returns for all assets in ascending order. Assign a rank to each asset.
- Calculate the difference (d) between the ranks for each asset.
- Square each difference (d²).
- Sum the squared differences (Σd²).
- Plug the values into the formula above to calculate ρ (Spearman's rank correlation). This ρ is your IC.
Alternative Methods:
While Spearman's rank correlation is prevalent, other correlation measures like Pearson's correlation can be used if the data meets the assumptions of normality and linearity. However, Spearman's is generally preferred for its robustness to outliers and its focus on rank rather than magnitude.
3. Examples and Interpretations:
Let's consider a simplified example:
Suppose you have a model that predicts the returns of five stocks:
Stock | Predicted Return Rank | Actual Return Rank | d | d² |
---|---|---|---|---|
A | 1 | 2 | -1 | 1 |
B | 2 | 1 | 1 | 1 |
C | 3 | 3 | 0 | 0 |
D | 4 | 4 | 0 | 0 |
E | 5 | 5 | 0 | 0 |
Using the formula: ρ = 1 - (6 * 2) / (5 * (25 - 1)) = 1 - 12/120 = 0.9
In this case, the IC is 0.9, indicating a very strong positive relationship between the predicted and actual returns. An IC of 1 would represent perfect prediction, while an IC of 0 would indicate no predictive ability. Negative IC values suggest an inverse relationship – the model predicts the opposite of what happens.
4. Strengths and Limitations:
Strengths:
- Robustness to outliers (especially Spearman's).
- Focuses on directional accuracy, not magnitude.
- Simple to interpret.
- Widely used and understood in the industry.
Limitations:
- Only measures linear relationships; non-linear relationships might be missed.
- Sensitive to the number of assets used in the calculation; more assets can lead to a more stable estimate.
- Doesn't consider transaction costs or other practical factors.
- Doesn't directly tell us about the magnitude of potential profits.
5. Applications in Investment Management:
The IC finds diverse uses in investment management:
- Factor Model Evaluation: Assess the predictive power of different factors (e.g., value, momentum, size).
- Portfolio Construction: Select assets with higher IC scores for inclusion in a portfolio.
- Risk Management: Identify models or signals with low or negative ICs, mitigating potential losses.
- Algorithmic Trading: Optimize trading strategies by identifying signals with high IC.
- Backtesting and Model Validation: Evaluate the performance of investment strategies over historical data.
Exploring the Connection Between Tracking Error and Information Coefficient
Tracking error measures the deviation of a portfolio's return from its benchmark. The connection between tracking error and IC is indirect but significant. A higher IC generally implies better predictive ability, leading to potentially lower tracking error (if the model is used to actively manage the portfolio to outperform the benchmark). However, a high IC doesn't guarantee low tracking error; other factors like portfolio turnover and market volatility also play a role.
Key Factors to Consider:
- Data Quality: Inaccurate or noisy data will severely impact IC calculations.
- Model Complexity: Overly complex models can lead to overfitting and low out-of-sample IC.
- Time Horizon: The IC can vary across different time horizons; a model with a high IC over a short period might perform poorly over the long term.
Risks and Mitigations:
- Data Mining Bias: Overfitting a model to historical data can lead to artificially inflated IC values. Robust cross-validation techniques are crucial to mitigate this risk.
- Survivorship Bias: Using data that excludes failed assets can lead to overly optimistic IC estimates.
- Market Regime Changes: A model with a high IC in one market regime might perform poorly in another.
Impact and Implications:
Understanding and optimizing the IC is vital for successful investment management. By improving the predictive power of investment models, managers can enhance portfolio performance, manage risk more effectively, and improve overall investment outcomes.
Conclusion: Reinforcing the Connection
The relationship between tracking error and IC highlights the importance of accurate prediction in portfolio management. While not directly proportional, a higher IC contributes to the possibility of lower tracking error when striving for active management. Careful consideration of data quality, model complexity, and potential biases is crucial for reliable IC estimations.
Further Analysis: Examining Tracking Error in Greater Detail
Tracking error, often measured as standard deviation of the difference between portfolio returns and benchmark returns, offers a distinct perspective on portfolio performance. A lower tracking error is often desirable when closely mirroring a benchmark, while higher tracking error can be acceptable (even desired) in active strategies aiming for higher returns. Understanding the nuances of tracking error and its interplay with IC provides a more holistic view of investment strategy performance.
FAQ Section: Answering Common Questions About the Information Coefficient
Q: What is the ideal IC value?
A: There's no single "ideal" IC value. It depends on the context, the investment strategy, and the level of noise in the data. An IC above 0.1 is generally considered good, while an IC above 0.25 is exceptional.
Q: How can I improve the IC of my investment model?
A: Improving the IC involves refining your predictive signal, using better data, improving model selection and tuning, and addressing potential biases such as data mining bias.
Q: Can the IC be negative?
A: Yes, a negative IC indicates that the model's predictions are inversely related to the actual returns – meaning the model is predicting the opposite of what happens in reality.
Practical Tips: Maximizing the Benefits of the Information Coefficient
- Focus on Data Quality: Ensure the data used for IC calculation is accurate, reliable, and free from biases.
- Use Appropriate Correlation Measures: Choose the correlation measure (Spearman's, Pearson's, etc.) that best suits the data and the goals of the analysis.
- Validate Out-of-Sample: Always test the model's performance on data not used for training to avoid overfitting.
- Monitor IC Regularly: The IC can change over time, so it's essential to monitor it regularly and adjust the model or strategy as needed.
Final Conclusion: Wrapping Up with Lasting Insights
The Information Coefficient is a fundamental tool in quantitative finance, providing a crucial metric for evaluating the predictive power of investment models. By understanding its calculation, interpretation, and limitations, investment managers can significantly enhance their ability to build robust, effective, and profitable investment strategies. The journey towards optimizing IC involves a continuous process of data refinement, model improvement, and careful consideration of potential biases. Ultimately, mastering the IC enhances the ability to navigate the complexities of financial markets and achieve better investment outcomes.
Latest Posts
Latest Posts
-
Instructing Bank Definition
Apr 24, 2025
-
Institutional Shares Definition Who Can Buy Them And Examples
Apr 24, 2025
-
Institutional Ownership Defined And Explained
Apr 24, 2025
-
Institutional Investor Index Definition
Apr 24, 2025
-
Institutional Deposits Corporation Idc Definition
Apr 24, 2025
Related Post
Thank you for visiting our website which covers about Information Coefficient Ic Definition Example And Formula . We hope the information provided has been useful to you. Feel free to contact us if you have any questions or need further assistance. See you next time and don't miss to bookmark.