Central Limit Theorem Clt Definition And Key Characteristics
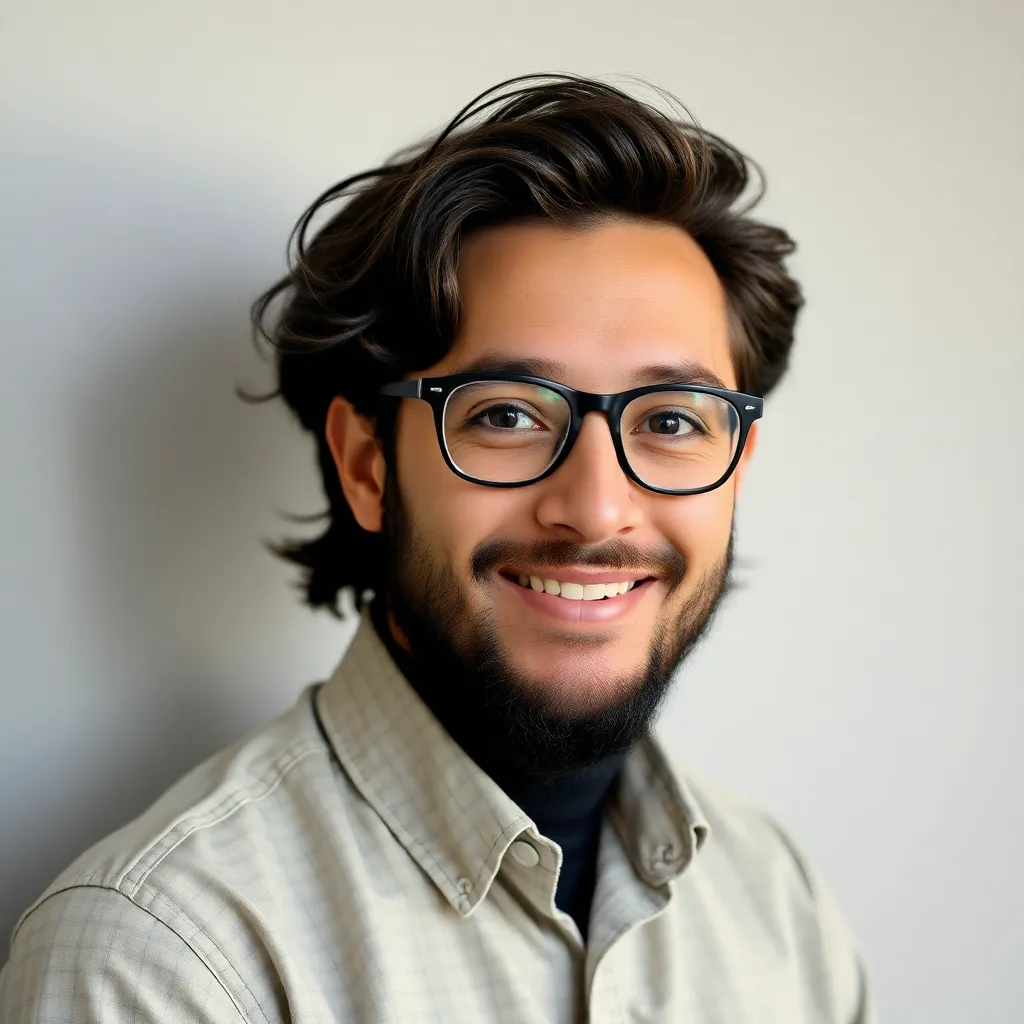
adminse
Mar 12, 2025 · 8 min read

Table of Contents
Unveiling the Power of the Central Limit Theorem: Definition and Key Characteristics
What if the seemingly unpredictable behavior of large datasets could be understood through a single, elegant theorem? The Central Limit Theorem (CLT) is that transformative concept, providing a cornerstone for statistical inference and unlocking a world of possibilities in data analysis.
Editor’s Note: This article on the Central Limit Theorem (CLT) provides a comprehensive overview of its definition, key characteristics, and practical implications. It aims to demystify this fundamental statistical concept, making it accessible to a broad audience.
Why the Central Limit Theorem Matters:
The Central Limit Theorem is not merely an academic curiosity; it's a powerful tool with far-reaching consequences across numerous fields. Its significance stems from its ability to simplify complex probability distributions, allowing researchers to make inferences and predictions about populations based on relatively small samples. From quality control in manufacturing to financial modeling and medical research, the CLT underpins countless statistical analyses, enabling informed decision-making in diverse sectors. Understanding the CLT is crucial for anyone working with data, allowing for more accurate interpretations and more robust conclusions.
Overview: What This Article Covers:
This article provides a detailed exploration of the Central Limit Theorem. We will begin by defining the CLT and explaining its core principles. Then, we will delve into its key characteristics, exploring the conditions under which it holds true and the factors that influence its accuracy. We will analyze the theorem's implications for statistical inference and examine its practical applications across various fields. Finally, we will address some common misconceptions and conclude with a summary of the theorem's enduring importance in statistics.
The Research and Effort Behind the Insights:
This article draws upon established statistical literature, including seminal texts on probability and statistics, along with numerous peer-reviewed research papers. The explanations and examples are designed to be clear, concise, and accessible, avoiding unnecessary technical jargon. The goal is to provide a rigorous yet understandable exposition of the CLT, making its power and utility evident to a wide audience.
Key Takeaways:
- Definition and Core Concepts: A precise definition of the Central Limit Theorem and its underlying principles.
- Conditions for Applicability: An examination of the necessary conditions for the CLT to hold, including sample size and population distribution.
- Impact on Sampling Distributions: How the CLT shapes the distribution of sample means, regardless of the underlying population distribution.
- Applications in Statistical Inference: The role of the CLT in hypothesis testing, confidence intervals, and other inferential procedures.
- Limitations and Misconceptions: Addressing common misunderstandings and limitations associated with the CLT.
Smooth Transition to the Core Discussion:
Having established the importance and scope of this article, let's now dive into a detailed explanation of the Central Limit Theorem itself.
Exploring the Key Aspects of the Central Limit Theorem:
1. Definition and Core Concepts:
The Central Limit Theorem states that the distribution of the sample means from a large number of independent, identically distributed (i.i.d.) random variables, regardless of the shape of the population distribution, will approximate a normal distribution as the sample size increases. More formally, if X₁, X₂, ..., Xₙ are i.i.d. random variables with mean μ and variance σ², then the sample mean X̄ = (X₁ + X₂ + ... + Xₙ)/n will have an approximately normal distribution with mean μ and variance σ²/n as n approaches infinity.
2. Conditions for Applicability:
While the CLT is remarkably robust, certain conditions are generally assumed for its application:
- Independence: The observations within the sample must be independent. This means that the value of one observation should not influence the value of another.
- Identical Distribution: The observations should come from the same population, implying that they share the same underlying probability distribution.
- Finite Variance: The population from which the samples are drawn must have a finite variance. This condition is crucial for the convergence to a normal distribution.
- Sample Size: While the CLT technically applies as the sample size approaches infinity, it often provides a reasonable approximation even with moderately sized samples (generally n ≥ 30 is considered a rule of thumb, but the required sample size depends on the shape of the underlying population distribution). The more skewed the population distribution, the larger the sample size required for the approximation to hold.
3. Impact on Sampling Distributions:
The most profound impact of the CLT is its effect on the sampling distribution of the mean. Even if the underlying population distribution is highly skewed or non-normal, the sampling distribution of the mean will tend toward a normal distribution as the sample size increases. This is crucial because many statistical methods rely on the assumption of normality.
4. Applications in Statistical Inference:
The CLT forms the foundation for many fundamental statistical inference procedures, including:
- Hypothesis Testing: Many hypothesis tests rely on the assumption of normality, and the CLT justifies this assumption even when the population distribution is unknown.
- Confidence Intervals: Confidence intervals for population means are often constructed using the normal distribution, with the CLT providing the justification for this approach.
- Estimation: The CLT allows for the estimation of population parameters using sample statistics, even when the population distribution is non-normal.
5. Limitations and Misconceptions:
It's important to acknowledge some limitations and potential misconceptions:
- Small Sample Sizes: The CLT is an approximation, and its accuracy decreases with smaller sample sizes, particularly when the population distribution is heavily skewed.
- Non-Independent Data: The CLT does not apply to dependent data, such as time series data where consecutive observations are correlated.
- Outliers: Extreme outliers can significantly affect the accuracy of the CLT approximation.
- Infinite Variance: If the population variance is infinite, the CLT does not hold.
Closing Insights: Summarizing the Core Discussion:
The Central Limit Theorem is a fundamental concept in statistics with far-reaching implications. Its ability to transform non-normal distributions into approximately normal distributions for sample means is a cornerstone of statistical inference. Understanding its conditions of applicability and limitations is crucial for proper application and interpretation.
Exploring the Connection Between Sample Size and the Central Limit Theorem:
The relationship between sample size and the CLT is paramount. The accuracy of the normal approximation improves as the sample size increases. A smaller sample size might not adequately reflect the population distribution, leading to inaccurate inferences.
Key Factors to Consider:
- Roles and Real-World Examples: Consider a survey aiming to estimate the average income of a city's residents. A small sample size might yield a highly variable estimate, while a larger sample size will produce a more accurate and reliable result, thanks to the CLT.
- Risks and Mitigations: Using a small sample size with a skewed population distribution risks a poor normal approximation, leading to inaccurate conclusions. Mitigation involves increasing the sample size or employing non-parametric methods.
- Impact and Implications: The sample size significantly impacts the precision of estimates and the validity of inferential tests. Larger samples lead to narrower confidence intervals and increased power in hypothesis tests.
Conclusion: Reinforcing the Connection:
The sample size directly dictates how closely the sampling distribution of the mean resembles a normal distribution, according to the CLT. Understanding this connection is vital for designing appropriate statistical studies and interpreting results effectively.
Further Analysis: Examining Sample Size in Greater Detail:
The impact of sample size isn't solely about achieving normality. Larger samples also reduce sampling error, making estimates more precise. This is reflected in the formula for the standard error of the mean (σ/√n), which decreases as n increases. Thus, larger samples lead to more reliable and precise inferences.
FAQ Section: Answering Common Questions About the Central Limit Theorem:
Q: What is the Central Limit Theorem?
A: The Central Limit Theorem states that the sampling distribution of the mean from a large number of independent, identically distributed random variables will be approximately normal, regardless of the shape of the original population distribution.
Q: What are the conditions required for the CLT to apply?
A: The primary conditions are independence of observations, identical distribution of observations, and a finite population variance. A sufficiently large sample size is also crucial for a good approximation.
Q: How does the CLT impact statistical inference?
A: The CLT underpins many statistical procedures, enabling us to make inferences about population parameters (e.g., mean) using sample data, even when the population distribution is unknown.
Q: What happens if the sample size is small?
A: With small sample sizes, the approximation to normality might be poor, leading to inaccurate inferences. For small samples and non-normal data, non-parametric methods may be preferred.
Practical Tips: Maximizing the Benefits of the Central Limit Theorem:
- Ensure Independence: Carefully consider the sampling method to avoid introducing dependencies among observations.
- Check for Outliers: Identify and address outliers to prevent them from unduly influencing the results.
- Assess Sample Size: Choose a sample size that is large enough to ensure a reasonable approximation to normality, considering the skewness of the population distribution.
- Use Appropriate Statistical Methods: Select statistical methods that are robust to violations of normality when necessary.
Final Conclusion: Wrapping Up with Lasting Insights:
The Central Limit Theorem is a cornerstone of statistical theory and practice. Its remarkable ability to simplify complex probability distributions has revolutionized the field, empowering researchers and practitioners across diverse domains. By understanding its principles and limitations, one can harness its power to make more accurate, reliable, and informed decisions based on data analysis. Its significance extends far beyond theoretical statistics; it's a practical tool for interpreting data and drawing meaningful conclusions in the real world.
Latest Posts
Related Post
Thank you for visiting our website which covers about Central Limit Theorem Clt Definition And Key Characteristics . We hope the information provided has been useful to you. Feel free to contact us if you have any questions or need further assistance. See you next time and don't miss to bookmark.