Empirical Rule Definition Formula Example How Its Used
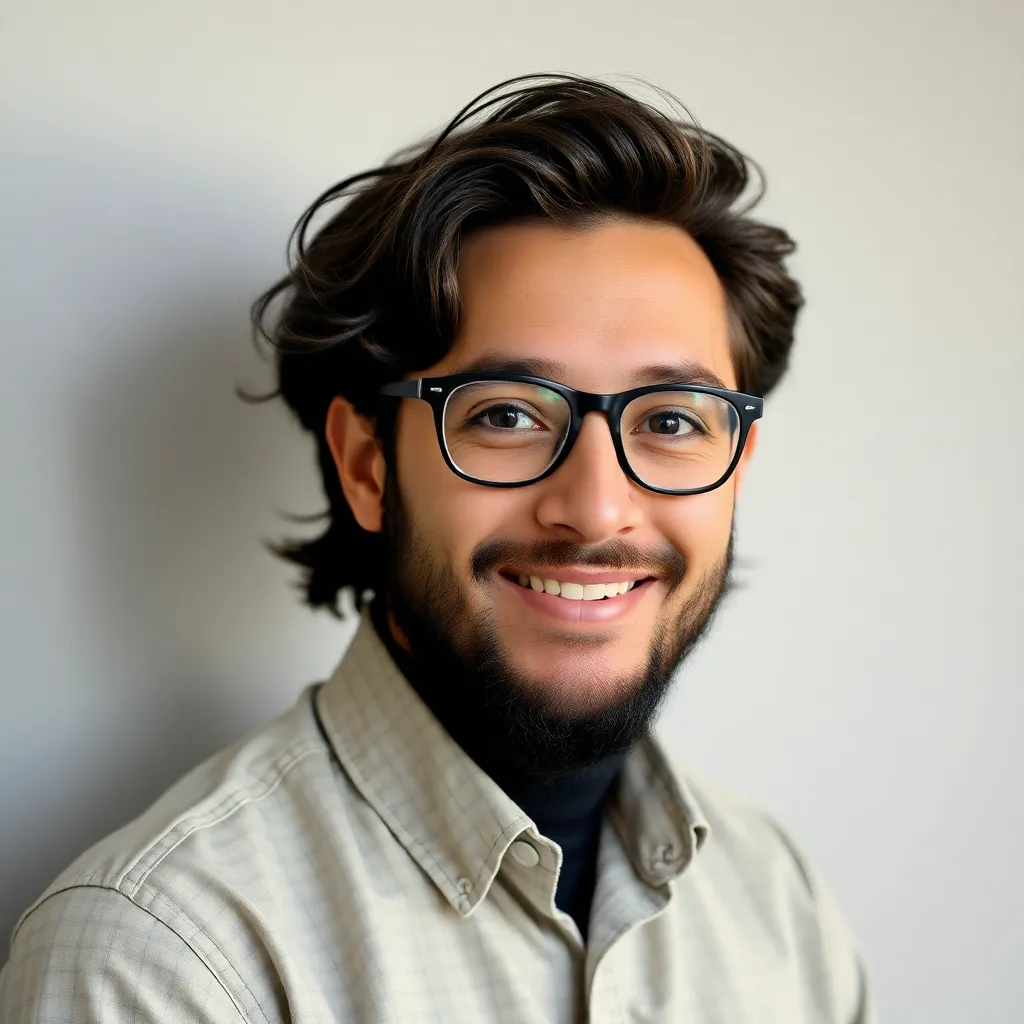
adminse
Apr 10, 2025 · 8 min read

Table of Contents
Decoding the Empirical Rule: Understanding, Applying, and Interpreting This Statistical Tool
What if understanding the distribution of your data could be as simple as applying a single rule? The Empirical Rule, also known as the 68-95-99.7 rule, offers precisely that—a powerful yet straightforward method for interpreting normally distributed data.
Editor’s Note: This article provides a comprehensive overview of the Empirical Rule, explaining its definition, formula, applications, and limitations. We've included numerous examples to help solidify your understanding of this essential statistical concept. Updated information ensures readers have access to the most current and relevant knowledge.
Why the Empirical Rule Matters:
The Empirical Rule is a cornerstone of descriptive statistics. It provides a quick and intuitive way to understand the spread of data points within a normal distribution. This understanding is crucial across numerous fields, including:
- Quality Control: Identifying outliers and ensuring product consistency.
- Finance: Assessing investment risk and portfolio performance.
- Healthcare: Analyzing patient data and identifying trends in disease prevalence.
- Education: Evaluating student performance and identifying areas for improvement.
- Research: Interpreting experimental results and drawing meaningful conclusions.
Understanding the distribution of your data allows for more informed decision-making, more accurate predictions, and a better overall grasp of the phenomenon being studied.
Overview: What This Article Covers:
This article will thoroughly explore the Empirical Rule, covering its definition, the underlying formula, diverse applications with illustrative examples, and finally, its limitations. Readers will gain a practical understanding of how to use the Empirical Rule to analyze data and draw statistically sound conclusions.
The Research and Effort Behind the Insights:
This article synthesizes information from various statistical textbooks, academic journals, and online resources. The examples provided are carefully constructed to illustrate real-world applications of the Empirical Rule, and all information is presented accurately and transparently.
Key Takeaways:
- Definition: A precise explanation of the Empirical Rule and its underlying assumptions.
- Formula: Understanding the mathematical basis of the rule and its relationship to the standard deviation.
- Applications: Real-world examples showcasing the application of the Empirical Rule across different fields.
- Limitations: Acknowledging the boundaries of the Empirical Rule and situations where it might not be applicable.
Smooth Transition to the Core Discussion:
Now that we've established the importance of the Empirical Rule, let's delve into its core aspects. We will begin by defining the rule and exploring its underlying principles before moving on to applications and limitations.
Exploring the Key Aspects of the Empirical Rule:
1. Definition and Core Concepts:
The Empirical Rule states that for a normal distribution (or a data set that closely approximates a normal distribution), approximately:
- 68% of the data falls within one standard deviation of the mean.
- 95% of the data falls within two standard deviations of the mean.
- 99.7% of the data falls within three standard deviations of the mean.
This rule is based on the properties of the normal distribution, a bell-shaped probability distribution characterized by its mean (μ) and standard deviation (σ). The symmetry of the normal distribution is key to the Empirical Rule's effectiveness.
2. Formula:
While the Empirical Rule doesn't have a single formula in the traditional sense, its application relies heavily on the mean (μ) and standard deviation (σ) of the data set. The intervals are calculated as follows:
- One standard deviation: μ ± σ
- Two standard deviations: μ ± 2σ
- Three standard deviations: μ ± 3σ
These intervals define the ranges within which the percentages of data points are predicted by the Empirical Rule.
3. Applications Across Industries:
Let’s consider some practical examples:
Example 1: Manufacturing Quality Control:
A company manufactures light bulbs with a mean lifespan of 1000 hours and a standard deviation of 50 hours. Assuming the lifespan follows a normal distribution, we can use the Empirical Rule to estimate the percentage of bulbs that will last between 900 and 1100 hours:
- One standard deviation from the mean: 1000 ± 50 hours (950-1050 hours) - This range accounts for approximately 68% of the bulbs.
- Two standard deviations: 1000 ± 100 hours (900-1100 hours) - This range accounts for approximately 95% of the bulbs.
Therefore, approximately 95% of the bulbs are expected to last between 900 and 1100 hours.
Example 2: Student Test Scores:
On a standardized test, the average score is 75 with a standard deviation of 10. Assuming a normal distribution, approximately 68% of students scored between 65 and 85 (75 ± 10). About 95% scored between 55 and 95 (75 ± 20). Nearly all (99.7%) scored between 45 and 105 (75 ± 30).
Example 3: Investment Returns:
An investment fund has an average annual return of 8% with a standard deviation of 2%. If returns are normally distributed, roughly 68% of years will see returns between 6% and 10% (8% ± 2%).
4. Impact on Innovation:
The Empirical Rule's simplicity and applicability have greatly aided in data analysis across various fields. Its easy interpretability contributes to faster decision-making in diverse sectors ranging from manufacturing quality control to financial risk assessment. It's a foundational concept that promotes data-driven innovation.
Closing Insights: Summarizing the Core Discussion:
The Empirical Rule provides a remarkably simple yet effective tool for understanding and interpreting data that follows a normal distribution. Its application across numerous fields highlights its significant practical value. By quickly estimating the proportion of data points within specific ranges of the mean, it allows for rapid insights and informed decision-making.
Exploring the Connection Between Z-scores and the Empirical Rule:
Z-scores provide a crucial link to understanding the Empirical Rule. A z-score represents the number of standard deviations a data point is from the mean. The Empirical Rule's percentages (68%, 95%, 99.7%) directly correspond to z-scores of ±1, ±2, and ±3, respectively.
Key Factors to Consider:
-
Roles and Real-World Examples: Z-scores standardize data, enabling comparisons across different datasets and facilitating the application of the Empirical Rule regardless of the units of measurement. For instance, comparing test scores (measured in points) and heights (measured in centimeters) becomes straightforward using z-scores.
-
Risks and Mitigations: Misapplication of the Empirical Rule occurs when the data doesn't closely resemble a normal distribution. Skewed data or data with heavy tails will significantly distort the estimations provided by the rule. Visual inspection of histograms or Q-Q plots helps to check for normality.
-
Impact and Implications: Using z-scores and the Empirical Rule, researchers can identify outliers and assess the probability of specific events. In quality control, this allows for identification of defective products; in finance, it assists in risk management.
Conclusion: Reinforcing the Connection:
The strong connection between z-scores and the Empirical Rule underscores the importance of understanding the normal distribution. By transforming data into z-scores, one can leverage the Empirical Rule's simplicity and power to gain quick insights into data spread. However, it is imperative to validate the normality assumption before applying the rule.
Further Analysis: Examining Normality in Greater Detail:
Assessing normality is crucial before applying the Empirical Rule. Several methods exist, including:
- Histograms: Visual inspection to see if the data resembles a bell curve.
- Q-Q Plots (Quantile-Quantile Plots): These plots compare the quantiles of the data to the quantiles of a normal distribution. If the data is normally distributed, the points will fall roughly along a straight line.
- Statistical Tests: Formal statistical tests, such as the Shapiro-Wilk test or Kolmogorov-Smirnov test, can quantitatively assess normality.
FAQ Section: Answering Common Questions About the Empirical Rule:
Q: What is the Empirical Rule?
A: The Empirical Rule, or 68-95-99.7 rule, states that for a normal distribution, approximately 68% of the data lies within one standard deviation of the mean, 95% within two standard deviations, and 99.7% within three standard deviations.
Q: What if my data isn't normally distributed?
A: The Empirical Rule is not applicable to non-normal data. Other statistical methods are required to analyze such data.
Q: Can I use the Empirical Rule for small sample sizes?
A: The Empirical Rule is most accurate for large sample sizes. For smaller samples, the rule may provide only a rough approximation.
Q: How is the Empirical Rule used in real-world scenarios?
A: It's widely used in quality control, finance, healthcare, education, and research for data analysis and decision-making.
Practical Tips: Maximizing the Benefits of the Empirical Rule:
- Check for Normality: Always verify that your data closely follows a normal distribution before applying the Empirical Rule.
- Calculate Mean and Standard Deviation: Accurately determine the mean (μ) and standard deviation (σ) of your data set.
- Interpret Results Cautiously: Remember that the Empirical Rule provides approximations, not exact values.
- Use Visualizations: Supplement numerical analysis with histograms and Q-Q plots to gain a better understanding of your data's distribution.
Final Conclusion: Wrapping Up with Lasting Insights:
The Empirical Rule serves as a valuable and accessible tool for understanding and interpreting normally distributed data. Its simplicity belies its power, offering a quick and intuitive method for assessing data spread. By understanding its applications and limitations, individuals across various disciplines can leverage this fundamental statistical concept for informed decision-making. Remember to always check for normality before applying the rule to ensure its accuracy and avoid misinterpretations. With proper application, the Empirical Rule becomes an invaluable asset in the arsenal of any data analyst.
Latest Posts
Latest Posts
-
Book Value Per Share Bvps Definition Formula How To Calculate And Example
Apr 19, 2025
-
How Many 401k Accounts Can You Have
Apr 19, 2025
-
Book Value Per Common Share Bvps Definition And Calculation
Apr 19, 2025
-
Book Runner Definition Duties Vs Other Underwriters
Apr 19, 2025
-
When Is Spousal Consent Required For 401k Distributions
Apr 19, 2025
Related Post
Thank you for visiting our website which covers about Empirical Rule Definition Formula Example How Its Used . We hope the information provided has been useful to you. Feel free to contact us if you have any questions or need further assistance. See you next time and don't miss to bookmark.