Chain Ladder Method Clm Definition Steps To Apply It
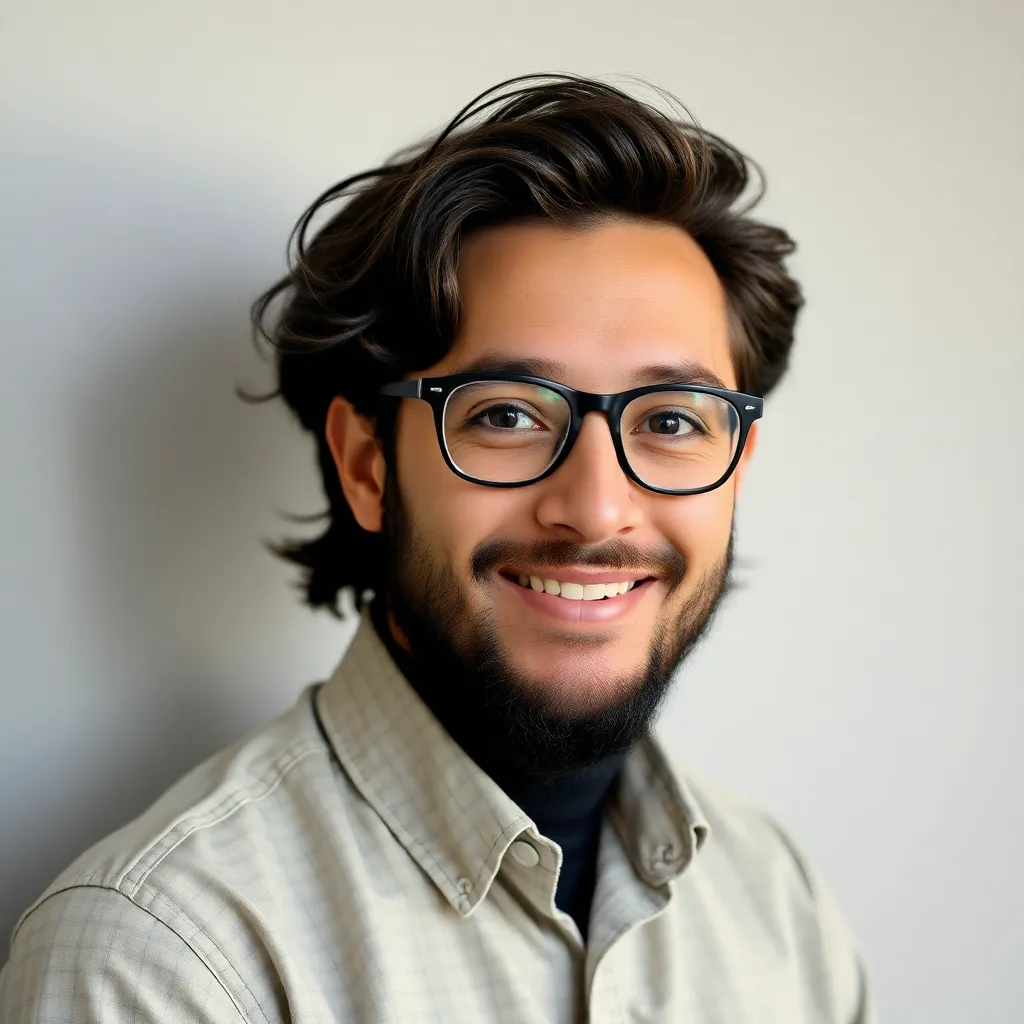
adminse
Mar 12, 2025 · 9 min read

Table of Contents
Unlocking the Future of Loss Reserving: A Deep Dive into the Chain Ladder Method (CLM)
What if accurately predicting future insurance claims could revolutionize risk management? The Chain Ladder Method (CLM), a powerful statistical technique, provides just that, offering crucial insights into outstanding claims and improving financial stability.
Editor’s Note: This article on the Chain Ladder Method (CLM) provides a comprehensive overview of its definition, application steps, and limitations. It's designed to equip readers with a practical understanding of this vital loss reserving technique.
Why the Chain Ladder Method Matters:
The Chain Ladder Method (CLM) is a cornerstone of actuarial science, used extensively in the insurance industry to estimate the ultimate cost of claims. Accurate loss reserving is paramount for insurers, enabling them to:
- Set appropriate premiums: By accurately predicting future claims, insurers can price their policies effectively, ensuring profitability and sustainability.
- Manage capital effectively: Knowing the likely future claims burden allows for better capital allocation and strategic financial planning.
- Meet regulatory requirements: Many regulatory bodies require insurers to maintain sufficient reserves to cover future claims. The CLM helps meet these obligations.
- Enhance financial reporting: Accurate loss reserves contribute to more transparent and reliable financial statements, improving investor confidence.
- Improve risk management: By understanding the patterns and trends in claims development, insurers can proactively identify and mitigate potential risks.
Overview: What This Article Covers:
This article will thoroughly dissect the Chain Ladder Method (CLM), covering its fundamental principles, step-by-step application, underlying assumptions, limitations, and potential refinements. We will explore its practical applications and discuss how to interpret the results effectively.
The Research and Effort Behind the Insights:
This article draws upon extensive research, combining established actuarial literature with practical examples to ensure clarity and understanding. The methodologies presented are widely accepted within the actuarial community, providing a robust framework for loss reserving.
Key Takeaways:
- Definition and Core Concepts: A clear understanding of the CLM’s fundamental principles.
- Step-by-Step Application: A practical guide on how to implement the CLM using readily available data.
- Assumptions and Limitations: A critical analysis of the CLM's underlying assumptions and their potential impact.
- Interpreting Results: Guidance on extracting meaningful insights from the CLM’s output.
- Advanced Considerations: An introduction to refinements and extensions of the basic CLM.
Smooth Transition to the Core Discussion:
Now that we understand the importance of the CLM, let's delve into the specifics of its methodology and application.
Exploring the Key Aspects of the Chain Ladder Method (CLM):
1. Definition and Core Concepts:
The Chain Ladder Method is a widely used actuarial technique for estimating the ultimate incurred losses for insurance claims. It relies on the analysis of historical claims data to project the development of outstanding claims over time. The method assumes that the pattern of claims development observed in the past will continue into the future. This pattern is captured through the calculation of age-to-age development factors.
2. Step-by-Step Application:
The CLM application involves several key steps:
-
Data Preparation: The process starts with assembling a historical claims development triangle. This triangle displays cumulative incurred losses for each accident year (the year in which the claim originated) at various development periods (time elapsed since the accident year). For instance, a 10-year triangle would show cumulative losses for 10 accident years, observed over 10 development periods.
-
Calculating Age-to-Age Factors: The next step involves calculating age-to-age development factors. These factors represent the average increase in cumulative losses from one development period to the next. They are calculated by dividing the cumulative losses at a given development period by the cumulative losses at the preceding period. For example, the factor from development period 1 to 2 would be calculated as:
Development Factor (1 to 2) = Cumulative Losses at Development Period 2 / Cumulative Losses at Development Period 1
This process is repeated for each development period and each accident year, resulting in a set of age-to-age factors for each age. These are often averaged across accident years to obtain a single development factor for each age. Various averaging techniques can be used, such as weighted averages or medians, depending on the data's characteristics.
-
Estimating Ultimate Losses: Once the age-to-age factors are calculated, they are used to project the ultimate losses for each accident year. For accident years with incomplete development, the age-to-age factors are applied iteratively to the most recent cumulative loss figures until the ultimate loss is estimated. For example, if the latest cumulative loss for accident year 10 is at development period 5, and age-to-age factors for periods 6 to 10 are available, the ultimate loss estimate for accident year 10 will be obtained by sequentially applying the age-to-age factors to the cumulative loss at development period 5.
-
Calculating Total Reserve: Finally, the total reserve is calculated by summing the difference between the estimated ultimate loss and the latest cumulative loss for each accident year. This represents the total amount of reserves that need to be set aside to cover future claim payments.
3. Assumptions and Limitations:
The CLM relies on several crucial assumptions, including:
- Homogeneity: The method assumes that claims development patterns are consistent across different accident years. Significant changes in underwriting practices, claims handling procedures, or the insured population can invalidate this assumption.
- Independence: It assumes that the development of claims in one accident year is independent of the development in other accident years. This assumption might be violated if there are common factors affecting all accident years, such as economic downturns or changes in legislation.
- Stationarity: The method assumes that the underlying process generating claims is stationary, meaning that the statistical properties of the process do not change over time. This assumption can be violated if there are significant changes in the risk profile of the insured population or in the claims handling process.
- No Catastrophic Events: The CLM generally doesn't adequately handle catastrophic events which can significantly distort the historical claims patterns.
These assumptions are often unrealistic, and deviations from these assumptions can lead to inaccurate reserve estimates.
4. Interpreting Results:
The output of the CLM is a set of estimated ultimate losses and a total reserve estimate. These estimates should be interpreted cautiously, considering the assumptions and limitations of the method. Sensitivity analysis, which involves varying the input parameters and observing the impact on the output, is crucial for assessing the uncertainty inherent in the estimates. Additionally, comparing the CLM results with those of other loss reserving methods can provide valuable insights and increase confidence in the accuracy of the estimates.
5. Advanced Considerations:
Several refinements and extensions of the basic CLM exist to address its limitations. These include:
- Weighted Chain Ladder: This method assigns weights to the age-to-age factors to account for the variability in the data.
- Bootstrap Method: This resampling technique helps quantify the uncertainty in the CLM estimates.
- Generalized Linear Models (GLMs): GLMs offer a more flexible framework for modeling claims development, allowing for the incorporation of external factors.
- Bayesian Methods: Bayesian methods provide a framework for incorporating prior information about claims development into the estimation process.
Exploring the Connection Between Data Quality and the Chain Ladder Method:
The accuracy of the CLM heavily relies on the quality of the underlying claims data. Incomplete or inaccurate data can lead to biased and unreliable reserve estimates.
Key Factors to Consider:
-
Roles and Real-World Examples: High-quality data involves accurate recording of claims, consistent reporting practices, and reliable data management systems. Inaccurate data entry, delayed claim reporting, or inconsistencies in claim classification can all affect the reliability of the CLM results.
-
Risks and Mitigations: Data quality issues can be mitigated through robust data validation procedures, regular audits of claims data, and the use of advanced data analytics techniques to identify and correct anomalies.
-
Impact and Implications: Poor data quality can result in underestimated or overestimated reserves, leading to financial instability, regulatory penalties, or inadequate coverage for future claims.
Conclusion: Reinforcing the Connection:
The quality of input data is critical for obtaining reliable reserve estimates using the CLM. Insurers need to invest in robust data management systems and implement rigorous data validation procedures to ensure the accuracy and reliability of their claims data.
Further Analysis: Examining Data Quality in Greater Detail:
A detailed examination of data quality involves assessing data completeness, accuracy, consistency, and timeliness. This can be achieved through various statistical techniques, such as outlier detection, data profiling, and data visualization.
FAQ Section: Answering Common Questions About the Chain Ladder Method:
-
Q: What are the main assumptions of the CLM?
- A: The CLM assumes homogeneity, independence, stationarity, and the absence of significant catastrophic events in the historical data.
-
Q: How can I improve the accuracy of my CLM estimates?
- A: By using more refined versions of the CLM, incorporating external factors (e.g., inflation, changes in legislation), and rigorously validating data quality.
-
Q: What are the limitations of the CLM?
- A: The CLM is highly sensitive to data quality and its underlying assumptions may not hold in practice. It also struggles to handle large, unpredictable claims or changes in the claim environment.
-
Q: What are some alternative methods to the CLM?
- A: Alternative loss reserving techniques include Bornhuetter-Ferguson, Cape Cod, and various statistical models like GLMs and Bayesian methods.
Practical Tips: Maximizing the Benefits of the Chain Ladder Method:
- Step 1: Data Cleaning and Validation: Before applying the CLM, rigorously clean and validate the claims data to remove inconsistencies and outliers.
- Step 2: Exploratory Data Analysis: Perform exploratory data analysis to understand the underlying patterns and trends in the claims data before applying the CLM.
- Step 3: Model Selection: Consider different versions of the CLM or alternative methods to find the most appropriate model for your specific data.
- Step 4: Sensitivity Analysis: Conduct sensitivity analysis to assess the uncertainty of the reserve estimates.
- Step 5: Regular Monitoring and Review: Regularly monitor and review the reserve estimates to account for changes in claims development patterns.
Final Conclusion: Wrapping Up with Lasting Insights:
The Chain Ladder Method is a fundamental tool in actuarial science, providing valuable insights into future claims costs. While it has limitations, its simplicity and widespread application make it a cornerstone of loss reserving. By understanding its assumptions, limitations, and refinements, insurers can leverage the CLM effectively, enhancing their risk management capabilities and ensuring financial stability. However, remember that the CLM should be used in conjunction with other methods and a thorough understanding of the underlying data and business context to provide the most accurate and reliable reserve estimates.
Latest Posts
Related Post
Thank you for visiting our website which covers about Chain Ladder Method Clm Definition Steps To Apply It . We hope the information provided has been useful to you. Feel free to contact us if you have any questions or need further assistance. See you next time and don't miss to bookmark.