Error Term Definition Example And How To Calculate With Formula
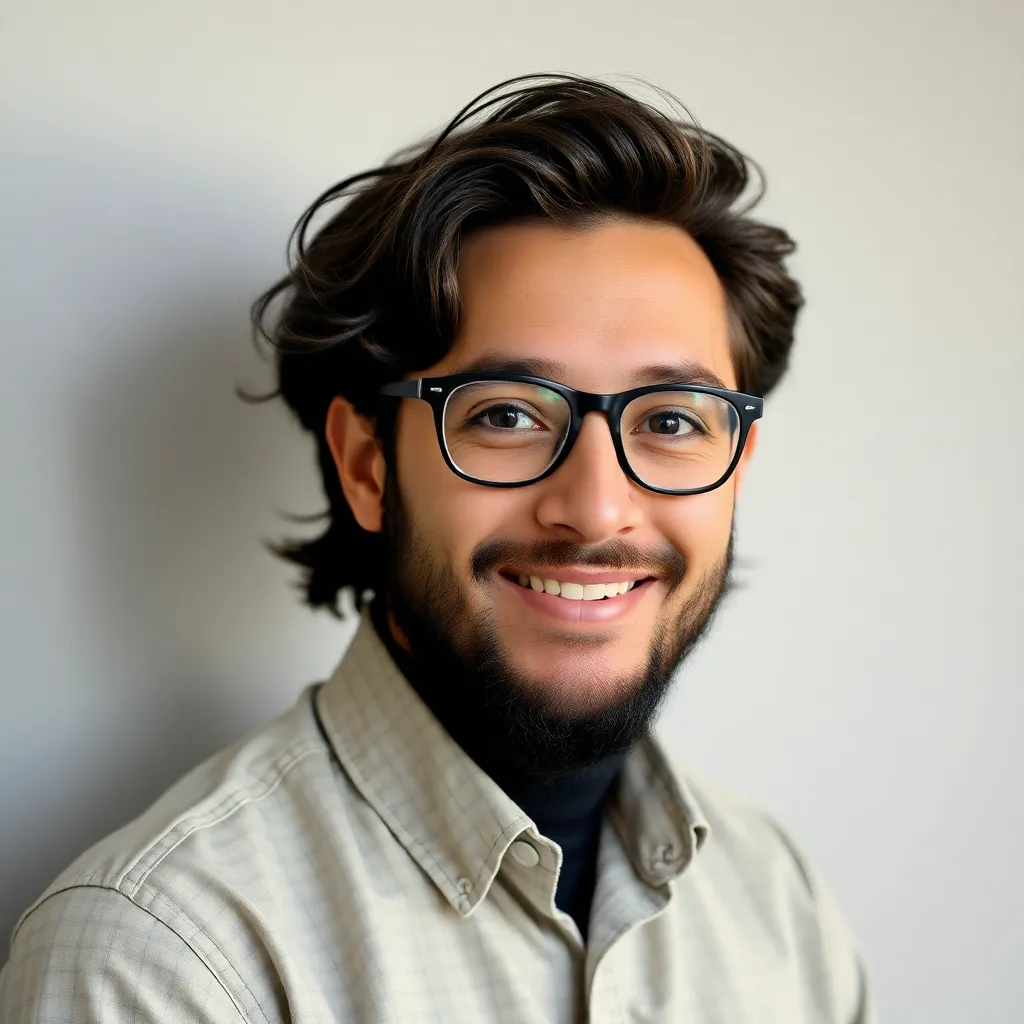
adminse
Apr 11, 2025 · 8 min read

Table of Contents
Unveiling the Enigma: Error Term Definition, Examples, and Calculation
What if the accuracy of our predictions hinges on understanding the elusive error term? This fundamental statistical concept underpins countless models, revealing the gap between reality and our estimations.
Editor's Note: This comprehensive article on the error term provides a detailed explanation, practical examples, and calculations. We aim to demystify this crucial statistical concept, making it accessible to both students and professionals.
Why the Error Term Matters: Relevance, Practical Applications, and Industry Significance
The error term, often denoted as ε (epsilon) or e, is a critical component of statistical models. It represents the difference between the observed value of a dependent variable and the value predicted by the model. Understanding and analyzing the error term is essential for several reasons:
- Model Accuracy: The magnitude and characteristics of the error term directly reflect the accuracy and predictive power of the model. Smaller, randomly distributed errors indicate a well-fitting model.
- Hypothesis Testing: Error terms are crucial in statistical hypothesis testing. They form the basis for calculating test statistics and assessing the significance of results.
- Model Improvement: Analyzing the error term can reveal deficiencies in the model, suggesting potential improvements through the inclusion of additional variables or adjustments to the model's structure.
- Forecasting and Prediction: Accurate estimation of the error term allows for more realistic predictions and forecasts, incorporating uncertainty inherent in the model.
- Across Industries: Applications span diverse fields including finance (risk assessment, portfolio management), economics (demand forecasting, econometric modeling), healthcare (disease prediction, treatment efficacy), and engineering (process optimization, quality control).
Overview: What This Article Covers
This article will systematically explore the error term, starting with its definition and progressing through various examples, showcasing calculation methods using relevant formulas, and discussing its implications in different statistical contexts. We will also analyze potential problems associated with error terms and methods to address them.
The Research and Effort Behind the Insights
This article draws upon established statistical literature, including textbooks, research papers, and online resources. Each concept is explained clearly, with illustrative examples and step-by-step calculations. The goal is to provide a comprehensive and accessible understanding of the error term.
Key Takeaways:
- Definition and Core Concepts: A precise definition of the error term and its fundamental role in statistical modeling.
- Types of Error Terms: Exploration of different types of error terms and their properties (e.g., homoscedasticity, normality).
- Calculating Error Terms: Step-by-step examples demonstrating the calculation of error terms in various models (e.g., simple linear regression, multiple linear regression).
- Interpreting Error Terms: Understanding the implications of different error term characteristics for model validity and interpretation.
- Addressing Error Term Issues: Strategies for handling problematic error terms (e.g., heteroscedasticity, autocorrelation).
Smooth Transition to the Core Discussion
Having established the importance of understanding the error term, let's delve into its core aspects.
Exploring the Key Aspects of the Error Term
1. Definition and Core Concepts:
The error term in a statistical model represents the difference between the actual observed value of the dependent variable (Y) and the value predicted by the model (Ŷ). Mathematically, it's expressed as:
ε = Y - Ŷ
Where:
- ε: The error term
- Y: The observed value of the dependent variable
- Ŷ: The predicted value of the dependent variable
2. Types of Error Terms and Their Properties:
Several assumptions are typically made about the error term in many statistical models. These assumptions are vital for the validity of statistical inferences. Key properties include:
- Zero Mean: The average of the error terms across all observations is zero, E(ε) = 0. This implies that the model, on average, correctly predicts the dependent variable.
- Constant Variance (Homoscedasticity): The variance of the error terms is constant across all levels of the independent variables. Violation of this assumption (heteroscedasticity) can lead to inefficient and biased estimates.
- Independence: The error terms are independent of each other. This means that the error in one observation does not influence the error in another observation. Violation (autocorrelation) is common in time series data.
- Normality: The error terms are normally distributed. This assumption is particularly important for hypothesis testing and confidence interval estimation.
3. Calculating Error Terms:
The calculation of the error term is straightforward. Once a model is fitted to the data, the predicted values (Ŷ) are generated. The error term for each observation is then calculated using the formula ε = Y - Ŷ.
Example: Simple Linear Regression
Let's consider a simple linear regression model: Y = β₀ + β₁X + ε
Where:
- Y: Dependent variable
- X: Independent variable
- β₀: Intercept
- β₁: Slope
- ε: Error term
Suppose we have the following data:
X | Y | Ŷ (Predicted Y) | ε (Error) |
---|---|---|---|
1 | 2 | 2.5 | -0.5 |
2 | 4 | 3.5 | 0.5 |
3 | 5 | 4.5 | 0.5 |
4 | 6 | 5.5 | 0.5 |
5 | 7 | 6.5 | 0.5 |
In this example, the predicted values (Ŷ) are calculated using the estimated regression equation. The error term (ε) is then calculated as the difference between the observed Y and the predicted Ŷ for each observation.
Example: Multiple Linear Regression
The calculation process extends to multiple linear regression models, involving multiple independent variables. The predicted values are obtained from the estimated regression equation, and the error terms are calculated as the difference between observed and predicted values, as before.
4. Interpreting Error Terms:
Analyzing the error terms provides valuable insights into the model's performance. Histograms, residual plots, and statistical tests can be used to assess the assumptions regarding the error terms (normality, homoscedasticity, independence). Large or systematically patterned errors suggest model inadequacies.
5. Addressing Error Term Issues:
If the assumptions regarding the error term are violated, several strategies can be employed to address these issues:
- Heteroscedasticity: Transforming the dependent variable (e.g., logarithmic transformation) or using weighted least squares regression.
- Autocorrelation: Using time series models that explicitly account for autocorrelation (e.g., ARIMA models) or including lagged variables in the regression.
- Non-normality: Transforming the dependent variable or using robust regression methods that are less sensitive to non-normality.
Exploring the Connection Between Residual Analysis and the Error Term
Residual analysis is a crucial aspect of evaluating the error term. Residuals are the observed values of the error term. By analyzing residuals, one can assess the validity of the underlying assumptions of a statistical model. This involves creating plots like residual plots (residuals vs. fitted values) and normal probability plots (to check normality). Outliers or patterns in the residuals can suggest problems with the model, such as violations of homoscedasticity or non-linearity.
Key Factors to Consider:
- Roles and Real-World Examples: Residual analysis in various models such as linear regression, ANOVA, and time series models reveal model fit and potential issues. For instance, a funnel-shaped residual plot points to heteroscedasticity in linear regression, while a cyclical pattern suggests autocorrelation in time series data.
- Risks and Mitigations: Failing to address problems revealed through residual analysis can lead to misleading conclusions from statistical tests and inaccurate predictions. Mitigations include transformation of variables, use of robust methods, and model respecification.
- Impact and Implications: Ignoring residual analysis can lead to incorrect inferences and unreliable results, jeopardizing decision-making processes in various fields.
Conclusion: Reinforcing the Connection
Residual analysis is intimately linked to understanding the error term. By carefully examining the residuals, we can gauge the validity of our model and address potential problems, leading to more accurate and reliable statistical inferences.
Further Analysis: Examining Residual Plots in Greater Detail
Residual plots are a visual representation of the error terms. By plotting residuals against fitted values or independent variables, potential issues like non-linearity, heteroscedasticity, and outliers become apparent. Detailed interpretation of these plots provides valuable information for improving model fit and accuracy.
FAQ Section: Answering Common Questions About the Error Term
- What is the difference between error and residual? While often used interchangeably, the error term is the true, unobservable difference between the observed value and the true population regression line, whereas the residual is the observable difference between the observed value and the estimated regression line.
- How does the error term affect hypothesis testing? The error term influences the standard error of the regression coefficients, impacting the t-statistics used in hypothesis tests. Violation of assumptions about the error term can lead to inaccurate p-values and incorrect conclusions.
- What are the consequences of ignoring error term assumptions? Ignoring assumptions can lead to inefficient or biased parameter estimates, invalid hypothesis tests, and unreliable predictions.
- How can I improve a model with problematic error terms? Depending on the specific issue, strategies include transforming variables, using weighted least squares, employing robust regression methods, or including additional variables to better explain the variation in the dependent variable.
Practical Tips: Maximizing the Benefits of Error Term Analysis
- Always inspect residuals: After model fitting, always create and examine residual plots.
- Understand the assumptions: Familiarize yourself with the key assumptions of the error term and their implications.
- Use appropriate diagnostic tests: Employ statistical tests to formally assess the assumptions of the error term (e.g., Breusch-Pagan test for heteroscedasticity, Durbin-Watson test for autocorrelation).
- Consider transformations: If assumptions are violated, explore data transformations to address these issues.
- Employ robust methods: When assumptions are severely violated, consider robust regression techniques less sensitive to deviations from assumptions.
Final Conclusion: Wrapping Up with Lasting Insights
The error term is not just a mathematical artifact; it is a crucial component of statistical models that reflects the inherent uncertainty and complexity in the data. By understanding its definition, properties, and implications, and through careful analysis of residuals, we can build more accurate, reliable, and robust statistical models, ultimately leading to improved decision-making and more valid conclusions. The journey to master statistical modeling begins with a thorough grasp of the often-overlooked, yet fundamentally important, error term.
Latest Posts
Latest Posts
-
Market Cycles Definition How They Work And Types
Apr 21, 2025
-
Market Conversion Price Definition
Apr 21, 2025
-
What Is Title 4 Funding
Apr 21, 2025
-
How To Get Funding To Flip A House
Apr 21, 2025
-
Market Capitalization Rule Definition
Apr 21, 2025
Related Post
Thank you for visiting our website which covers about Error Term Definition Example And How To Calculate With Formula . We hope the information provided has been useful to you. Feel free to contact us if you have any questions or need further assistance. See you next time and don't miss to bookmark.