Endogenous Variable Definition Meaning And Examples
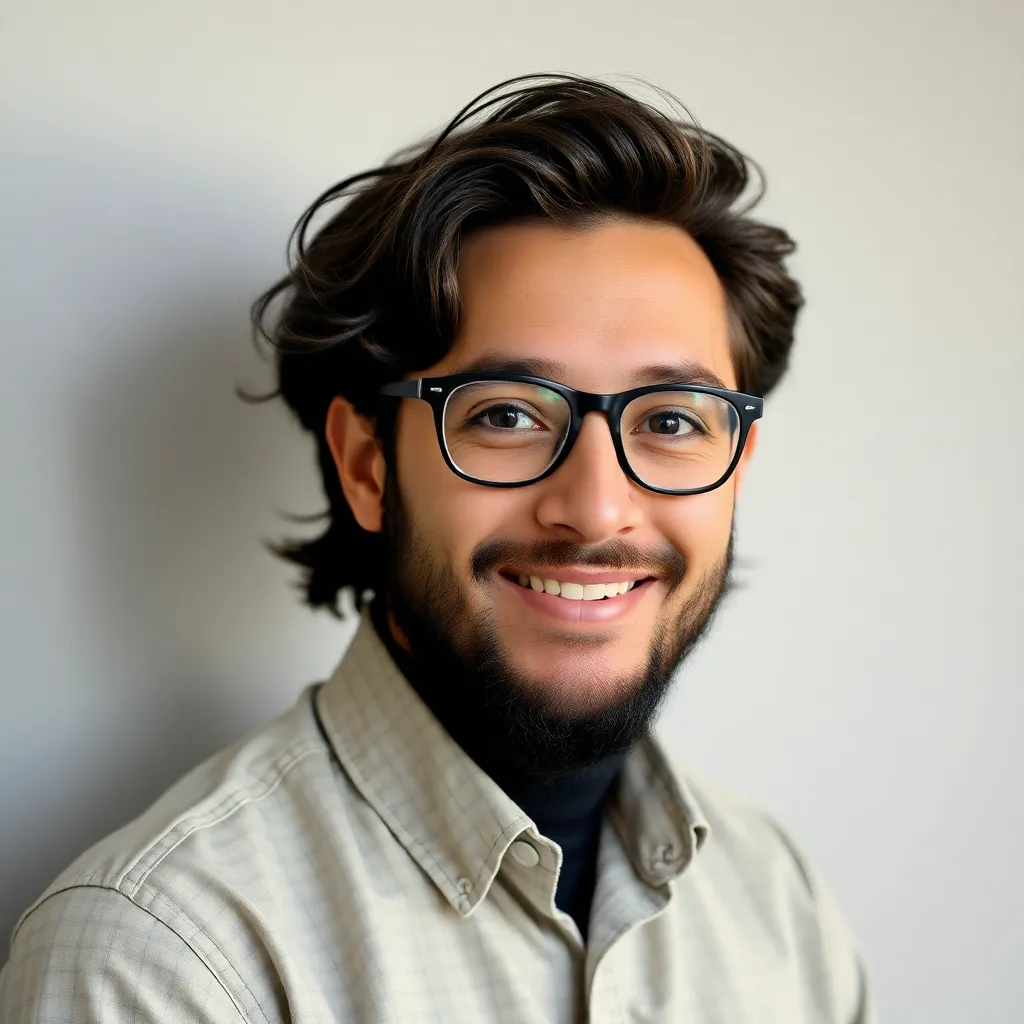
adminse
Apr 11, 2025 · 9 min read

Table of Contents
Understanding Endogenous Variables: Definition, Meaning, and Examples
What if the reliability of your research hinges on correctly identifying endogenous variables? Failing to account for endogeneity can lead to severely biased and misleading conclusions, undermining the validity of your entire study.
Editor’s Note: This comprehensive article on endogenous variables provides a detailed explanation of their definition, meaning, and implications for various research methodologies. We explore real-world examples across different fields to illuminate the concept and highlight the crucial role of appropriate statistical techniques in addressing endogeneity.
Why Endogenous Variables Matter:
Understanding endogenous variables is paramount for anyone conducting quantitative research, particularly in econometrics, statistics, and social sciences. An endogenous variable is one that is correlated with the error term in a statistical model. This correlation introduces bias into the estimation of the model's coefficients, leading to inaccurate inferences about the relationships between variables. Ignoring endogeneity can lead to flawed conclusions that misrepresent causal relationships and have significant implications for policy decisions, business strategies, and scientific understanding. The consequences of not addressing endogeneity can range from misinterpreting the effects of a treatment to making inaccurate predictions about future outcomes.
Overview: What This Article Covers:
This article will delve into the core concept of endogenous variables, providing a clear definition and exploring its meaning within the context of various statistical models. We will explore different sources of endogeneity, illustrate the problem with real-world examples, and discuss various methods used to mitigate the bias introduced by endogenous variables. Finally, we will provide practical tips to help researchers identify and address endogeneity in their own work.
The Research and Effort Behind the Insights:
This article is the result of extensive research, drawing upon established econometric texts, peer-reviewed journal articles, and online resources dedicated to statistical modeling and causal inference. The examples provided are carefully selected to represent diverse applications and illustrate the pervasiveness of endogeneity across various disciplines. Each section is supported by evidence and carefully explained to ensure readers receive accurate and readily understandable information.
Key Takeaways:
- Definition and Core Concepts: A precise definition of endogenous variables and a discussion of its core implications for statistical inference.
- Sources of Endogeneity: Examination of the various factors that can lead to endogeneity in a statistical model.
- Consequences of Ignoring Endogeneity: An exploration of the biases and inaccuracies that can result from neglecting endogeneity.
- Methods for Addressing Endogeneity: An overview of common techniques used to mitigate the effects of endogenous variables, including instrumental variables (IV) and control function approaches.
- Real-world Examples: Illustrative case studies from various fields demonstrating the practical implications of endogenous variables.
Smooth Transition to the Core Discussion:
Having established the importance of understanding endogenous variables, let's delve into a more detailed examination of its definition, sources, and implications.
Exploring the Key Aspects of Endogenous Variables:
1. Definition and Core Concepts:
An endogenous variable is a variable within a statistical model that is correlated with the error term. The error term represents unobserved factors that influence the dependent variable but are not included in the model. When an endogenous variable is correlated with the error term, it means that the observed relationship between the endogenous variable and the dependent variable is confounded by these unobserved factors. This correlation violates one of the key assumptions of ordinary least squares (OLS) regression, leading to biased and inconsistent estimates of the model's parameters. In simpler terms, it means the variable you are trying to explain is also influencing what you think is explaining it, creating a circular relationship.
2. Sources of Endogeneity:
Several factors can lead to endogeneity:
-
Omitted Variable Bias: This occurs when a relevant variable that influences both the independent and dependent variables is excluded from the model. The effect of the omitted variable is absorbed into the error term, leading to a correlation between the included independent variable and the error term.
-
Simultaneity Bias: This arises when there is a reciprocal causal relationship between the independent and dependent variables. Each variable influences the other, creating a feedback loop that makes it difficult to isolate the effect of one variable on the other.
-
Measurement Error: If the independent variable is measured with error, the error in measurement can be correlated with the true value of the variable. This correlation can be transferred to the error term of the model, resulting in endogeneity.
-
Sample Selection Bias: This occurs when the sample used for the analysis is not representative of the population of interest. If the selection process is related to the dependent variable, it can induce correlation between the independent variables and the error term.
3. Consequences of Ignoring Endogeneity:
Failing to address endogeneity leads to several serious problems:
-
Inconsistent Estimates: The estimated coefficients will not converge to their true values, even with an infinitely large sample size.
-
Biased Standard Errors: The standard errors of the estimated coefficients will be incorrect, leading to inaccurate hypothesis tests and confidence intervals.
-
Misleading Policy Implications: In policy analysis, ignoring endogeneity can lead to recommendations based on flawed causal inferences.
4. Methods for Addressing Endogeneity:
Several techniques exist to mitigate the problems caused by endogenous variables:
-
Instrumental Variables (IV) Regression: This technique involves finding an instrumental variable that is correlated with the endogenous variable but uncorrelated with the error term. The instrumental variable is used to create an estimate of the endogenous variable that is purged of its correlation with the error term.
-
Two-Stage Least Squares (2SLS): A specific application of IV regression commonly used in econometrics.
-
Control Function Approach: This method involves adding control variables to the model that capture the influence of the unobserved factors that are correlated with both the independent and dependent variables.
-
Panel Data Methods: Using panel data (observations on the same individuals or entities over multiple time periods) can help control for unobserved heterogeneity that could cause endogeneity.
5. Real-world Examples:
-
Economics: Estimating the effect of education on wages. Ability is an omitted variable that affects both education level and wages, leading to endogeneity. Using measures like aptitude tests as instrumental variables can help address this.
-
Marketing: Assessing the effectiveness of an advertising campaign. The decision to advertise heavily might be correlated with the inherent quality of a product (unobserved factor), creating endogeneity. A good instrumental variable might be the use of a randomized control trial.
-
Public Health: Evaluating the impact of a public health intervention on disease rates. Compliance with the intervention might be correlated with individual health behaviors (unobserved), leading to endogeneity. Randomized assignment of participants to intervention and control groups is often a solution here.
-
Political Science: Measuring the effect of campaign spending on election outcomes. The decision to invest heavily in campaign spending is often tied to factors like candidate quality, making it endogenous. Analysis might use past election results or other external factors as instrumental variables.
Exploring the Connection Between Omitted Variable Bias and Endogenous Variables:
Omitted variable bias is a primary source of endogeneity. It arises when a relevant variable that affects both the independent and dependent variables is left out of the model. For example, consider a study investigating the relationship between years of education and income. If "innate ability" is omitted, it creates a problem because individuals with higher innate ability are likely to obtain more education and earn higher incomes. The omitted variable (innate ability) is correlated with both education (independent variable) and income (dependent variable), leading to a spurious correlation between education and income in the model. This spurious correlation contaminates the estimate of the effect of education on income, making the independent variable endogenous.
Key Factors to Consider:
-
Roles and Real-World Examples: As illustrated in the examples above, omitted variables can significantly impact various fields. Ignoring omitted variables biases coefficient estimates.
-
Risks and Mitigations: Failing to address omitted variable bias leads to misleading conclusions and invalid policy recommendations. Including relevant control variables, using instrumental variables, or employing panel data methods are effective mitigations.
-
Impact and Implications: The effect of omitted variable bias can be substantial, underestimating or overestimating the true effect of the independent variable on the dependent variable.
Conclusion: Reinforcing the Connection:
The relationship between omitted variable bias and endogenous variables is crucial. Recognizing this connection is fundamental to understanding and addressing endogeneity in empirical research. By acknowledging the potential for omitted variables and employing appropriate statistical techniques, researchers can improve the accuracy and reliability of their findings.
Further Analysis: Examining Omitted Variable Bias in Greater Detail:
Omitted variable bias's severity depends on the strength of the correlation between the omitted variable and both the included independent variable and the dependent variable. A stronger correlation leads to more severe bias. The direction of the bias depends on the relationship between the omitted variable and the included independent variable, as well as the relationship between the omitted variable and the dependent variable. Understanding these relationships is critical in identifying and mitigating the bias.
FAQ Section: Answering Common Questions About Endogenous Variables:
-
What is the difference between an endogenous and an exogenous variable? An exogenous variable is one that is uncorrelated with the error term, while an endogenous variable is correlated with it. Exogenous variables are independent of the error term, while endogenous variables are not.
-
How can I identify an endogenous variable in my model? Several diagnostic tests exist, including the Hausman test and the Durbin-Wu-Hausman test. Visual inspection of scatterplots can also suggest the presence of endogeneity. The presence of omitted variable bias or simultaneous causality is strong indicators of endogeneity.
-
What are the most common methods for dealing with endogeneity? Instrumental variable (IV) regression and the control function approach are frequently employed. Panel data methods can also be effective.
-
Why is addressing endogeneity so important? Ignoring endogeneity leads to biased and inconsistent estimates, misleading inferences, and flawed policy recommendations.
Practical Tips: Maximizing the Understanding of Endogenous Variables:
-
Careful Model Specification: Begin by thoroughly considering all potential variables that might affect the dependent variable. Include as many relevant variables as possible.
-
Diagnostic Testing: Utilize statistical tests (like the Hausman test) to formally assess for endogeneity.
-
Instrumental Variable Strategy: If endogeneity is detected, consider employing an IV strategy. Finding a valid instrumental variable is critical.
-
Transparency and Reporting: Clearly document your approach to addressing endogeneity in your research. Report any diagnostic tests performed and explain your choice of method for handling endogeneity.
Final Conclusion: Wrapping Up with Lasting Insights:
Understanding endogenous variables is fundamental to conducting reliable quantitative research. The potential for bias resulting from endogeneity is substantial and can have far-reaching consequences. By employing appropriate methods and techniques, researchers can minimize the risks associated with endogeneity and improve the quality and credibility of their findings. The continuous development and application of advanced statistical methods underscore the ongoing importance of understanding and effectively addressing endogenous variables in various fields of study.
Latest Posts
Latest Posts
-
Boon Definition
Apr 19, 2025
-
Boom Definition In Economics And Finance Length Examples
Apr 19, 2025
-
What Is The Difference Between A 401k And A 401a
Apr 19, 2025
-
Boolean Algebra Definition
Apr 19, 2025
-
Boom And Bust Cycle Definition How It Works And History
Apr 19, 2025
Related Post
Thank you for visiting our website which covers about Endogenous Variable Definition Meaning And Examples . We hope the information provided has been useful to you. Feel free to contact us if you have any questions or need further assistance. See you next time and don't miss to bookmark.