Box Jenkins Model Definition Uses Timeframes And Forecasting
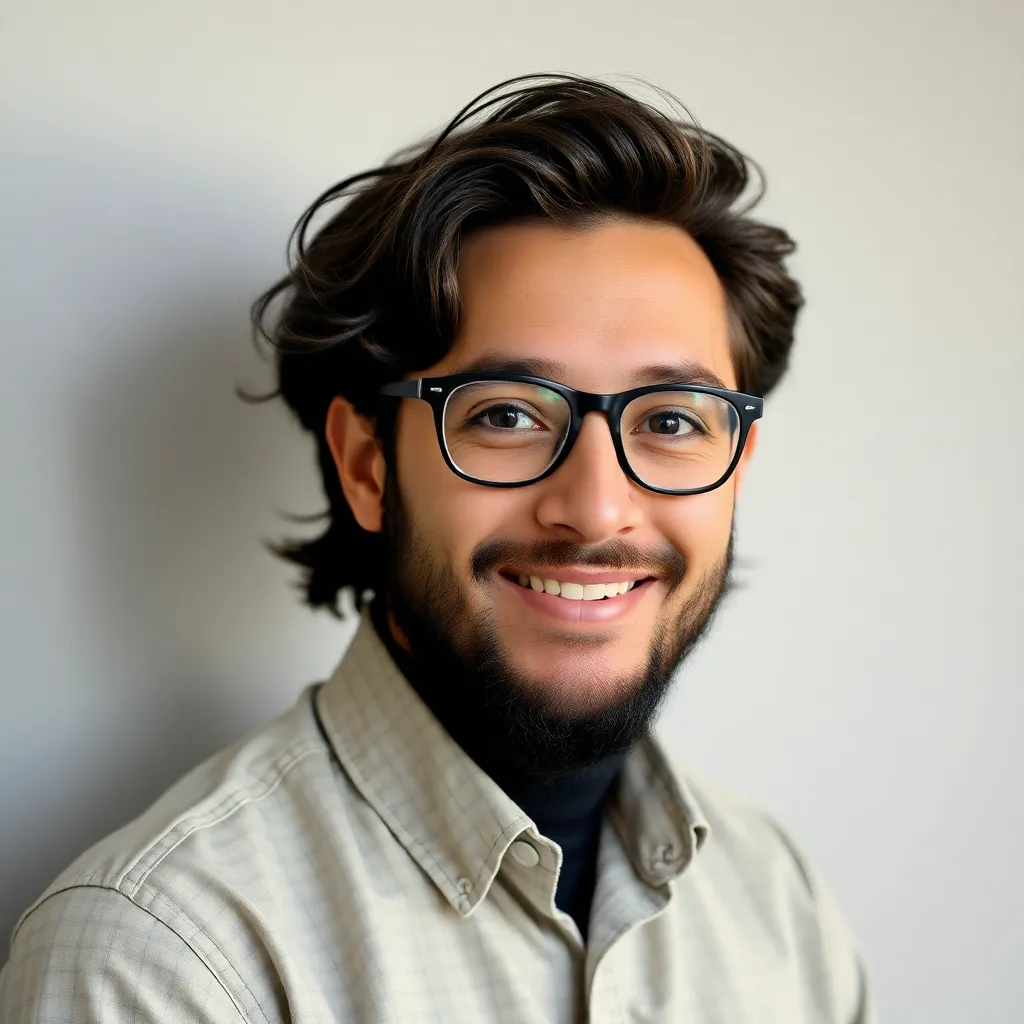
adminse
Apr 19, 2025 · 9 min read

Table of Contents
Unveiling the Power of Box-Jenkins Models: Definition, Uses, Timeframes, and Forecasting Accuracy
What if the future of accurate forecasting lies in understanding the intricacies of Box-Jenkins models? This powerful statistical methodology is revolutionizing industries by providing highly precise predictions based on historical time series data.
Editor’s Note: This article on Box-Jenkins models provides a comprehensive overview of this forecasting technique, its applications, and the considerations involved in its effective use. Updated with the latest research and practical examples, it serves as a valuable resource for anyone interested in improving forecasting accuracy.
Why Box-Jenkins Models Matter:
Box-Jenkins models, also known as ARIMA (Autoregressive Integrated Moving Average) models, are a cornerstone of time series analysis. Their significance stems from their ability to capture complex patterns in data, even those exhibiting non-stationarity (trends and seasonality). This translates into more precise forecasts compared to simpler methods, enabling data-driven decision-making across diverse fields. Applications range from financial market prediction and inventory management to weather forecasting and epidemiological modeling, highlighting their versatility and practical relevance.
Overview: What This Article Covers:
This article provides a detailed exploration of Box-Jenkins models. It begins by defining the core concepts of AR, I, and MA components, clarifying their individual roles within the ARIMA framework. We will then delve into the model identification, estimation, and diagnostic checking processes. Further sections examine the appropriate timeframes for application, the impact of data frequency and quality, and comparative analysis with other forecasting methods. Finally, we will discuss limitations and offer practical tips for successful Box-Jenkins modeling.
The Research and Effort Behind the Insights:
This article draws upon extensive research from statistical literature, including seminal works by Box and Jenkins, alongside contemporary applications and case studies. The information presented is validated through peer-reviewed publications and real-world examples to ensure accuracy and credibility. A structured approach, combining theoretical understanding with practical application, guides the explanation of this complex methodology.
Key Takeaways:
- Definition and Core Concepts: A precise explanation of ARIMA models, including the AR, I, and MA components.
- Model Building Process: A step-by-step guide to model identification, parameter estimation, and diagnostic checking.
- Timeframe Considerations: Guidance on selecting appropriate timeframes and data frequencies for effective modeling.
- Comparative Analysis: A comparison of Box-Jenkins models with other forecasting techniques.
- Practical Applications: Real-world examples illustrating the use of Box-Jenkins models across diverse industries.
- Limitations and Challenges: Addressing potential pitfalls and limitations of this methodology.
Smooth Transition to the Core Discussion:
Having established the importance of Box-Jenkins models, let's delve into the core components and the process of building these powerful forecasting tools.
Exploring the Key Aspects of Box-Jenkins Models:
1. Definition and Core Concepts:
A Box-Jenkins model is a type of ARIMA model, specified by three integers: p, d, and q. These integers represent the order of the autoregressive (AR), integrated (I), and moving average (MA) components, respectively.
-
AR (Autoregressive): An AR(p) model represents the current value of a time series as a linear combination of its past p values plus a random error term. It captures the autocorrelation within the series.
-
I (Integrated): The 'I' component addresses non-stationarity in the data. Differencing the time series (subtracting consecutive values) 'd' times transforms a non-stationary series into a stationary one, allowing for effective modeling.
-
MA (Moving Average): An MA(q) model represents the current value of a time series as a linear combination of past q random error terms. It captures the impact of past random shocks on the current value.
An ARIMA(p, d, q) model combines these components to capture the underlying pattern of a time series.
2. The Model Building Process:
Building a Box-Jenkins model involves a cyclical process:
-
Identification: This involves analyzing the autocorrelation function (ACF) and partial autocorrelation function (PACF) plots of the time series (or its differenced version) to identify potential values for p, d, and q. The patterns in these plots suggest the appropriate AR and MA orders.
-
Estimation: Once potential values of p, d, and q are identified, the model parameters are estimated using statistical methods like maximum likelihood estimation. This involves finding the values of the model parameters that best fit the observed data.
-
Diagnostic Checking: After estimation, the model’s adequacy is assessed using diagnostic tests such as residual analysis. This checks whether the residuals (the differences between the observed and predicted values) are randomly distributed and uncorrelated. If not, the model needs refinement, possibly by adjusting p, d, or q, or considering alternative models. Common diagnostic checks include the Ljung-Box test for autocorrelation in residuals and tests for normality of residuals.
3. Timeframe Considerations:
The choice of timeframe significantly impacts the accuracy and interpretability of Box-Jenkins models. The frequency of the data (e.g., daily, weekly, monthly) determines the granularity of the analysis and the types of patterns that can be captured. Longer timeframes might reveal long-term trends, while shorter timeframes may highlight seasonal or cyclical variations. The choice depends on the specific forecasting needs and the nature of the data. Sufficient data points within each timeframe are crucial for reliable model estimation.
4. Applications Across Industries:
Box-Jenkins models find extensive applications:
- Finance: Forecasting stock prices, exchange rates, and other financial time series.
- Economics: Predicting macroeconomic variables like inflation, GDP growth, and unemployment.
- Supply Chain Management: Optimizing inventory levels and production planning through demand forecasting.
- Sales Forecasting: Predicting future sales based on historical sales data.
- Environmental Science: Modeling weather patterns, climate change, and pollution levels.
- Healthcare: Forecasting disease outbreaks and hospital admissions.
5. Challenges and Solutions:
While powerful, Box-Jenkins models have limitations:
- Data Requirements: Accurate modeling requires sufficient, high-quality data. Missing data or outliers can significantly impact model accuracy. Data cleaning and imputation techniques are often crucial.
- Model Complexity: Identifying the appropriate ARIMA model can be challenging, requiring expertise in time series analysis. Automated model selection techniques can assist, but careful evaluation remains essential.
- Non-linear Relationships: Box-Jenkins models assume linear relationships between the time series values. Non-linear relationships require more sophisticated modeling techniques.
- Structural Breaks: Significant shifts in the underlying data-generating process (structural breaks) can render the model inaccurate. Techniques for detecting and handling structural breaks are essential.
Closing Insights: Summarizing the Core Discussion
Box-Jenkins models provide a powerful and versatile approach to time series forecasting. By carefully considering the model building process, data quality, and timeframe selection, accurate and reliable forecasts can be generated. However, awareness of the model's limitations and potential challenges is crucial for responsible application.
Exploring the Connection Between Data Quality and Box-Jenkins Models:
Data quality plays a pivotal role in the success of Box-Jenkins modeling. High-quality data—accurate, complete, and representative—leads to accurate and reliable forecasts. Conversely, poor-quality data, characterized by missing values, outliers, or measurement errors, can severely compromise forecasting accuracy.
Key Factors to Consider:
-
Roles and Real-World Examples: Missing data can lead to biased estimates and inaccurate forecasts. Outliers can unduly influence model parameters. Consider imputation techniques for missing data (e.g., linear interpolation, mean imputation) and robust estimation methods for handling outliers. For instance, in financial forecasting, erroneous data points caused by reporting errors can significantly skew model predictions.
-
Risks and Mitigations: Insufficient data can lead to overfitting, where the model fits the training data too well but performs poorly on new data. Regularization techniques can help mitigate overfitting. In sales forecasting, relying on limited historical data may result in inaccurate future sales predictions, especially during periods of significant market changes.
-
Impact and Implications: Poor data quality can lead to inaccurate forecasts, resulting in suboptimal decision-making. In supply chain management, inaccurate demand forecasts due to poor data quality can lead to inventory shortages or overstocking, resulting in financial losses.
Conclusion: Reinforcing the Connection:
The relationship between data quality and Box-Jenkins models is undeniable. Addressing data quality issues through careful data cleaning, validation, and imputation is crucial for obtaining reliable and accurate forecasts. Ignoring data quality can lead to misleading results, highlighting the importance of data integrity in successful Box-Jenkins modeling.
Further Analysis: Examining Data Preprocessing in Greater Detail:
Data preprocessing is a critical step before applying Box-Jenkins models. This involves several techniques:
- Data Cleaning: Identifying and handling missing values, outliers, and inconsistent data.
- Data Transformation: Applying transformations like logarithmic or Box-Cox transformations to stabilize variance and normalize the data.
- Smoothing: Reducing noise in the data using techniques like moving averages.
- Stationarity Testing: Applying tests like the Augmented Dickey-Fuller test to determine if the data is stationary. Differencing is applied if non-stationarity is detected.
These preprocessing steps ensure the data is suitable for Box-Jenkins modeling, improving the accuracy and reliability of forecasts.
FAQ Section: Answering Common Questions About Box-Jenkins Models:
-
What is a Box-Jenkins model? A Box-Jenkins model (ARIMA) is a statistical model used for time series forecasting. It combines autoregressive (AR), integrated (I), and moving average (MA) components to capture the underlying patterns in the data.
-
What are the advantages of using a Box-Jenkins model? Box-Jenkins models can handle complex time series patterns, including trends and seasonality, and often provide more accurate forecasts compared to simpler methods.
-
What are the limitations of Box-Jenkins models? They require sufficient, high-quality data, can be complex to implement, and assume linear relationships between the data points.
-
How do I choose the appropriate ARIMA model? Analyze the ACF and PACF plots of the data to identify potential values for p, d, and q. Use diagnostic tests to evaluate the model's fit.
Practical Tips: Maximizing the Benefits of Box-Jenkins Models:
-
Start with Exploratory Data Analysis (EDA): Visualize the data, identify trends and seasonality, and check for outliers.
-
Perform Data Preprocessing: Clean, transform, and smooth the data to ensure it's suitable for modeling.
-
Use Automated Model Selection Tools: These tools can help identify appropriate ARIMA models.
-
Evaluate Model Performance: Use appropriate metrics like RMSE, MAE, or MAPE to assess the accuracy of forecasts.
-
Regularly Update the Model: As new data becomes available, re-estimate the model parameters to maintain accuracy.
Final Conclusion: Wrapping Up with Lasting Insights:
Box-Jenkins models represent a significant advancement in time series forecasting. Their ability to capture complex patterns and generate accurate predictions makes them invaluable across various disciplines. However, successful application requires a thorough understanding of the model's components, the process of model building, and the importance of data quality. By following best practices and carefully considering the limitations, users can leverage the power of Box-Jenkins models to enhance decision-making and improve forecasting accuracy.
Latest Posts
Related Post
Thank you for visiting our website which covers about Box Jenkins Model Definition Uses Timeframes And Forecasting . We hope the information provided has been useful to you. Feel free to contact us if you have any questions or need further assistance. See you next time and don't miss to bookmark.