Crop Yield Definition Formula Statistics
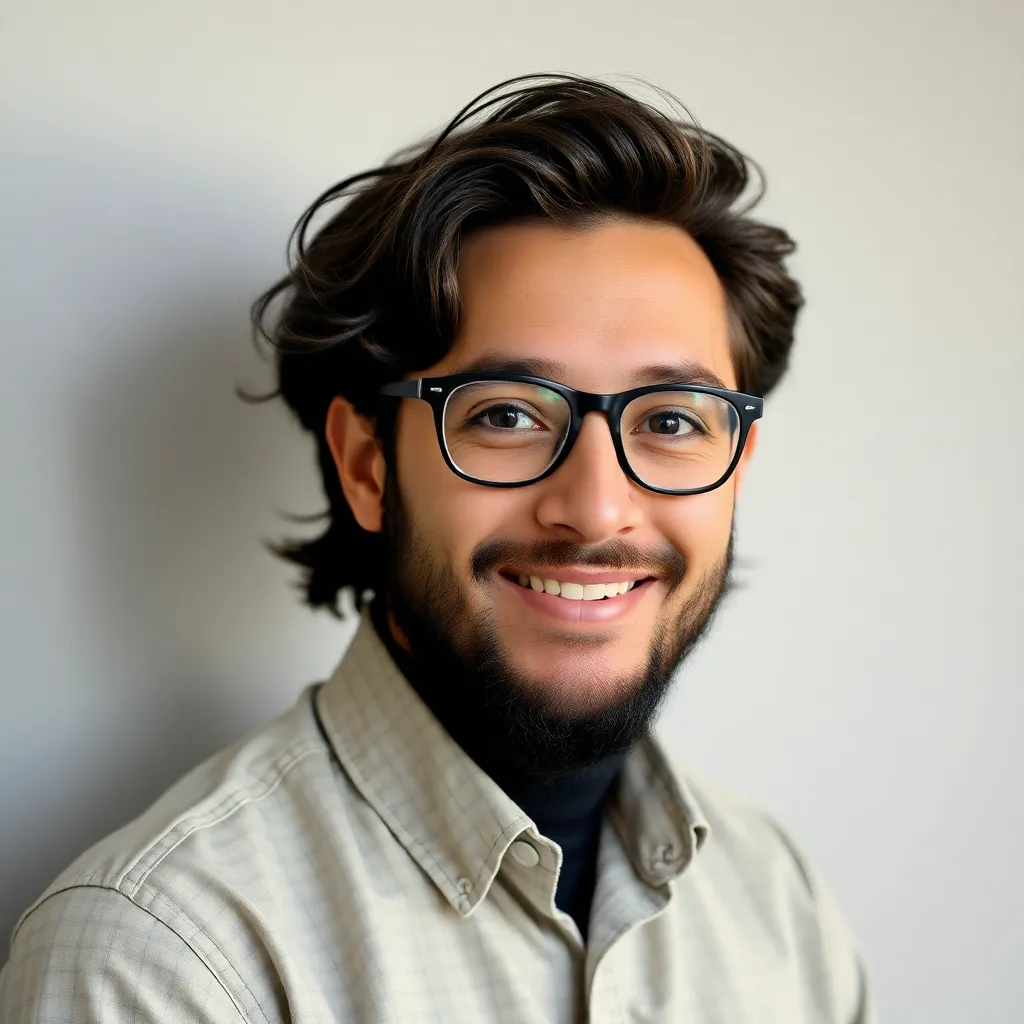
adminse
Mar 24, 2025 · 9 min read

Table of Contents
Unlocking the Secrets of Crop Yield: Definition, Formula, and Statistical Insights
What if understanding crop yield prediction could revolutionize food security? Mastering the complexities of crop yield—from its definition to advanced statistical modeling—is crucial for feeding a growing global population.
Editor’s Note: This article on crop yield definition, formula, and statistics was published today, providing readers with the most up-to-date information and analytical techniques in this vital field.
Why Crop Yield Matters: Relevance, Practical Applications, and Industry Significance
Crop yield, a seemingly simple concept, is a cornerstone of agricultural economics, food security, and environmental sustainability. It represents the efficiency of agricultural production, impacting everything from food prices and farmer incomes to national trade balances and global hunger. Understanding and optimizing crop yield is paramount for ensuring sufficient food supplies for a burgeoning global population, particularly in the face of climate change and resource scarcity. Accurate yield prediction allows for better resource allocation, informed policy decisions, and more efficient market functioning. The implications extend beyond immediate food production; efficient crop yields are intrinsically linked to environmental sustainability, minimizing land use, fertilizer application, and water consumption.
Overview: What This Article Covers
This article provides a comprehensive exploration of crop yield, encompassing its precise definition, calculation methods, and the statistical techniques employed to analyze and predict it. We delve into the various factors influencing yield, explore different statistical models, and discuss the challenges and future directions in this critical field. Readers will gain a nuanced understanding of the complexities involved and the potential for leveraging statistical analysis to improve agricultural practices and food security.
The Research and Effort Behind the Insights
This article is the result of extensive research, integrating information from peer-reviewed scientific journals, government agricultural reports, and industry publications. Data from various agricultural databases and statistical analyses have been employed to illustrate key concepts and support the arguments presented. The aim is to provide readers with a reliable and evidence-based understanding of crop yield and its related statistical analysis.
Key Takeaways:
- Definition and Core Concepts: A clear definition of crop yield, distinguishing between different types and units of measurement.
- Formula and Calculation Methods: A step-by-step guide on how to calculate crop yield, considering different scenarios and factors.
- Statistical Analysis Techniques: An overview of various statistical methods utilized in yield prediction and analysis, including regression analysis, time series analysis, and spatial statistics.
- Factors Influencing Crop Yield: An in-depth exploration of biotic and abiotic factors that impact crop yield, including climate, soil conditions, pests, and diseases.
- Challenges and Future Directions: Discussion of the limitations of current methods and the future potential of advanced technologies in crop yield prediction.
Smooth Transition to the Core Discussion
Having established the importance of understanding crop yield, let's now delve into its precise definition, the methodologies for its calculation, and the statistical approaches employed to analyze and predict it.
Exploring the Key Aspects of Crop Yield
1. Definition and Core Concepts:
Crop yield is defined as the amount of a harvested crop produced per unit area of land. It's typically expressed in units of weight (e.g., kilograms, bushels, tons) per unit area (e.g., hectare, acre). There are several ways to define and measure yield depending on the crop and the specific application. For example, grain yield might refer to the weight of the grain only, while total biomass yield includes the entire plant. Furthermore, yield can be expressed in terms of economic value (e.g., dollars per hectare) rather than weight.
2. Formula and Calculation Methods:
The basic formula for crop yield is:
Yield = Total harvested weight / Total cultivated area
However, this formula needs refinement depending on the specifics of the crop and the data available. For example, if the yield is calculated from a sample, adjustments might be necessary to extrapolate to the entire field. In such cases, the formula may involve sample size and area considerations. Furthermore, for crops with variable harvest components, separate yield calculations might be performed for each component (e.g., grain, straw, leaves).
3. Applications Across Industries:
Understanding crop yield is critical across several industries. Farmers use yield data to optimize their practices, while agricultural economists utilize it for market analysis and price forecasting. Government agencies rely on yield estimates for policy development and food security assessments. Insurance companies use it for risk assessment, and researchers employ it for developing improved crop varieties and agricultural techniques.
4. Challenges and Solutions:
Accurate crop yield prediction faces numerous challenges. The inherent variability in agricultural systems due to weather patterns, soil conditions, pests, and diseases makes precise prediction difficult. Data collection can also be problematic, particularly in developing countries where resources and infrastructure might be limited. Addressing these challenges involves implementing improved data collection methods, utilizing advanced statistical models, and incorporating remote sensing and other technological advancements.
5. Impact on Innovation:
Recent advancements in precision agriculture, including GPS technology, sensors, and data analytics, have greatly enhanced crop yield prediction and management. These technologies allow for site-specific management, enabling farmers to tailor their inputs (fertilizers, water, pesticides) to the specific needs of each section of their fields. This approach enhances efficiency and reduces environmental impact while increasing yields.
Closing Insights: Summarizing the Core Discussion
Accurate crop yield prediction is crucial for food security, economic stability, and sustainable agricultural practices. While challenges remain, advancements in technology and statistical modeling offer promising avenues for improving the accuracy and reliability of yield estimates. Understanding the complexities of crop yield is essential for optimizing agricultural production and ensuring a sufficient food supply for a growing global population.
Exploring the Connection Between Data Quality and Crop Yield Prediction
The relationship between data quality and crop yield prediction is pivotal. High-quality data is the bedrock of accurate and reliable yield forecasting. Poor data quality, on the other hand, can lead to inaccurate predictions, hindering effective decision-making in agriculture.
Key Factors to Consider:
Roles and Real-World Examples: High-quality data, encompassing accurate measurements of harvested weight and cultivated area, is essential for calculating crop yield. Inaccurate measurements due to faulty equipment or inconsistent recording practices will directly affect yield estimates. For instance, using an inaccurate weighing scale can lead to systematic underestimation or overestimation of yield.
Risks and Mitigations: The risks associated with poor data quality include flawed yield predictions, leading to suboptimal resource allocation (fertilizers, water, pesticides). Mitigation strategies include implementing standardized data collection protocols, investing in calibrated equipment, and utilizing quality control measures during data entry and analysis.
Impact and Implications: The impact of poor data quality on crop yield prediction cascades through the agricultural value chain. Inaccurate predictions can affect market prices, farmer incomes, and government policies related to food security and trade. The consequences can range from economic losses to food shortages.
Conclusion: Reinforcing the Connection
The interplay between data quality and crop yield prediction emphasizes the importance of robust data collection and management practices. Investing in improved data collection methods, quality control mechanisms, and training for data collectors is crucial for enhancing the accuracy and reliability of crop yield predictions, thereby supporting sustainable agricultural development and global food security.
Further Analysis: Examining Statistical Modeling Techniques in Greater Detail
Various statistical models are employed to analyze and predict crop yield. These models range from simple linear regression to complex machine learning algorithms.
Linear Regression: A basic yet valuable method, linear regression examines the linear relationship between yield and various predictor variables (e.g., rainfall, temperature, fertilizer application). This approach provides a straightforward way to understand the influence of different factors on yield.
Multiple Linear Regression: An extension of simple linear regression, this method allows for the simultaneous analysis of multiple predictor variables, providing a more comprehensive understanding of yield determinants.
Generalized Linear Models (GLMs): GLMs are suitable for handling non-normal data distributions, a common characteristic of yield data. They allow for the incorporation of various error distributions and link functions, offering greater flexibility in modeling yield.
Time Series Analysis: This approach focuses on analyzing yield data over time, identifying trends, seasonality, and other patterns. Time series models can be used for yield forecasting, considering past yield performance.
Spatial Statistics: Spatial statistics accounts for the spatial correlation in yield data, common in agricultural fields due to variations in soil conditions and microclimate. Spatial models can provide more accurate yield estimates by considering the spatial dependence among observations.
Machine Learning: Advanced machine learning techniques, such as neural networks and support vector machines, can handle complex relationships between yield and various predictors. These methods can capture non-linear relationships and improve the accuracy of yield forecasts.
FAQ Section: Answering Common Questions About Crop Yield
What is the difference between gross yield and net yield? Gross yield represents the total yield before any losses due to harvesting, post-harvest handling, or spoilage. Net yield considers these losses, reflecting the actual amount available for consumption or sale.
How can crop yield data be used for sustainable agriculture? Crop yield data, combined with environmental data and resource-use information, helps optimize inputs, reduce environmental impact, and promote efficient resource management.
What are the limitations of using statistical models for crop yield prediction? Statistical models rely on assumptions that may not always hold true in reality. Unforeseen events, such as extreme weather events or pest outbreaks, can significantly impact yield and are difficult to predict accurately.
Practical Tips: Maximizing the Benefits of Crop Yield Data
- Invest in accurate measurement tools: Accurate weighing scales, land surveying equipment, and GPS technology are crucial for obtaining reliable yield data.
- Implement standardized data collection protocols: Standardizing methods ensures consistency and comparability across different fields and time periods.
- Utilize appropriate statistical models: Selecting the correct model based on data characteristics and research questions is essential for accurate yield prediction.
- Integrate remote sensing data: Remote sensing provides valuable information on crop growth and health, enhancing the accuracy of yield forecasts.
- Regularly evaluate and refine models: Continuously evaluating model performance and updating models as new data become available improves prediction accuracy.
Final Conclusion: Wrapping Up with Lasting Insights
Crop yield, a multifaceted concept, represents a crucial aspect of agricultural productivity and global food security. Understanding its definition, calculation methods, and the application of statistical analysis is essential for improving agricultural practices, managing resources efficiently, and ensuring sufficient food supplies for a growing global population. By leveraging technological advancements and refined statistical modeling techniques, the agricultural sector can enhance its ability to predict crop yields accurately, leading to improved efficiency, reduced waste, and greater food security worldwide.
Latest Posts
Related Post
Thank you for visiting our website which covers about Crop Yield Definition Formula Statistics . We hope the information provided has been useful to you. Feel free to contact us if you have any questions or need further assistance. See you next time and don't miss to bookmark.