Garch Model Definition And Uses In Statistics
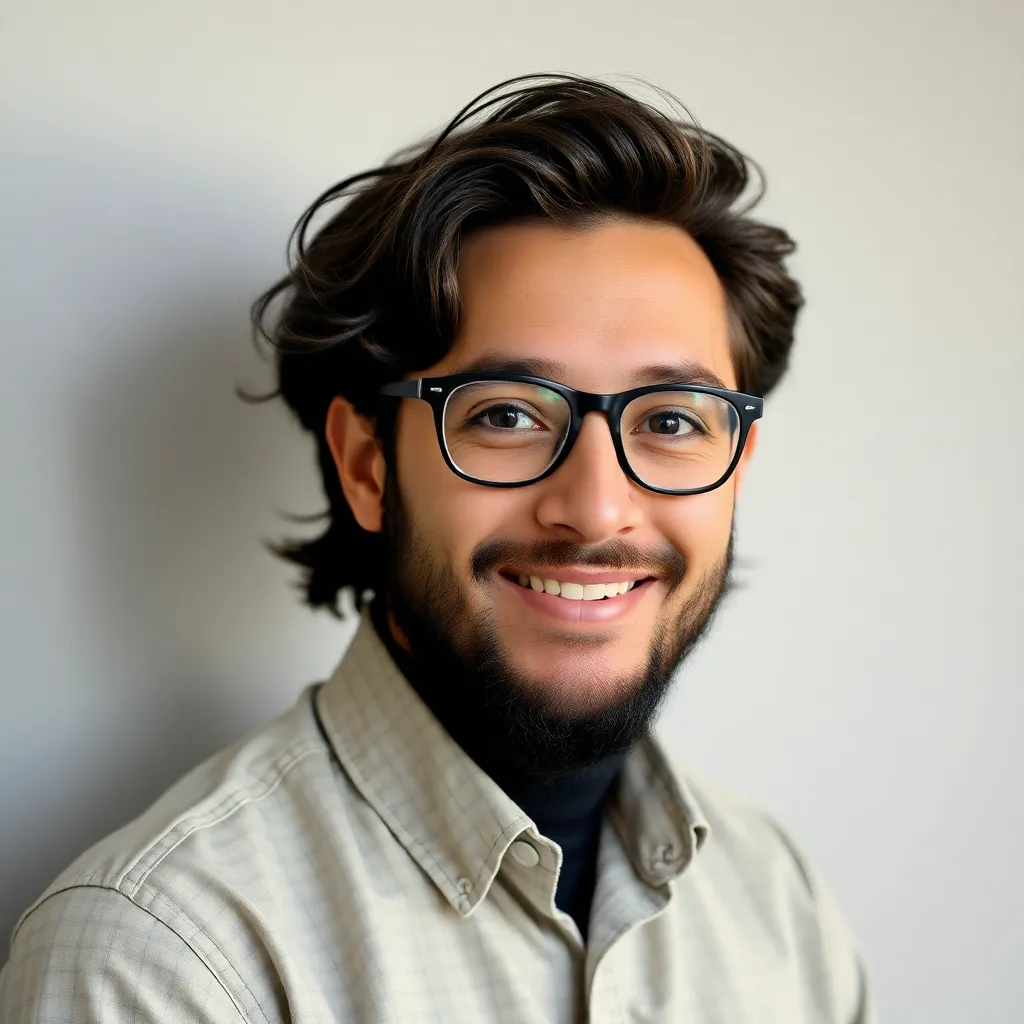
adminse
Apr 23, 2025 · 8 min read

Table of Contents
Unlocking Volatility: A Deep Dive into GARCH Models and Their Statistical Applications
What if accurately predicting market volatility was the key to unlocking superior investment strategies? Generalized Autoregressive Conditional Heteroskedasticity (GARCH) models offer a powerful statistical framework for precisely that, providing insights into the ever-changing nature of risk.
Editor’s Note: This article on GARCH models provides a comprehensive overview of their definition, underlying principles, and diverse applications in statistics, particularly within financial econometrics. Readers will gain a practical understanding of how GARCH models are used to model and forecast volatility, making it relevant for students, researchers, and practitioners alike.
Why GARCH Models Matter: Relevance, Practical Applications, and Industry Significance
GARCH models are indispensable tools in financial econometrics, offering a robust approach to modeling volatility clustering—the tendency for large price swings to be followed by more large swings, and small swings by more small swings. This phenomenon is pervasive in financial markets, making accurate volatility forecasting crucial for risk management, portfolio optimization, and option pricing. Beyond finance, GARCH models find applications in various fields dealing with time series data exhibiting volatility clustering, including meteorology (modeling rainfall intensity), hydrology (analyzing river flow), and even neuroscience (studying brainwave activity). The ability to forecast volatility allows for better decision-making across these disciplines.
Overview: What This Article Covers
This article provides a detailed exploration of GARCH models. We will delve into their definition, core concepts, various specifications (including GJR-GARCH and EGARCH), model estimation techniques, diagnostic testing, and practical applications across diverse fields. Readers will gain a strong understanding of how to interpret GARCH model outputs and apply them to real-world problems.
The Research and Effort Behind the Insights
This article is the result of extensive research, drawing upon seminal works in econometrics and financial time series analysis. Numerous academic papers, textbooks, and industry reports have been consulted to ensure accuracy and provide a comprehensive understanding of GARCH models. The explanations are grounded in statistical theory and supported by illustrative examples to enhance clarity and comprehension.
Key Takeaways:
- Definition and Core Concepts: A clear explanation of GARCH models and their underlying assumptions.
- Model Specifications: An overview of various GARCH model extensions, including GJR-GARCH and EGARCH.
- Model Estimation: A description of the techniques used to estimate GARCH model parameters.
- Diagnostic Testing: Methods for evaluating the adequacy and validity of estimated GARCH models.
- Practical Applications: Examples of GARCH model applications in finance and other fields.
- Limitations: Acknowledging the inherent limitations and potential pitfalls of GARCH models.
Smooth Transition to the Core Discussion:
With a foundation laid on the importance and scope of GARCH models, let's now delve into the core concepts, exploring their intricacies and applications in detail.
Exploring the Key Aspects of GARCH Models
1. Definition and Core Concepts:
At its core, a GARCH model is a statistical model used to analyze time series data with time-varying volatility. It extends the ARCH (Autoregressive Conditional Heteroskedasticity) model by incorporating past conditional variances into the current conditional variance equation. This captures the autoregressive nature of volatility clustering. The standard GARCH(p,q) model is defined as follows:
- Equation 1 (Conditional Mean Equation):
y_t = μ + ε_t
- Equation 2 (Conditional Variance Equation):
h_t = ω + α₁ε²_(t-1) + ... + α_qε²_(t-q) + β₁h_(t-1) + ... + β_ph_(t-p)
Where:
y_t
is the observed time series variable at time t.μ
is the unconditional mean of the series.ε_t
is the error term at time t, which is assumed to be normally distributed with mean 0 and conditional varianceh_t
.h_t
is the conditional variance ofε_t
at time t.ω
,αᵢ
, andβⱼ
are parameters to be estimated.ω
represents the long-run average variance.αᵢ
captures the impact of past squared errors on current volatility, andβⱼ
captures the impact of past conditional variances on current volatility. p and q represent the orders of the autoregressive components for the conditional variance.
2. Applications Across Industries:
The primary application of GARCH models lies in finance, where they are extensively used for:
- Volatility Forecasting: Predicting future volatility of asset returns, crucial for risk management.
- Value-at-Risk (VaR) Calculation: Estimating the potential loss in an investment portfolio over a specified time horizon and confidence level.
- Option Pricing: Incorporating volatility forecasts into option pricing models, like the Black-Scholes model, to obtain more accurate valuations.
- Portfolio Optimization: Developing optimal investment portfolios that balance risk and return, considering time-varying volatility.
- Risk Management: Implementing effective risk management strategies based on accurate volatility forecasts.
Beyond finance, GARCH models find applications in various fields:
- Meteorology: Modeling the variability of rainfall intensity.
- Hydrology: Analyzing the fluctuating nature of river flow.
- Epidemiology: Studying the volatility of disease outbreaks.
- Econometrics: Modeling the time-varying volatility of macroeconomic variables.
3. Challenges and Solutions:
While GARCH models are powerful, certain challenges need consideration:
- Model Selection: Choosing the appropriate order (p, q) for the GARCH model can be challenging, often requiring diagnostic tests and information criteria.
- Parameter Estimation: Estimating GARCH model parameters can be computationally intensive, particularly for higher-order models.
- Model Misspecification: If the underlying assumptions of the model are violated (e.g., non-normality of errors), the model's forecasts can be inaccurate.
- Data Requirements: GARCH models require relatively long time series data to obtain reliable parameter estimates.
4. Impact on Innovation:
GARCH models have significantly impacted financial innovation by providing more sophisticated tools for risk management and investment decision-making. Their ability to capture and forecast volatility has led to the development of more robust hedging strategies and improved portfolio allocation techniques. This has fostered greater market efficiency and reduced systemic risk.
Closing Insights: Summarizing the Core Discussion
GARCH models provide a fundamental framework for understanding and forecasting volatility in time series data. Their versatility and adaptability have made them an indispensable tool across diverse fields. While challenges exist, advancements in estimation techniques and model diagnostics continue to enhance their accuracy and reliability.
Exploring the Connection Between Leverage Effects and GARCH Models
The leverage effect describes the asymmetric relationship between asset returns and volatility: negative returns tend to increase volatility more than positive returns of the same magnitude. This asymmetry is often not captured by standard GARCH models.
Key Factors to Consider:
- Roles and Real-World Examples: The leverage effect is frequently observed in financial markets. A sharp market decline, for instance, generates heightened volatility, whereas a similar-sized increase might not have the same impact.
- Risks and Mitigations: Ignoring the leverage effect can lead to underestimated risk and inaccurate volatility forecasts. Addressing this requires models that explicitly account for asymmetry.
- Impact and Implications: Accurate modeling of the leverage effect is critical for risk management, option pricing, and portfolio optimization. Underestimating volatility due to neglecting asymmetry can lead to significant financial losses.
Conclusion: Reinforcing the Connection
The leverage effect significantly impacts the accuracy of GARCH models. Incorporating this asymmetry, as seen in extensions like GJR-GARCH and EGARCH, is crucial for obtaining more reliable volatility forecasts and better risk management.
Further Analysis: Examining GJR-GARCH and EGARCH in Greater Detail
GJR-GARCH (Glosten, Jagannathan, and Runkle GARCH): This model extends the standard GARCH model to account for the leverage effect by introducing a dummy variable that captures the impact of negative shocks.
EGARCH (Exponential GARCH): This model uses a logarithmic specification for the conditional variance, allowing for a more flexible representation of the leverage effect and potentially better capturing the dynamics of volatility. Its advantage lies in its ability to easily accommodate non-negative volatility.
FAQ Section: Answering Common Questions About GARCH Models
-
Q: What is the difference between ARCH and GARCH models? A: ARCH models only consider past squared errors to predict current volatility, while GARCH models also incorporate past conditional variances. GARCH is a more general and often more accurate model.
-
Q: How are GARCH model parameters estimated? A: Maximum Likelihood Estimation (MLE) is the most common method used to estimate GARCH model parameters.
-
Q: How can I assess the goodness of fit of a GARCH model? A: Diagnostic tests, such as Ljung-Box tests on the standardized residuals and squared standardized residuals, are used to check for remaining autocorrelation and ARCH effects. Information criteria (AIC, BIC) help compare different model specifications.
-
Q: What are the limitations of GARCH models? A: GARCH models assume that volatility is conditionally normally distributed, which may not always hold. They can also be sensitive to outliers and model misspecification. Furthermore, they are generally better suited for short-term forecasting.
Practical Tips: Maximizing the Benefits of GARCH Models
- Data Preprocessing: Ensure data is clean, stationary, and free from outliers.
- Model Selection: Employ diagnostic tests and information criteria to select the appropriate GARCH specification.
- Parameter Estimation: Use appropriate software (e.g., R, EViews, Stata) for accurate parameter estimation.
- Diagnostic Testing: Thoroughly check the model's assumptions and residuals for any remaining autocorrelation or ARCH effects.
- Forecasting Evaluation: Evaluate the accuracy of forecasts using appropriate metrics (e.g., RMSE, MAE).
Final Conclusion: Wrapping Up with Lasting Insights
GARCH models represent a significant advancement in statistical modeling of time-varying volatility. Their wide-ranging applications, particularly in finance, highlight their importance in risk management, investment strategies, and economic forecasting. While limitations exist, ongoing research and refinements continue to enhance their effectiveness and broaden their applicability across various disciplines. By understanding their strengths and limitations, researchers and practitioners can leverage the power of GARCH models to gain valuable insights from volatile time series data.
Latest Posts
Latest Posts
-
Gorilla Definition
Apr 23, 2025
-
Gordon Growth Model Ggm Defined Example And Formula
Apr 23, 2025
-
Goodwill Impairment Definition Examples Standards And Tests
Apr 23, 2025
-
Goods And Services Tax Gst Definition Types And How Its Calculated
Apr 23, 2025
-
Good Through Definition
Apr 23, 2025
Related Post
Thank you for visiting our website which covers about Garch Model Definition And Uses In Statistics . We hope the information provided has been useful to you. Feel free to contact us if you have any questions or need further assistance. See you next time and don't miss to bookmark.