Heteroscedasticity Definition Simple Meaning And Types Explained
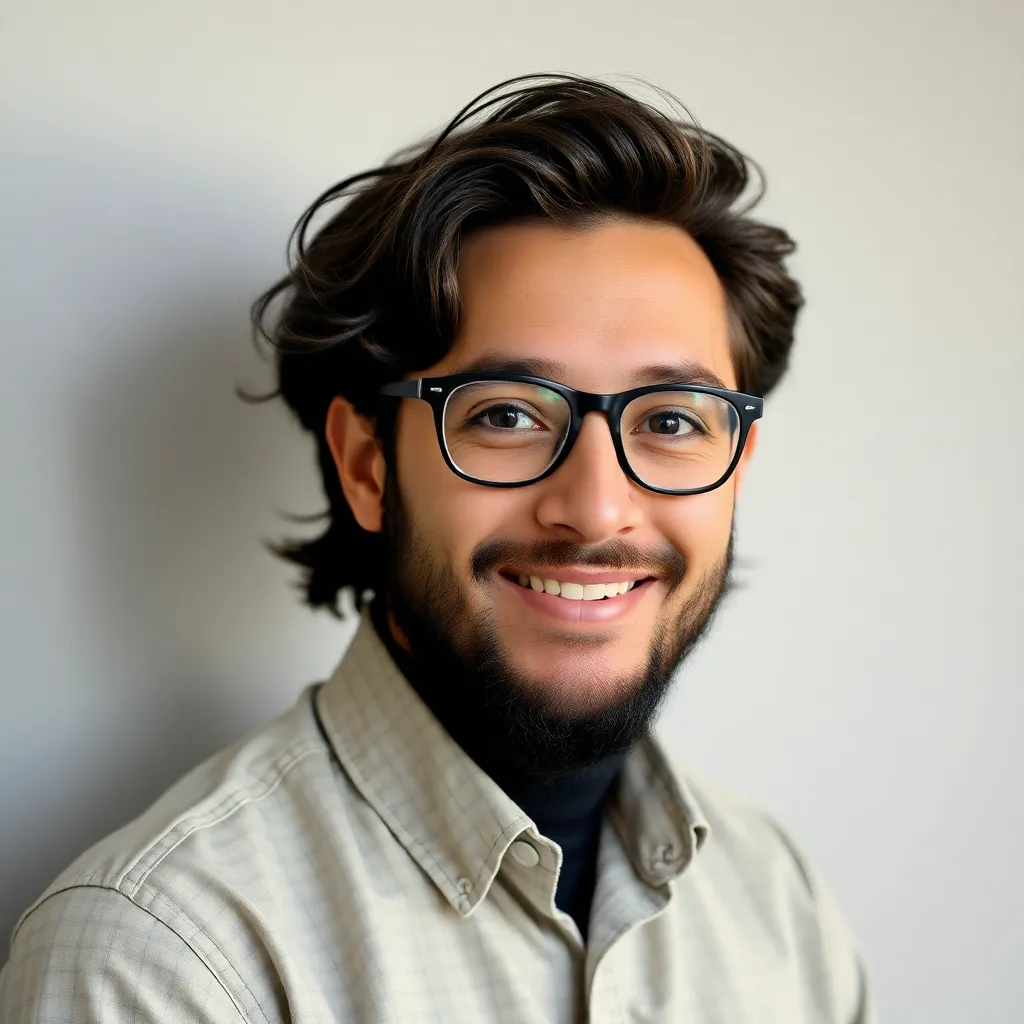
adminse
Apr 24, 2025 · 9 min read

Table of Contents
Understanding Heteroscedasticity: Simple Meaning, Types, and Implications
What if the accuracy of your statistical model depends on the data points themselves? Heteroscedasticity, a common yet often overlooked statistical phenomenon, significantly impacts the reliability of your analyses, demanding careful consideration and potentially necessitating corrective measures.
Editor’s Note: This article on heteroscedasticity provides a comprehensive overview of this important statistical concept. It explains its meaning in simple terms, details the different types, and explores the implications for various statistical analyses. Readers will gain a practical understanding of how to identify and address heteroscedasticity in their own work.
Why Heteroscedasticity Matters:
Heteroscedasticity, a mouthful of a word, simply means that the variability (or scatter) of your data points is not consistent across all levels of your independent variable(s). In simpler terms, the spread of your data changes depending on where you are on the x-axis of your graph. Imagine plotting income (y-axis) against age (x-axis). You might find that the income variability is much smaller for younger people (less spread in their income) and much larger for older people (greater spread in their income – some are very wealthy, some less so). This is heteroscedasticity. Ignoring it can lead to inaccurate conclusions and unreliable statistical inferences. Understanding its presence and impact is critical for the validity of numerous statistical analyses, from simple linear regressions to complex econometric models. Its relevance spans across various fields, including economics, finance, social sciences, and medicine, impacting the interpretation of research findings and the efficacy of predictive models.
Overview: What This Article Covers:
This article provides a detailed exploration of heteroscedasticity. We will begin by defining the concept and contrasting it with its opposite, homoscedasticity. Then, we will delve into the various types of heteroscedasticity, exploring their characteristics and manifestations. We will discuss the consequences of ignoring heteroscedasticity in statistical analysis and present methods for detection and correction. Finally, we will examine practical examples and offer actionable advice for researchers and analysts.
The Research and Effort Behind the Insights:
This article is the culmination of extensive research, drawing upon established statistical literature, textbooks, and peer-reviewed articles. The explanations provided are designed to be accessible to a broad audience, avoiding overly technical jargon while maintaining scientific rigor. Each concept is illustrated with examples to enhance understanding and practical application.
Key Takeaways:
- Definition and Core Concepts: A clear understanding of heteroscedasticity and its implications.
- Types of Heteroscedasticity: Identification of different patterns of unequal variances.
- Consequences of Heteroscedasticity: The impact on statistical inferences and model reliability.
- Detection Methods: Techniques to identify the presence of heteroscedasticity.
- Corrective Measures: Strategies to mitigate the effects of heteroscedasticity.
Smooth Transition to the Core Discussion:
Now that we understand the importance of addressing heteroscedasticity, let's delve deeper into its intricacies. We will start by defining the core concept and then explore its different forms.
Exploring the Key Aspects of Heteroscedasticity:
1. Definition and Core Concepts:
Homoscedasticity (the opposite of heteroscedasticity) refers to the condition where the variance of the error term in a regression model is constant across all levels of the independent variable. In simpler terms, the spread of the data points around the regression line remains consistent. Heteroscedasticity, therefore, is the violation of this assumption – the variance of the error term is not constant. This means that the variability of the dependent variable differs systematically across different values of the independent variable. The presence of heteroscedasticity invalidates some of the assumptions underlying many statistical tests, leading to inaccurate standard errors, t-statistics, and p-values.
2. Types of Heteroscedasticity:
Heteroscedasticity can manifest in various forms:
-
Linear Heteroscedasticity: The variance of the error term increases or decreases linearly with the independent variable. This is a relatively straightforward pattern to identify.
-
Quadratic Heteroscedasticity: The relationship between the variance of the error term and the independent variable follows a quadratic pattern (a U-shape or an inverted U-shape).
-
Multiplicative Heteroscedasticity: The variance of the error term is proportional to the value of the independent variable raised to some power.
-
Random Heteroscedasticity: The variance of the error term varies randomly without any discernible pattern. This is the most difficult type to detect and correct.
3. Consequences of Heteroscedasticity:
Ignoring heteroscedasticity has significant consequences:
-
Inefficient Estimates: The ordinary least squares (OLS) estimators remain unbiased, but they are no longer the most efficient. This means that the estimates are less precise than they could be.
-
Inaccurate Standard Errors: Standard errors of the regression coefficients become biased, leading to inaccurate confidence intervals and hypothesis tests. This can result in incorrect conclusions about the significance of the independent variables.
-
Inflated Type I Error Rate: The probability of rejecting the null hypothesis when it is actually true (a false positive) increases. This means that you are more likely to conclude that there is a relationship between variables when there isn't one.
-
Unreliable p-values: The p-values associated with the regression coefficients are unreliable and cannot be trusted for hypothesis testing.
4. Detection Methods:
Several methods can help detect heteroscedasticity:
-
Visual Inspection: Plotting the residuals (the difference between the observed and predicted values) against the predicted values or the independent variable can reveal patterns suggestive of heteroscedasticity. A cone-shaped or fanning pattern indicates heteroscedasticity.
-
Breusch-Pagan Test: A statistical test that assesses the significance of the relationship between the squared residuals and the independent variables. A significant p-value indicates the presence of heteroscedasticity.
-
White Test: A more general test than the Breusch-Pagan test, which can detect more complex forms of heteroscedasticity.
-
Goldfeld-Quandt Test: This test divides the data into two groups and compares the variances of the residuals in each group. A significant difference suggests heteroscedasticity.
5. Corrective Measures:
Several methods can be employed to address heteroscedasticity:
-
Weighted Least Squares (WLS): This method assigns different weights to the observations based on their variances. Observations with larger variances are given smaller weights, reducing their influence on the regression results.
-
Transformations: Transforming the dependent variable (e.g., taking the logarithm) or the independent variables can sometimes stabilize the variance.
-
Robust Standard Errors: These are standard errors that are less sensitive to heteroscedasticity. They provide more reliable inference even in the presence of heteroscedasticity. Methods like the White robust standard errors are commonly used.
-
Generalized Least Squares (GLS): This is a more general approach that explicitly models the heteroscedasticity and incorporates this information into the estimation process. This often requires specifying a structure for the variance-covariance matrix of the errors.
Closing Insights: Summarizing the Core Discussion:
Heteroscedasticity is a crucial consideration in statistical analysis. Its presence invalidates some key assumptions of common statistical procedures, leading to inaccurate inferences and unreliable results. By understanding its various forms, employing appropriate detection methods, and implementing corrective measures, researchers and analysts can ensure the validity and robustness of their statistical analyses.
Exploring the Connection Between Outliers and Heteroscedasticity:
Outliers, data points that lie significantly far from the rest of the data, can often contribute to or exacerbate heteroscedasticity. Extreme values can inflate the variance of the error term, particularly if they are clustered at certain levels of the independent variable. This is because the outliers increase the spread of the data, leading to unequal variances across different ranges of the independent variable.
Key Factors to Consider:
-
Roles and Real-World Examples: Consider a study examining the relationship between advertising expenditure and sales revenue. A few exceptionally high-spending companies with unexpectedly low sales could create heteroscedasticity. Similarly, in income versus age data, a few extremely high-earning individuals in older age groups will contribute to the increased variance.
-
Risks and Mitigations: Failing to address outlier-induced heteroscedasticity can lead to biased coefficient estimates and inaccurate predictions. Mitigations include carefully examining outliers, investigating their causes, and considering robust regression techniques. Winsorizing or trimming the data might be an option in some cases but should be done cautiously and justified.
-
Impact and Implications: Uncorrected heteroscedasticity stemming from outliers can lead to misleading conclusions about the relationship between variables, potentially impacting resource allocation, policy decisions, or business strategies.
Conclusion: Reinforcing the Connection:
The relationship between outliers and heteroscedasticity highlights the interconnectedness of various statistical issues. Outliers, if not properly handled, can create heteroscedasticity, ultimately jeopardizing the reliability of statistical inferences. Thorough data exploration, appropriate diagnostic checks, and careful consideration of robust methods are crucial for producing valid and dependable statistical results.
Further Analysis: Examining Outliers in Greater Detail:
Detecting and dealing with outliers requires a multifaceted approach:
-
Visual Inspection: Scatter plots and box plots are useful for initially identifying potential outliers.
-
Statistical Methods: Methods such as the Z-score or interquartile range (IQR) can quantitatively identify outliers based on their deviation from the mean or median.
-
Investigating the Cause: It’s crucial to understand why an outlier exists. Is it a genuine data point, or is it due to data entry error, measurement error, or a fundamentally different underlying process?
FAQ Section: Answering Common Questions About Heteroscedasticity:
Q: What is the most common type of heteroscedasticity?
A: While various types exist, linear heteroscedasticity, where variance increases or decreases linearly with the independent variable, is frequently encountered.
Q: Can heteroscedasticity be ignored if the sample size is large?
A: While larger sample sizes can sometimes mitigate the impact, heteroscedasticity still leads to inefficient estimates and unreliable standard errors, even with large samples. Addressing it is generally recommended.
Q: Are there any non-parametric methods to handle heteroscedasticity?
A: Yes, non-parametric methods such as robust regression techniques offer alternatives to OLS when heteroscedasticity is present. These methods are less sensitive to violations of distributional assumptions.
Q: How do I choose between WLS and robust standard errors?
A: WLS requires an understanding or an assumption about the nature of the heteroscedasticity; robust standard errors are a more general approach that don't necessitate this, making them more widely applicable.
Practical Tips: Maximizing the Benefits of Addressing Heteroscedasticity:
-
Always plot your data: Visual inspection is a crucial first step in detecting heteroscedasticity.
-
Conduct diagnostic tests: Employ statistical tests like the Breusch-Pagan or White test to formally assess for heteroscedasticity.
-
Consider data transformations: Explore logarithmic or other transformations to stabilize variance.
-
Utilize robust standard errors: Even if you cannot completely eliminate heteroscedasticity, using robust standard errors will improve the reliability of your inferences.
-
Employ weighted least squares when appropriate: If the pattern of heteroscedasticity is clearly defined, WLS can be an effective approach.
Final Conclusion: Wrapping Up with Lasting Insights:
Heteroscedasticity, while a complex statistical concept, is crucial for ensuring the accuracy and reliability of your statistical analyses. By understanding its meaning, different forms, detection methods, and corrective techniques, researchers can avoid drawing erroneous conclusions and build more robust and trustworthy statistical models. The impact of ignoring this issue can be significant, ranging from inaccurate estimates and flawed p-values to flawed decision-making based on unreliable inferences. Proactive assessment and appropriate mitigation strategies are essential for maintaining the integrity of your research and analysis.
Latest Posts
Latest Posts
-
Hotellings Theory Definition How It Works And History
Apr 24, 2025
-
Hot Wallet Definition Types Examples And Safety Tips
Apr 24, 2025
-
Hot Money Definition
Apr 24, 2025
-
Hot Issue Definition
Apr 24, 2025
-
Hot Ipo Definition
Apr 24, 2025
Related Post
Thank you for visiting our website which covers about Heteroscedasticity Definition Simple Meaning And Types Explained . We hope the information provided has been useful to you. Feel free to contact us if you have any questions or need further assistance. See you next time and don't miss to bookmark.