Conditional Value At Risk Cvar Definition Uses Formula
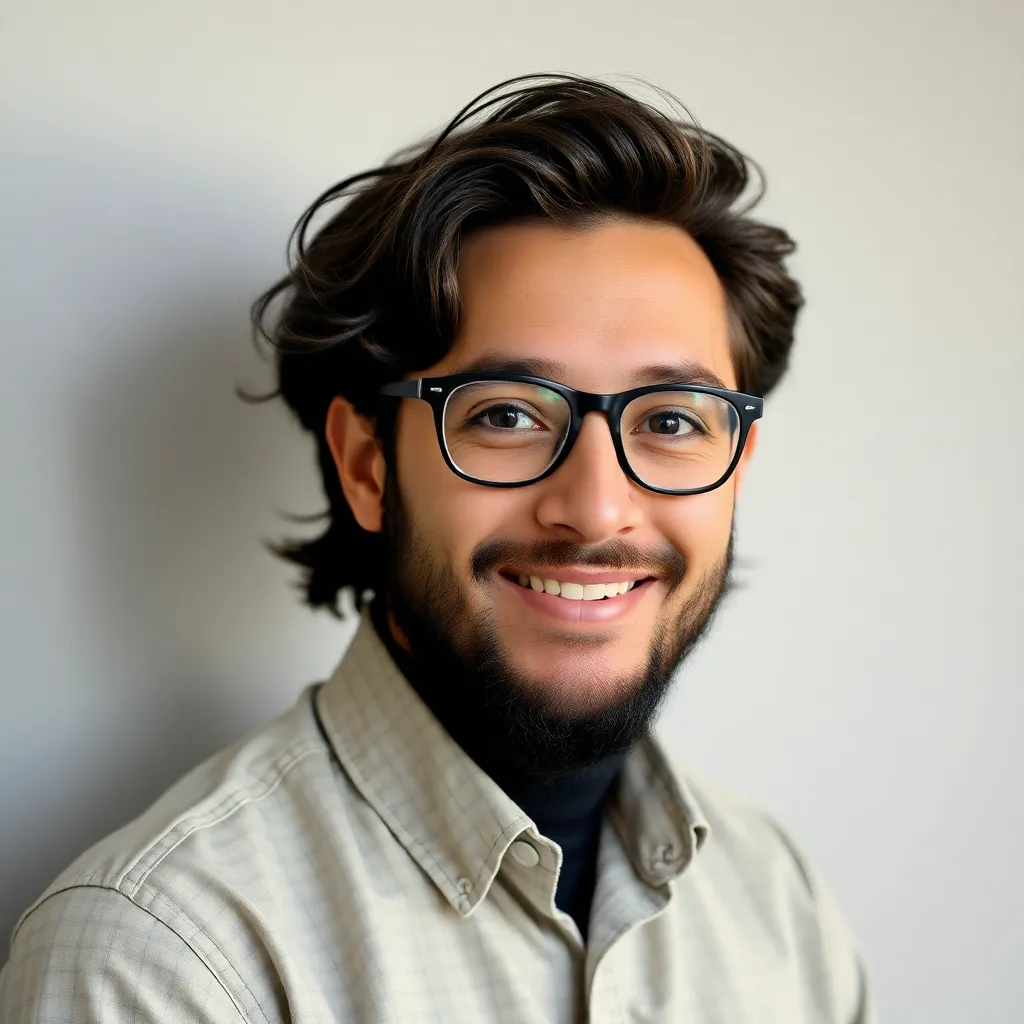
adminse
Mar 23, 2025 · 9 min read

Table of Contents
Unlocking the Power of Conditional Value at Risk (CVaR): Definition, Uses, and Formula
What if a more comprehensive risk measure could go beyond simply identifying potential losses? Conditional Value at Risk (CVaR), also known as Expected Shortfall (ES), offers precisely that – a deeper dive into the severity of tail risks.
Editor’s Note: This article on Conditional Value at Risk (CVaR) provides a comprehensive overview of its definition, practical uses, and mathematical formulation. It's designed to be accessible to both finance professionals and those seeking a deeper understanding of risk management. Updated [Date of Publication].
Why CVaR Matters: Relevance, Practical Applications, and Industry Significance
Value at Risk (VaR) is a widely used risk management metric, providing a quantifiable estimate of potential losses under normal market conditions at a given confidence level. However, VaR has limitations. It only tells us the magnitude of the loss at a specific percentile, neglecting the severity of losses beyond that point. This is where CVaR steps in. CVaR provides a more complete picture by considering the average of all losses exceeding the VaR threshold. This makes it particularly valuable in scenarios involving extreme market events or "tail risks," where large losses can have devastating consequences. Its applications span various financial sectors, including portfolio management, investment banking, and insurance. Regulatory bodies increasingly recognize CVaR as a superior alternative to VaR for assessing and mitigating risk.
Overview: What This Article Covers
This article delves into the core aspects of CVaR, starting with a precise definition and its mathematical formulation. We will then explore its practical applications across different financial instruments and asset classes. The article also addresses the challenges associated with CVaR calculation and its relationship to VaR. Finally, it will provide practical tips for effectively implementing CVaR in risk management strategies.
The Research and Effort Behind the Insights
This article is the result of extensive research, drawing upon academic literature in financial risk management, industry best practices, and regulatory guidelines. The formulas and explanations are grounded in established statistical and financial theory.
Key Takeaways:
- Definition and Core Concepts: A detailed explanation of CVaR and its relationship to VaR.
- Mathematical Formulation: Understanding the different methods for calculating CVaR.
- Practical Applications: Exploring the use of CVaR in portfolio optimization, risk budgeting, and stress testing.
- Limitations and Considerations: Addressing the challenges and assumptions associated with CVaR.
- Comparison to VaR: Highlighting the advantages and disadvantages of CVaR compared to VaR.
Smooth Transition to the Core Discussion:
Having established the importance of CVaR, let's now delve into its core components, exploring its mathematical foundation and practical applications in detail.
Exploring the Key Aspects of CVaR
1. Definition and Core Concepts:
CVaR, or Expected Shortfall (ES), is a coherent risk measure that quantifies the expected loss in the worst (1-α)% of cases. Unlike VaR, which only identifies the minimum loss at a given confidence level, CVaR considers the average of all losses exceeding that threshold. For example, a 95% CVaR of $1 million implies that the average loss in the worst 5% of scenarios is $1 million. This provides a more comprehensive and nuanced understanding of potential losses compared to the single-point estimate provided by VaR. The coherence property of CVaR ensures it satisfies desirable properties for a risk measure, including sub-additivity (meaning the risk of a portfolio is less than or equal to the sum of the risks of its individual components).
2. Mathematical Formulation:
The calculation of CVaR depends on the underlying distribution of portfolio returns. There are several methods for its computation:
-
Historical Simulation: This approach uses historical data to estimate the empirical distribution of returns. The CVaR is then calculated as the average of the returns falling below the VaR threshold. This method is simple but sensitive to the length and quality of the historical data.
-
Parametric Method: This method assumes a specific probability distribution for portfolio returns (e.g., Normal distribution). Using this assumption, the CVaR can be calculated analytically. While computationally efficient, this approach relies heavily on the accuracy of the assumed distribution.
-
Monte Carlo Simulation: This method involves generating a large number of random returns based on a given model. The CVaR is then estimated as the average of the simulated returns falling below the VaR threshold. This approach is flexible but computationally intensive.
The general formula for CVaR at a confidence level α is:
CVaR<sub>α</sub> = E[L | L > VaR<sub>α</sub>]
Where:
- CVaR<sub>α</sub> is the Conditional Value at Risk at confidence level α
- E[L | L > VaR<sub>α</sub>] is the expected value of the loss L, given that L exceeds the VaR at confidence level α
- VaR<sub>α</sub> is the Value at Risk at confidence level α
For a continuous distribution function F(x) with a probability density function f(x), the formula is:
CVaR<sub>α</sub> = (1/ (1 - α)) ∫<sub>VaRα</sub><sup>∞</sup> x * f(x) dx
3. Applications Across Industries:
CVaR finds broad applications across numerous industries:
-
Portfolio Management: CVaR is used to optimize portfolios by minimizing the expected shortfall at a given confidence level, leading to more robust investment strategies.
-
Risk Budgeting: Allocation of risk capital across different business units or portfolios can be managed more effectively using CVaR as a benchmark.
-
Stress Testing: CVaR is crucial in evaluating the potential impact of adverse market scenarios on a portfolio or financial institution.
-
Regulatory Compliance: Many regulatory bodies are increasingly using CVaR as a measure of risk for capital adequacy requirements.
-
Insurance and Reinsurance: CVaR provides a more accurate assessment of tail risks, particularly crucial in underwriting catastrophic events.
4. Challenges and Solutions:
Calculating CVaR presents several challenges:
-
Data Requirements: Accurate CVaR estimation necessitates a large and reliable dataset of historical returns. Insufficient data can lead to unreliable estimates.
-
Computational Complexity: Especially for Monte Carlo simulations, computing CVaR can be computationally intensive, requiring significant processing power.
-
Model Risk: The choice of the probability distribution (parametric method) or the model generating random returns (Monte Carlo) can introduce biases and errors.
Solutions to address these challenges include:
- Robust estimation techniques: Employing methods that are less sensitive to outliers and data noise.
- Advanced computational techniques: Leveraging parallel computing and efficient algorithms.
- Model validation: Rigorous testing and validation of the chosen model to ensure its accuracy.
5. Impact on Innovation:
The use of CVaR has driven innovation in risk management, leading to the development of more sophisticated risk models and management tools. It’s fostered research into improved estimation techniques and the integration of CVaR into broader risk frameworks.
Closing Insights: Summarizing the Core Discussion
CVaR is a powerful tool for assessing and managing tail risks, offering a more comprehensive and nuanced approach than traditional VaR. Its ability to capture the average severity of losses in extreme scenarios makes it particularly valuable in situations where large losses can have significant consequences. While challenges exist in its calculation and interpretation, ongoing advancements in computational techniques and statistical methods are continually improving its applicability and accuracy.
Exploring the Connection Between VaR and CVaR
The relationship between VaR and CVaR is fundamental. VaR identifies the minimum loss at a given confidence level, while CVaR provides the expected loss exceeding that threshold. They are intrinsically linked; CVaR considers the entire tail beyond the VaR point, providing a more complete picture of risk.
Key Factors to Consider:
-
Roles and Real-World Examples: In portfolio optimization, VaR might be used as a constraint, while CVaR would be the objective function to minimize. For example, a portfolio manager might aim to minimize the expected shortfall (CVaR) subject to a VaR constraint.
-
Risks and Mitigations: The primary risk associated with both VaR and CVaR is model risk – the risk that the chosen model doesn't accurately represent the underlying distribution of returns. Mitigation strategies include rigorous model validation, sensitivity analysis, and using multiple models for comparison.
-
Impact and Implications: The adoption of CVaR has significant implications for regulatory compliance, portfolio construction, and risk management practices overall. It shifts the focus from simply identifying potential losses to understanding the severity of those losses.
Conclusion: Reinforcing the Connection
VaR and CVaR work in tandem. VaR sets the stage, identifying the point beyond which extreme losses occur; CVaR then quantifies the average magnitude of those losses. Understanding this relationship is key to effective risk management.
Further Analysis: Examining Parametric Methods in Greater Detail
Parametric methods for CVaR calculation assume a specific probability distribution for portfolio returns. The most common assumption is a normal distribution, which simplifies the calculation considerably. However, financial returns often exhibit fat tails and skewness, making the normal distribution a potentially inaccurate assumption. More sophisticated parametric methods utilize distributions like the Student's t-distribution or skewed distributions to better capture the characteristics of financial returns. The choice of distribution is crucial and should be carefully justified based on the specific asset class and data characteristics.
FAQ Section: Answering Common Questions About CVaR
-
What is CVaR? CVaR, or Expected Shortfall, is a risk measure that calculates the expected loss in the worst (1-α)% of cases, providing a more comprehensive picture of potential losses than VaR.
-
How is CVaR different from VaR? VaR only specifies the minimum loss at a given confidence level, while CVaR considers the average loss exceeding that threshold.
-
How is CVaR calculated? CVaR can be calculated using historical simulation, parametric methods (assuming a specific distribution), or Monte Carlo simulation.
-
What are the limitations of CVaR? CVaR calculations rely on accurate data, appropriate model selection, and can be computationally intensive.
-
How is CVaR used in practice? CVaR is used in portfolio optimization, risk budgeting, stress testing, and regulatory compliance.
Practical Tips: Maximizing the Benefits of CVaR
-
Understand the Basics: Begin with a solid grasp of the definition, calculations, and interpretations of CVaR.
-
Choose the Appropriate Method: Select the calculation method best suited to your data and computational capabilities (historical simulation, parametric method, or Monte Carlo simulation).
-
Validate Your Model: Thoroughly test and validate your chosen model to ensure accuracy and reliability.
-
Consider Data Quality: Use high-quality, reliable data to achieve accurate CVaR estimates.
-
Interpret Results Carefully: Understand the limitations of CVaR and avoid over-reliance on a single risk measure.
Final Conclusion: Wrapping Up with Lasting Insights
Conditional Value at Risk (CVaR) is a valuable tool for improving risk management strategies. By providing a more comprehensive assessment of tail risks than traditional VaR, CVaR empowers financial institutions and investors to make more informed decisions. While computational complexity and model risk require careful consideration, the benefits of incorporating CVaR into risk assessment frameworks significantly outweigh the challenges. The continued refinement of CVaR methodologies and their integration with other risk metrics will undoubtedly play a crucial role in shaping the future of risk management.
Latest Posts
Related Post
Thank you for visiting our website which covers about Conditional Value At Risk Cvar Definition Uses Formula . We hope the information provided has been useful to you. Feel free to contact us if you have any questions or need further assistance. See you next time and don't miss to bookmark.