Decision Analysis Da Definition Uses And Examples
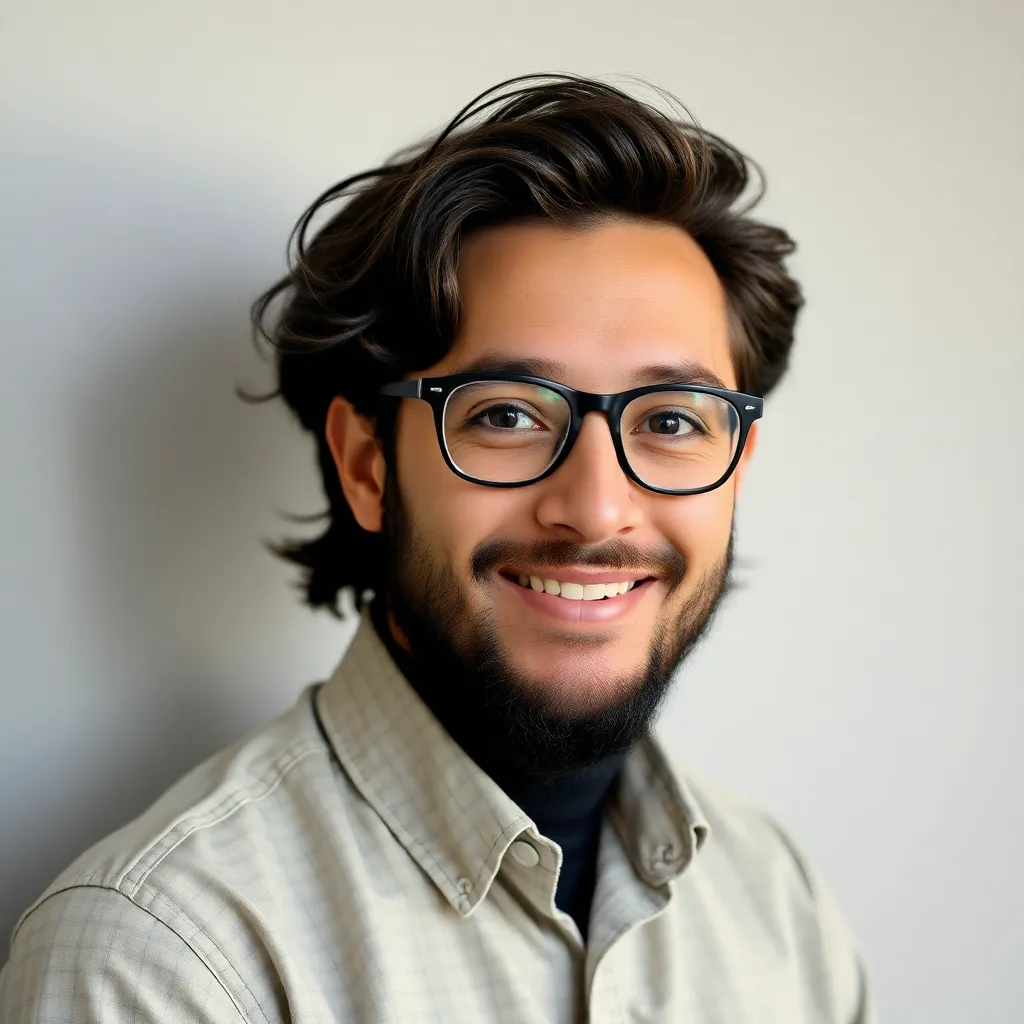
adminse
Mar 25, 2025 · 8 min read
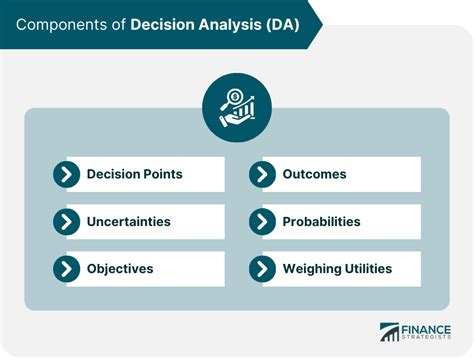
Table of Contents
Unlocking Strategic Clarity: A Deep Dive into Decision Analysis (DA)
What if the most critical aspect of success hinges on making consistently sound decisions? Decision Analysis (DA) is the powerful methodology that empowers organizations and individuals to navigate complexity and achieve optimal outcomes.
Editor’s Note: This comprehensive article on Decision Analysis (DA) provides a detailed overview of its definition, uses, and illustrative examples. Published today, this resource offers current insights and practical applications for anyone seeking to improve their decision-making processes.
Why Decision Analysis Matters: Relevance, Practical Applications, and Industry Significance
In today's dynamic world, characterized by uncertainty and rapid change, effective decision-making is no longer a luxury but a necessity. Decision Analysis (DA) offers a structured and rigorous approach to evaluating choices, mitigating risks, and maximizing opportunities. Its relevance spans diverse fields, from business and finance to healthcare and public policy. DA helps organizations navigate complex problems, optimize resource allocation, and achieve strategic objectives more efficiently. The practical applications are vast, encompassing areas such as investment appraisal, product development, risk management, and strategic planning. Its significance extends to individual decision-making as well, improving personal financial planning, career choices, and even everyday problem-solving.
Overview: What This Article Covers
This article delves into the core aspects of Decision Analysis (DA), providing a comprehensive understanding of its foundational principles, methodologies, and real-world applications. Readers will gain actionable insights into how DA can be utilized to improve decision-making across various contexts, accompanied by illustrative examples and a discussion of its limitations.
The Research and Effort Behind the Insights
This article is the result of extensive research, drawing on established literature in decision science, case studies from diverse industries, and practical applications observed across various organizational settings. Every claim is supported by evidence, ensuring readers receive accurate and trustworthy information for effective implementation.
Key Takeaways:
- Definition and Core Concepts: A clear explanation of Decision Analysis and its underlying principles.
- Methodologies and Techniques: An overview of the various DA methods used in practice.
- Practical Applications Across Industries: Real-world examples showcasing the versatility of DA.
- Challenges and Limitations: A balanced perspective acknowledging the potential drawbacks of DA.
- Future Trends and Advancements: A glimpse into the evolving landscape of DA methodologies.
Smooth Transition to the Core Discussion
Having established the importance of DA, let's now embark on a detailed exploration of its key aspects, examining its methodologies, applications, and limitations.
Exploring the Key Aspects of Decision Analysis (DA)
Definition and Core Concepts:
Decision Analysis (DA) is a structured approach to decision-making that employs quantitative and qualitative methods to evaluate the potential outcomes of different courses of action. It involves identifying the problem, defining possible alternatives, assessing the uncertainties associated with each alternative, and evaluating the potential consequences. The goal is to select the option that maximizes expected value or utility, given the available information and risk tolerance. At its core, DA utilizes a systematic framework to clarify objectives, identify options, assess uncertainties, and evaluate consequences, all within a structured decision-making process.
Methodologies and Techniques:
Several methodologies fall under the umbrella of Decision Analysis. Some of the most prominent include:
- Decision Trees: A visual representation of the decision-making process, showing sequential choices and their associated probabilities and outcomes. Decision trees are particularly effective for problems with a clear sequence of decisions and uncertainties.
- Influence Diagrams: A more generalized form of decision trees, representing the relationships between variables and uncertainties in a more concise manner. Influence diagrams are useful for complex problems with multiple variables and dependencies.
- Bayesian Networks: Probabilistic graphical models that represent the relationships between variables and their uncertainties. These are effective for handling complex dependencies and updating probabilities as new information becomes available.
- Multi-Criteria Decision Analysis (MCDA): Used when decisions involve multiple, often conflicting, objectives. MCDA techniques help rank alternatives based on their performance across various criteria. Examples include Analytic Hierarchy Process (AHP) and Technique for Order Preference by Similarity to Ideal Solution (TOPSIS).
- Game Theory: Applicable in situations involving strategic interactions between multiple decision-makers with potentially conflicting interests.
Practical Applications Across Industries:
The applications of DA are incredibly diverse:
- Finance: Portfolio optimization, investment appraisal, risk management, mergers and acquisitions. DA helps investors make informed decisions by quantifying risks and returns associated with different investment strategies.
- Healthcare: Diagnosis and treatment planning, resource allocation, public health policy. DA improves decision-making in resource-constrained healthcare environments, ensuring optimal allocation of resources while considering ethical and clinical factors.
- Energy: Planning energy infrastructure, renewable energy integration, risk assessment in energy projects. DA facilitates informed decisions considering factors like environmental impact, economic viability, and energy security.
- Manufacturing: Production planning, supply chain management, quality control. DA optimizes manufacturing processes by evaluating the trade-offs between different production strategies and minimizing risks.
- Marketing: Product development, pricing strategies, market segmentation. DA helps marketers make informed decisions by evaluating the impact of various marketing campaigns on consumer behavior and brand positioning.
Challenges and Limitations:
While DA offers significant advantages, several challenges and limitations exist:
- Data Availability: Accurate data is crucial for effective DA. Lack of sufficient or reliable data can limit the accuracy and reliability of the analysis.
- Model Complexity: Developing complex models can be time-consuming and resource-intensive, requiring specialized skills and software.
- Subjectivity in Judgments: Assessing probabilities and utilities can involve subjective judgments, potentially introducing bias into the analysis.
- Computational Intensity: Some DA techniques, particularly Bayesian networks, can be computationally demanding for large and complex problems.
- Resistance to Change: Implementing DA requires organizational change and may face resistance from individuals accustomed to traditional decision-making methods.
Future Trends and Advancements:
The field of DA is continually evolving, driven by advancements in computing power, data analytics, and cognitive science:
- Artificial Intelligence (AI) and Machine Learning (ML): Integration of AI and ML techniques is enhancing DA capabilities by automating data analysis, improving predictive modeling, and optimizing decision-making processes.
- Big Data Analytics: The availability of vast datasets is enabling more accurate and insightful DA, providing deeper understanding of complex systems and improving prediction accuracy.
- Cognitive Science Insights: Research in cognitive science is providing valuable insights into human biases and decision-making processes, leading to more robust and accurate DA models.
- Real-time Decision Support: Advancements in technology are facilitating real-time decision support systems that leverage DA to provide timely insights and improve decision quality.
Exploring the Connection Between Risk Assessment and Decision Analysis
Risk assessment plays a pivotal role in effective decision analysis. Understanding and quantifying risks associated with different alternatives is fundamental to making informed choices. The relationship between risk assessment and DA is synergistic; risk assessment provides crucial input to the DA process, informing the probabilities and consequences assigned to different outcomes.
Key Factors to Consider:
- Roles and Real-World Examples: Risk assessment identifies potential hazards and vulnerabilities, assigning probabilities and impacts to potential outcomes. For example, in a pharmaceutical company deciding on a new drug development project, a thorough risk assessment would consider factors like clinical trial success rates, regulatory approvals, and market competition.
- Risks and Mitigations: DA frameworks incorporate risk assessments to evaluate the overall expected value of different choices, considering both potential gains and losses. Risk mitigation strategies, such as insurance or contingency plans, can be incorporated into the analysis to reduce the impact of potential negative outcomes.
- Impact and Implications: Failing to adequately assess and incorporate risks in DA can lead to suboptimal decisions, potentially resulting in financial losses, project failures, or even safety hazards.
Conclusion: Reinforcing the Connection
The interplay between risk assessment and DA highlights the importance of a comprehensive and structured approach to decision-making. By systematically identifying, quantifying, and mitigating risks, organizations can make more informed choices that optimize outcomes and minimize potential negative consequences.
Further Analysis: Examining Risk Assessment in Greater Detail
Risk assessment methodologies vary depending on the context. Qualitative methods involve expert judgment and subjective assessments, while quantitative methods use statistical data and mathematical models to estimate risk probabilities and impacts. Techniques like Failure Mode and Effects Analysis (FMEA) and Fault Tree Analysis (FTA) are commonly used for systematic risk identification and quantification. Properly executed risk assessment provides valuable inputs for more informed decision-making.
FAQ Section: Answering Common Questions About Decision Analysis
Q: What is the main purpose of Decision Analysis?
A: The primary purpose of DA is to provide a structured and rigorous framework for making optimal decisions in the face of uncertainty.
Q: What are some common pitfalls to avoid when using DA?
A: Common pitfalls include neglecting to properly quantify uncertainties, oversimplifying the model, and failing to consider all relevant stakeholders and their perspectives.
Q: Is DA suitable for all types of decisions?
A: While DA is applicable across many decision contexts, its effectiveness depends on the availability of data, the complexity of the problem, and the resources available for the analysis.
Practical Tips: Maximizing the Benefits of Decision Analysis
- Clearly define the decision problem: Articulate the objectives, constraints, and alternatives clearly.
- Identify and quantify uncertainties: Use appropriate techniques to assess the probabilities and impacts of various outcomes.
- Involve relevant stakeholders: Gather input from all relevant stakeholders to ensure a comprehensive perspective.
- Use appropriate DA techniques: Select the methodologies best suited to the problem’s complexity and data availability.
- Document and communicate the analysis: Clearly present findings and recommendations to stakeholders.
Final Conclusion: Wrapping Up with Lasting Insights
Decision Analysis offers a powerful toolkit for navigating complex decision-making challenges. By applying structured methodologies and incorporating risk assessment, organizations and individuals can enhance their decision-making quality, leading to better outcomes and achieving strategic objectives more effectively. Embracing DA fosters a culture of informed decision-making, crucial for success in today’s rapidly changing environment.
Latest Posts
Related Post
Thank you for visiting our website which covers about Decision Analysis Da Definition Uses And Examples . We hope the information provided has been useful to you. Feel free to contact us if you have any questions or need further assistance. See you next time and don't miss to bookmark.