Benchmark For Correlation Values Definition
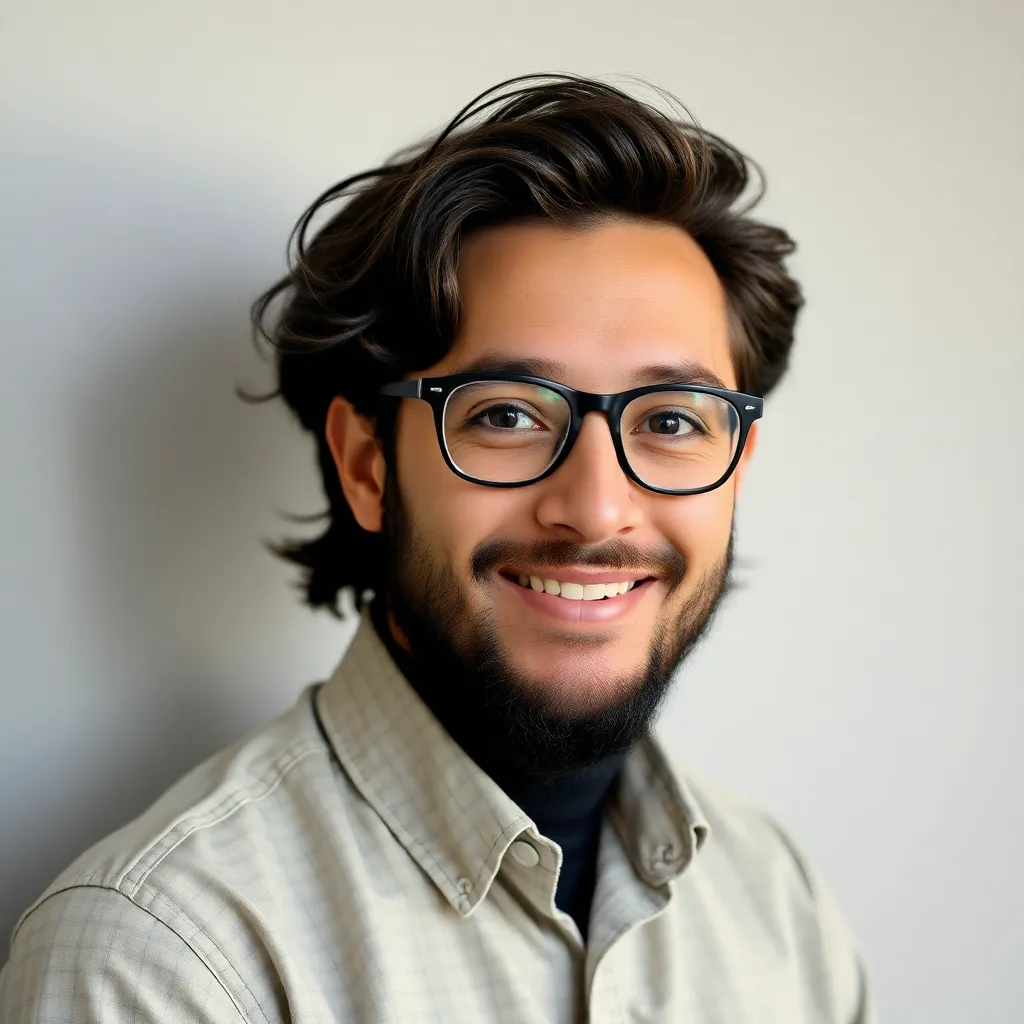
adminse
Apr 18, 2025 · 8 min read

Table of Contents
Benchmarking Correlation Values: Defining Strength and Significance in Relationships
What if the seemingly simple act of interpreting a correlation coefficient held the key to unlocking deeper insights from your data? Understanding benchmarks for correlation values is crucial for accurately assessing the strength and significance of relationships between variables, avoiding misinterpretations and enabling data-driven decision-making.
Editor’s Note: This article on benchmarking correlation values was published today, providing readers with up-to-date insights and best practices for interpreting correlation coefficients effectively.
Why Benchmarking Correlation Values Matters:
Correlation analysis is a cornerstone of statistical analysis, allowing researchers and analysts to quantify the linear association between two or more variables. The correlation coefficient, typically represented by 'r', ranges from -1 to +1. However, simply obtaining a correlation coefficient isn't enough; understanding what constitutes a "strong," "moderate," or "weak" correlation is critical for valid conclusions. Benchmarking helps establish these thresholds, ensuring consistent interpretation across different studies and datasets. This is vital in various fields, including finance (assessing asset correlations), healthcare (investigating relationships between risk factors and disease), and social sciences (exploring correlations between social indicators). Misinterpreting correlation values can lead to flawed conclusions, incorrect predictions, and ultimately, suboptimal decision-making.
Overview: What This Article Covers:
This article provides a comprehensive guide to benchmarking correlation values. We will explore the definition and interpretation of correlation coefficients, discuss common benchmarks used to classify correlation strength, delve into the importance of statistical significance testing, and examine the limitations and potential pitfalls of solely relying on correlation analysis. We will also consider the impact of sample size on correlation benchmarks and address frequently asked questions about interpreting correlation results.
The Research and Effort Behind the Insights:
This article is based on a thorough review of statistical literature, including seminal works on correlation analysis and contemporary research employing correlation techniques across various disciplines. Established statistical guidelines and best practices have been incorporated to ensure accuracy and clarity.
Key Takeaways:
- Definition and Core Concepts: A precise understanding of correlation coefficients and their range.
- Benchmark Categories: Exploring commonly used benchmarks for classifying correlation strength (e.g., weak, moderate, strong).
- Statistical Significance: Understanding the importance of p-values and their role in interpreting correlation results.
- Sample Size Considerations: Acknowledging the impact of sample size on correlation strength and statistical significance.
- Limitations of Correlation: Recognizing the limitations of correlation analysis and the potential for spurious correlations.
- Practical Applications: Illustrative examples of how correlation benchmarks are used across various fields.
Smooth Transition to the Core Discussion:
Having established the importance of benchmarking correlation values, let’s delve into the specifics of interpreting correlation coefficients and understanding their strength and significance.
Exploring the Key Aspects of Benchmarking Correlation Values:
1. Definition and Core Concepts:
The Pearson correlation coefficient (r) measures the linear association between two continuous variables. A positive r indicates a positive relationship (as one variable increases, the other tends to increase), while a negative r indicates a negative relationship (as one variable increases, the other tends to decrease). An r of 0 suggests no linear relationship. It's crucial to remember that correlation does not imply causation. A strong correlation might exist between two variables without one directly causing changes in the other; a third, unmeasured variable could be influencing both.
2. Benchmark Categories for Correlation Strength:
While there isn't a universally agreed-upon set of benchmarks, the following categories are commonly used to interpret the magnitude of a correlation coefficient:
-
Weak Correlation: |r| < 0.3. Indicates a weak linear relationship between the variables. The relationship is subtle and may not be practically meaningful.
-
Moderate Correlation: 0.3 ≤ |r| < 0.7. Suggests a noticeable linear relationship, but the association isn't exceptionally strong. Further investigation might be warranted to understand the relationship better.
-
Strong Correlation: |r| ≥ 0.7. Indicates a substantial linear relationship between the variables. The relationship is likely to be practically significant.
It's important to note that these benchmarks are guidelines, not rigid rules. The practical significance of a correlation depends heavily on the context of the study and the variables involved. A correlation of 0.4 might be considered strong in one field but weak in another.
3. Statistical Significance and p-values:
A correlation coefficient alone doesn't tell the whole story. Statistical significance testing determines whether the observed correlation is likely due to chance or represents a true relationship within the population. This is assessed using the p-value. A p-value less than a predetermined significance level (commonly 0.05) indicates that the correlation is statistically significant, meaning it's unlikely to have occurred by chance. A statistically significant correlation, however, doesn't necessarily imply practical significance. A small but statistically significant correlation might not be meaningful in a real-world context.
4. Sample Size Considerations:
The sample size significantly impacts the reliability of correlation estimates. Smaller samples are more prone to random variation, potentially leading to inflated or deflated correlation coefficients. Larger samples generally provide more precise and reliable estimates, making it easier to detect weaker but still meaningful correlations. The required sample size for detecting a correlation of a certain strength depends on factors such as the desired power of the test and the expected effect size.
5. Limitations of Correlation Analysis:
Correlation analysis has limitations. It primarily assesses linear relationships; non-linear relationships may be missed. It doesn't account for confounding variables (variables that influence both the variables being studied), and it doesn't establish causality. A strong correlation may exist due to a third, unmeasured variable influencing both variables being examined. Over-reliance on correlation without considering other aspects of the data can lead to misleading conclusions.
Exploring the Connection Between Statistical Significance and Correlation Strength:
The connection between statistical significance (p-value) and correlation strength (magnitude of 'r') is crucial. A statistically significant correlation means the relationship is unlikely due to chance. However, even a statistically significant correlation might be weak in magnitude, having limited practical implications. Conversely, a strong correlation might not be statistically significant if the sample size is small.
Key Factors to Consider:
-
Roles and Real-World Examples: A statistically significant correlation with a large effect size ('r') is more reliable than one with a small effect size, especially in prediction models. For instance, a strong correlation between blood pressure and age in a large, well-designed study would have significant implications for healthcare.
-
Risks and Mitigations: The risk of misinterpreting correlation lies in assuming causation. Careful consideration of potential confounding variables and causal pathways is necessary. Employing multiple statistical methods and incorporating domain expertise can help mitigate this risk.
-
Impact and Implications: Understanding both the strength and statistical significance of a correlation is crucial for making informed decisions based on data analysis. A strong and statistically significant correlation often informs robust predictions and interventions.
Conclusion: Reinforcing the Connection:
The interplay between correlation strength and statistical significance highlights the complexity of interpreting correlation analysis. Researchers and analysts should not solely rely on p-values but consider the magnitude of 'r' in the context of the research question and potential confounding factors.
Further Analysis: Examining Statistical Power in Greater Detail:
Statistical power refers to the probability of detecting a true effect (correlation) when it exists. Low power increases the risk of Type II error (failing to reject a false null hypothesis – concluding there’s no correlation when one truly exists). Factors impacting power include sample size, effect size (correlation strength), and significance level. Larger sample sizes and larger effect sizes generally lead to higher power. Researchers should aim for sufficient power to ensure their study can detect correlations of meaningful size.
FAQ Section: Answering Common Questions About Benchmarking Correlation Values:
-
Q: What is the most important benchmark for correlation values? A: There's no single most important benchmark. The practical significance of a correlation depends on the context. Both magnitude ('r') and statistical significance (p-value) should be considered.
-
Q: Can a weak correlation be meaningful? A: Yes, a weak correlation might be meaningful in certain contexts, particularly if it involves a large number of observations. Context and the nature of the variables being studied determine the relevance of the correlation coefficient.
-
Q: How do I decide what benchmark to use for my research? A: Review literature in your field to determine what benchmarks are commonly used. Consider the practical implications of different correlation strengths in relation to your research question.
-
Q: What if my correlation is statistically significant but weak? A: This means that while the correlation is unlikely to be due to chance, its magnitude might be too small to have practical implications.
Practical Tips: Maximizing the Benefits of Understanding Correlation Benchmarks:
- Context is Key: Always consider the context of your data and the variables being studied.
- Visualize Your Data: Create scatter plots to visualize the relationship between your variables.
- Report Both Magnitude and Significance: Report both the correlation coefficient ('r') and the p-value.
- Consider Confounding Variables: Think about potential factors influencing both variables of interest.
- Don't Confuse Correlation with Causation: Never assume that correlation implies causation.
- Use Appropriate Statistical Software: Utilize statistical software to perform correlation analysis correctly.
Final Conclusion: Wrapping Up with Lasting Insights:
Benchmarking correlation values is essential for accurately interpreting the strength and significance of relationships between variables. By understanding the benchmarks, considering statistical significance, and acknowledging the limitations of correlation analysis, researchers and analysts can make more informed decisions, avoid misinterpretations, and unlock richer insights from their data. The interplay between correlation magnitude and statistical significance demands a nuanced approach, emphasizing the importance of context, sample size, and a critical appraisal of potential confounding factors. Only with a thorough understanding of these elements can the power of correlation analysis be fully harnessed for data-driven discovery.
Latest Posts
Latest Posts
-
Boon Definition
Apr 19, 2025
-
Boom Definition In Economics And Finance Length Examples
Apr 19, 2025
-
What Is The Difference Between A 401k And A 401a
Apr 19, 2025
-
Boolean Algebra Definition
Apr 19, 2025
-
Boom And Bust Cycle Definition How It Works And History
Apr 19, 2025
Related Post
Thank you for visiting our website which covers about Benchmark For Correlation Values Definition . We hope the information provided has been useful to you. Feel free to contact us if you have any questions or need further assistance. See you next time and don't miss to bookmark.