Hedonic Regression Definition
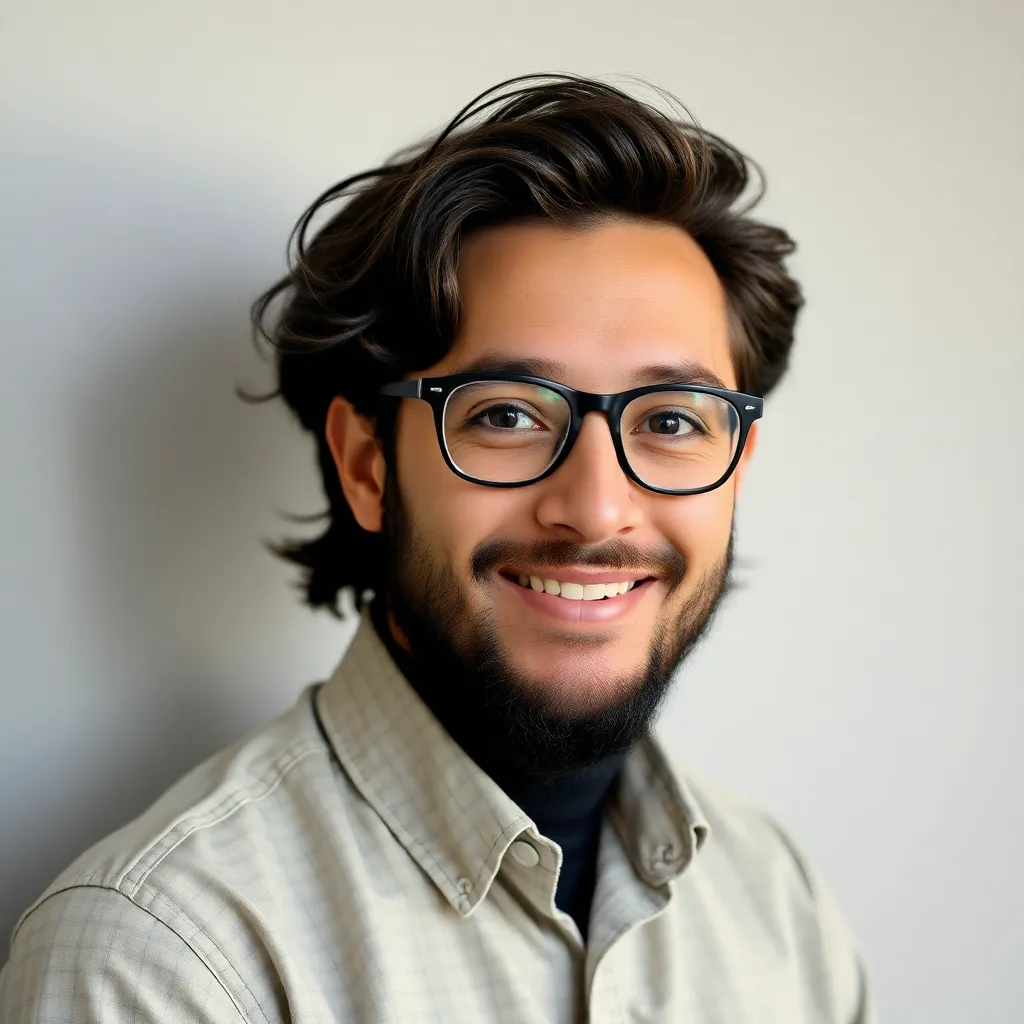
adminse
Apr 24, 2025 · 8 min read

Table of Contents
Hedonic Regression: Unraveling the Mystery of Happiness and Prices
What if understanding how happiness influences pricing could revolutionize market analysis? Hedonic regression, a powerful statistical tool, is already doing just that, offering invaluable insights into consumer behavior and market dynamics.
Editor’s Note: This article on hedonic regression was published today, providing readers with the most up-to-date information and analysis on this crucial economic and statistical modeling technique. It explores its definition, applications, limitations, and future implications for researchers and businesses alike.
Why Hedonic Regression Matters:
Hedonic regression is a crucial tool for economists, market researchers, and data scientists. It allows for the disentanglement of the value of a product or service into its individual characteristics, ultimately helping to understand how consumers value specific features and how these preferences affect pricing. This understanding is vital for pricing strategies, product development, and market forecasting. Its applications extend far beyond simple goods; it's used to understand the pricing of complex bundles like houses, cars, and even jobs, providing a nuanced perspective on consumer behavior and market forces.
Overview: What This Article Covers:
This article provides a comprehensive overview of hedonic regression. We will define the technique, explore its underlying assumptions, delve into its practical applications across various industries, examine its limitations, and discuss the future of this powerful analytical tool. Readers will gain a thorough understanding of hedonic regression, enabling them to critically evaluate its use and appreciate its significance in diverse fields.
The Research and Effort Behind the Insights:
This article draws upon extensive research from academic journals, industry reports, and real-world case studies. The information presented is meticulously vetted to ensure accuracy and reliability. The analysis integrates both theoretical frameworks and practical examples to offer a balanced and comprehensive understanding of hedonic regression.
Key Takeaways:
- Definition and Core Concepts: A clear definition of hedonic regression and its fundamental principles.
- Practical Applications: Diverse examples of hedonic regression's use across various industries.
- Assumptions and Limitations: A critical assessment of the technique's underlying assumptions and potential weaknesses.
- Advanced Techniques and Extensions: Exploring variations and extensions of the basic hedonic regression model.
- Future Implications: Discussing the ongoing development and future potential of hedonic regression.
Smooth Transition to the Core Discussion:
Having established the importance of hedonic regression, let's delve into the specifics, starting with its definition and core principles.
Exploring the Key Aspects of Hedonic Regression:
1. Definition and Core Concepts:
Hedonic regression is a statistical technique used to estimate the implicit prices of individual characteristics of a good or service. Instead of directly observing the price of a specific attribute, hedonic regression uses regression analysis to decompose the overall price of a complex good into the values of its constituent attributes. This decomposition allows researchers to quantify the marginal contribution of each attribute to the overall price. For example, when analyzing house prices, hedonic regression can separate the effects of lot size, number of bedrooms, proximity to schools, and other features on the final price, revealing how much each characteristic contributes to the overall value.
The model typically takes the form of a multiple linear regression, where the dependent variable is the price of the good and the independent variables are the various attributes. The estimated coefficients of the independent variables represent the implicit prices of the corresponding attributes, holding other factors constant.
2. Applications Across Industries:
The versatility of hedonic regression makes it a valuable tool across numerous sectors:
- Real Estate: Determining the value of different house characteristics (size, location, amenities).
- Automotive: Assessing the impact of features (engine size, fuel efficiency, safety features) on car prices.
- Labor Economics: Evaluating the contribution of skills, experience, and education to wage levels.
- Environmental Economics: Estimating the value of environmental amenities (clean air, scenic views).
- Consumer Goods: Analyzing the pricing of electronics, appliances, and other products with diverse features.
3. Assumptions and Limitations:
While powerful, hedonic regression relies on several assumptions:
- Additivity: The value of the combined attributes equals the sum of the individual attribute values. This assumption might not always hold true, particularly when attributes interact in complex ways.
- No omitted variables: The model should include all relevant attributes affecting price. Omitted variables can lead to biased estimates.
- Linearity: The relationship between price and attributes is assumed to be linear. Non-linear relationships might require more sophisticated modeling techniques.
- Data quality: Accurate and reliable data are crucial for obtaining meaningful results. Measurement errors or inconsistencies in data can severely impact the accuracy of the estimates.
4. Advanced Techniques and Extensions:
Basic hedonic regression can be extended to address some of its limitations:
- Non-linear models: Allow for more flexible relationships between price and attributes.
- Interaction effects: Capture the influence of attribute combinations on price.
- Qualitative variables: Incorporate non-numerical attributes using dummy variables or other techniques.
- Spatial hedonic models: Account for spatial correlations in data, particularly important in real estate applications.
5. Impact on Innovation:
Hedonic regression contributes to innovation by providing valuable information for:
- Product development: Identifying features consumers value most, guiding design and development efforts.
- Pricing strategies: Optimizing prices based on the relative values of different attributes.
- Market analysis: Understanding consumer preferences and market trends.
Closing Insights: Summarizing the Core Discussion:
Hedonic regression is a sophisticated technique providing significant insights into how consumers value goods and services. While it presents limitations, its ability to decompose complex prices into individual attribute values makes it an indispensable tool for researchers and businesses across various fields. Addressing its limitations through advanced techniques enhances its precision and applicability.
Exploring the Connection Between Data Quality and Hedonic Regression:
The quality of the data used in hedonic regression is paramount. Inaccurate or incomplete data can lead to biased and unreliable results, undermining the entire analysis. This section explores the critical role of data quality in ensuring the validity and reliability of hedonic regression models.
Key Factors to Consider:
-
Roles and Real-World Examples: Illustrative examples showcasing how poor data quality leads to flawed estimations of attribute prices. For instance, inconsistent measurements of house sizes or inaccurate descriptions of amenities can significantly skew the results, leading to misinterpretations of consumer preferences.
-
Risks and Mitigations: Discussing potential risks associated with poor data quality, including biased coefficient estimates, inaccurate price predictions, and flawed conclusions about consumer behavior. Mitigation strategies involve thorough data cleaning, validation, and the implementation of robust data collection procedures.
-
Impact and Implications: Highlighting the consequences of using low-quality data. This might involve misallocation of resources by businesses based on faulty pricing models, incorrect policy decisions by governments based on flawed valuations of environmental goods, and misleading conclusions in academic research.
Conclusion: Reinforcing the Connection:
The connection between data quality and hedonic regression is undeniable. High-quality, accurate, and consistently measured data are essential for producing reliable and meaningful results. Failure to prioritize data quality can lead to significant errors in price estimations and flawed conclusions about consumer preferences, market dynamics, and policy implications.
Further Analysis: Examining Data Quality in Greater Detail:
This section will delve into specifics of ensuring data quality in hedonic regression. This includes discussing data sources, validation methods, handling missing data, outlier detection, and the use of data visualization techniques to identify potential problems.
FAQ Section: Answering Common Questions About Hedonic Regression:
-
Q: What is the difference between hedonic regression and other regression techniques?
- A: Hedonic regression specifically focuses on decomposing the price of a good into the values of its constituent attributes, unlike other regression models that might focus on different dependent variables or relationships.
-
Q: How can I handle qualitative variables in hedonic regression?
- A: Qualitative variables (e.g., color, brand) can be incorporated using dummy variables, creating binary indicators for each category.
-
Q: What are some common software packages used for hedonic regression?
- A: Statistical software like R, Stata, and SPSS are commonly used for performing hedonic regression.
-
Q: How can I interpret the coefficients from a hedonic regression model?
- A: The coefficients represent the marginal impact of each attribute on the price, holding other attributes constant. A positive coefficient indicates that an increase in the attribute increases the price.
-
Q: What are the limitations of hedonic regression?
- A: Limitations include the assumptions of additivity and linearity, the potential for omitted variables bias, and the need for high-quality data.
Practical Tips: Maximizing the Benefits of Hedonic Regression:
- Careful Data Collection: Ensure data is accurate, consistent, and complete.
- Variable Selection: Choose relevant and non-collinear attributes.
- Model Specification: Select appropriate functional forms to capture the relationship between price and attributes.
- Diagnostic Checks: Examine model diagnostics to assess the quality of the model.
- Interpretation: Carefully interpret the results, acknowledging the model's limitations.
Final Conclusion: Wrapping Up with Lasting Insights:
Hedonic regression, while possessing certain limitations, remains a powerful statistical tool for unraveling the complexities of price determination. Its ability to disentangle the implicit prices of individual characteristics offers invaluable insights across a range of disciplines. By carefully addressing its assumptions and limitations and prioritizing data quality, researchers and businesses can effectively leverage hedonic regression to gain a deeper understanding of consumer preferences, market dynamics, and the intricate relationship between happiness and price. The continued development and refinement of this technique promise even greater insights into the world of economic valuation in the years to come.
Latest Posts
Latest Posts
-
Hot Wallet Definition Types Examples And Safety Tips
Apr 24, 2025
-
Hot Money Definition
Apr 24, 2025
-
Hot Issue Definition
Apr 24, 2025
-
Hot Ipo Definition
Apr 24, 2025
-
Hostile Bid Definition
Apr 24, 2025
Related Post
Thank you for visiting our website which covers about Hedonic Regression Definition . We hope the information provided has been useful to you. Feel free to contact us if you have any questions or need further assistance. See you next time and don't miss to bookmark.