Definition Z Score
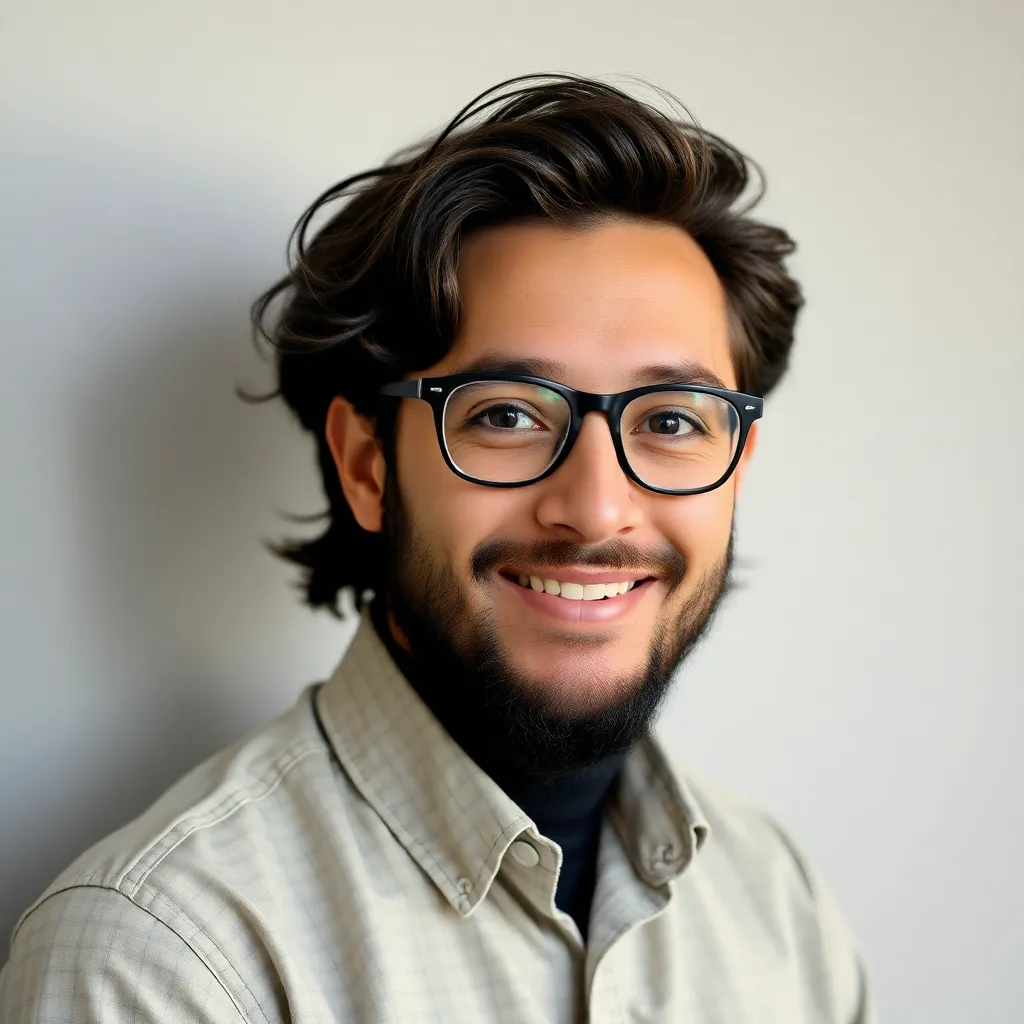
adminse
Apr 02, 2025 · 8 min read
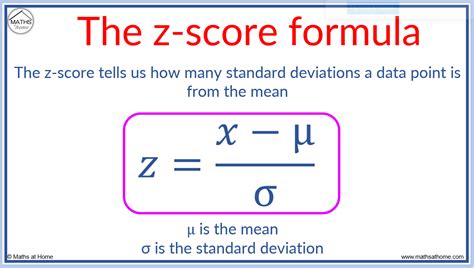
Table of Contents
Decoding the Z-Score: A Comprehensive Guide to Understanding and Applying This Powerful Statistical Tool
What if understanding the z-score unlocked a deeper understanding of data analysis and decision-making? This fundamental statistical concept is a cornerstone of numerous analytical techniques, offering valuable insights across diverse fields.
Editor’s Note: This article on the z-score provides a comprehensive explanation of this crucial statistical concept, offering practical examples and applications. Updated for accuracy and relevance, it serves as a valuable resource for students, researchers, and anyone seeking to improve their data analysis skills.
Why Z-Scores Matter: Relevance, Practical Applications, and Industry Significance
The z-score, also known as a standard score, is a fundamental concept in statistics with widespread applications across various disciplines. It measures how many standard deviations a data point is away from the mean of a data set. This seemingly simple calculation provides a powerful tool for:
- Data standardization: Comparing data points from different datasets with different units or scales.
- Outlier detection: Identifying unusual or extreme values that may warrant further investigation.
- Hypothesis testing: Evaluating the significance of research findings and drawing statistical inferences.
- Probability calculations: Determining the likelihood of observing a particular data point within a normally distributed dataset.
- Process control: Monitoring and improving the consistency and quality of manufacturing processes.
- Risk management: Assessing and managing financial risk by analyzing deviations from expected returns.
Its versatility makes it indispensable in fields like finance, healthcare, engineering, social sciences, and more. Understanding z-scores empowers better decision-making based on data-driven insights.
Overview: What This Article Covers
This article provides a comprehensive exploration of z-scores, encompassing their definition, calculation, interpretation, applications, and limitations. We will delve into the underlying principles, illustrate them with practical examples, and discuss their role in various statistical analyses. Readers will gain a solid understanding of how to calculate and interpret z-scores, enabling them to effectively utilize this powerful tool in their own work.
The Research and Effort Behind the Insights
This article is the result of extensive research, drawing upon established statistical textbooks, academic papers, and widely accepted statistical practices. Each concept is clearly explained, supported by illustrative examples, and presented in an accessible manner to ensure a thorough understanding.
Key Takeaways:
- Definition and Core Concepts: A precise definition of the z-score and its underlying principles.
- Calculation Methods: Step-by-step instructions on how to compute z-scores for individual data points and entire datasets.
- Interpretation of Z-Scores: Understanding the meaning of positive, negative, and large/small z-scores.
- Applications in Different Fields: Exploring diverse uses of z-scores across various disciplines.
- Limitations and Considerations: Acknowledging the situations where z-scores may not be appropriate.
Smooth Transition to the Core Discussion:
Having established the importance and scope of z-scores, let's now delve into the core concepts and calculations that define this powerful statistical tool.
Exploring the Key Aspects of Z-Scores
1. Definition and Core Concepts:
The z-score quantifies the distance between a data point and the mean of a dataset in terms of standard deviations. A positive z-score indicates that the data point lies above the mean, while a negative z-score indicates it lies below the mean. A z-score of zero means the data point is exactly equal to the mean. The magnitude of the z-score represents the distance from the mean in standard deviation units.
2. Calculation Methods:
The formula for calculating a z-score is:
Z = (X - μ) / σ
Where:
- Z = the z-score
- X = the individual data point
- μ = the population mean
- σ = the population standard deviation
If the population parameters (μ and σ) are unknown, sample statistics (x̄ and s) are used as estimates:
Z = (X - x̄) / s
Where:
- x̄ = the sample mean
- s = the sample standard deviation
Example:
Let's say a student scored 85 on a test where the class average (x̄) was 75 and the standard deviation (s) was 10. The z-score for this student's score would be:
Z = (85 - 75) / 10 = 1
This means the student's score is one standard deviation above the class average.
3. Interpretation of Z-Scores:
- Z = 0: The data point is equal to the mean.
- Z > 0: The data point is above the mean.
- Z < 0: The data point is below the mean.
- |Z| > 3: The data point is considered an outlier (often, but not always). The threshold for an outlier can vary depending on the context and the dataset's characteristics.
4. Applications Across Industries:
- Finance: Assessing investment risk, evaluating portfolio performance, detecting market anomalies.
- Healthcare: Monitoring patient vital signs, identifying at-risk patients, analyzing clinical trial data.
- Education: Comparing student performance across different classes or schools, evaluating the effectiveness of teaching methods.
- Manufacturing: Monitoring process variability, detecting defects, improving product quality.
5. Challenges and Solutions:
One major challenge is the assumption of normality. Z-scores rely on the data being approximately normally distributed. If the data is significantly skewed or non-normal, the interpretation of z-scores may be less reliable. Transformations like logarithmic or square root transformations can sometimes help address this issue. Another challenge arises when dealing with small sample sizes, where the sample standard deviation may not be a good estimate of the population standard deviation, leading to less accurate z-scores.
Impact on Innovation:
The z-score's simplicity and widespread applicability have fueled innovation in data analysis and decision-making across diverse fields. Its use in statistical software packages has made complex analyses more accessible, allowing researchers and practitioners to extract valuable insights from their data more efficiently.
Closing Insights: Summarizing the Core Discussion
The z-score is a versatile and powerful tool for understanding and interpreting data. Its ability to standardize data, detect outliers, and facilitate hypothesis testing makes it indispensable in numerous applications. However, it's crucial to remember the underlying assumptions and potential limitations to ensure accurate and meaningful interpretations.
Exploring the Connection Between Data Normalization and Z-Scores
Data normalization is a crucial preprocessing step in many data analysis tasks, often used to bring different variables onto a comparable scale. Z-score standardization is a specific type of data normalization. Understanding this connection is vital for applying z-scores effectively.
Key Factors to Consider:
-
Roles and Real-World Examples: Data normalization, including z-score standardization, ensures that variables with larger scales don't disproportionately influence the analysis. For example, in a dataset containing income and age, income might have a much larger range. Z-score normalization ensures both variables contribute equally to, say, a distance calculation or a machine learning algorithm.
-
Risks and Mitigations: Improper normalization can lead to distorted relationships between variables. Carefully considering the distribution of the data and choosing the appropriate normalization method (z-score normalization isn't always the best choice) is crucial.
-
Impact and Implications: By standardizing data, z-scores allow for fair comparisons, improved model performance, and more accurate interpretations of statistical results.
Conclusion: Reinforcing the Connection
The close link between data normalization and z-scores highlights the importance of preprocessing data before analysis. Z-score standardization, in particular, offers a powerful method for transforming data into a standard normal distribution, facilitating more robust and reliable statistical analyses.
Further Analysis: Examining Data Transformation Techniques in Greater Detail
Beyond z-score standardization, other data transformation techniques exist, such as min-max scaling, robust scaling, and logarithmic transformations. The choice of transformation depends on the specific characteristics of the data and the goals of the analysis. Understanding these different techniques is crucial for selecting the most appropriate approach for a given dataset.
FAQ Section: Answering Common Questions About Z-Scores
-
What is a z-score? A z-score is a numerical measure that describes a value's relationship to the mean of a group of values, measured in terms of standard deviations from the mean.
-
How is a z-score calculated? The z-score is calculated using the formula: Z = (X - μ) / σ (or Z = (X - x̄) / s for sample data).
-
What does a z-score of 2 mean? A z-score of 2 indicates that the data point is two standard deviations above the mean.
-
What is the significance of a negative z-score? A negative z-score indicates that the data point is below the mean.
-
Can z-scores be used with non-normal data? While z-scores are ideally used with normally distributed data, they can still be applied to non-normal data, but the interpretation may be less straightforward. Transformations may be needed.
-
What are the limitations of using z-scores? Limitations include the assumption of normality, sensitivity to outliers, and potential issues with small sample sizes.
Practical Tips: Maximizing the Benefits of Z-Scores
-
Understand the data: Before calculating z-scores, ensure you understand the distribution of your data and its characteristics.
-
Choose the right method: Determine whether to use population parameters or sample statistics in your calculations.
-
Interpret cautiously: Be aware of the limitations and assumptions involved in using z-scores.
-
Consider data transformations: If your data is non-normal, consider appropriate transformations before calculating z-scores.
-
Visualize your results: Create histograms or box plots to visualize the distribution of your data and the location of z-scores.
Final Conclusion: Wrapping Up with Lasting Insights
The z-score, a seemingly simple statistical concept, provides a powerful and versatile tool for data analysis. By understanding its calculation, interpretation, and limitations, researchers and practitioners can leverage its power to extract meaningful insights from their data, make informed decisions, and advance knowledge across various fields. Mastering the z-score is a key step towards becoming a more effective data analyst.
Latest Posts
Latest Posts
-
What Is Liquidity Mining Crypto
Apr 03, 2025
-
What Is The Meaning Of Liquidity Mining
Apr 03, 2025
-
Liquidity Mining Adalah
Apr 03, 2025
-
Is Liquidity Mining Profitable
Apr 03, 2025
-
Is Liquidity Mining Safe
Apr 03, 2025
Related Post
Thank you for visiting our website which covers about Definition Z Score . We hope the information provided has been useful to you. Feel free to contact us if you have any questions or need further assistance. See you next time and don't miss to bookmark.