Bell Curve Definition Normal Distribution Meaning Example In Finance
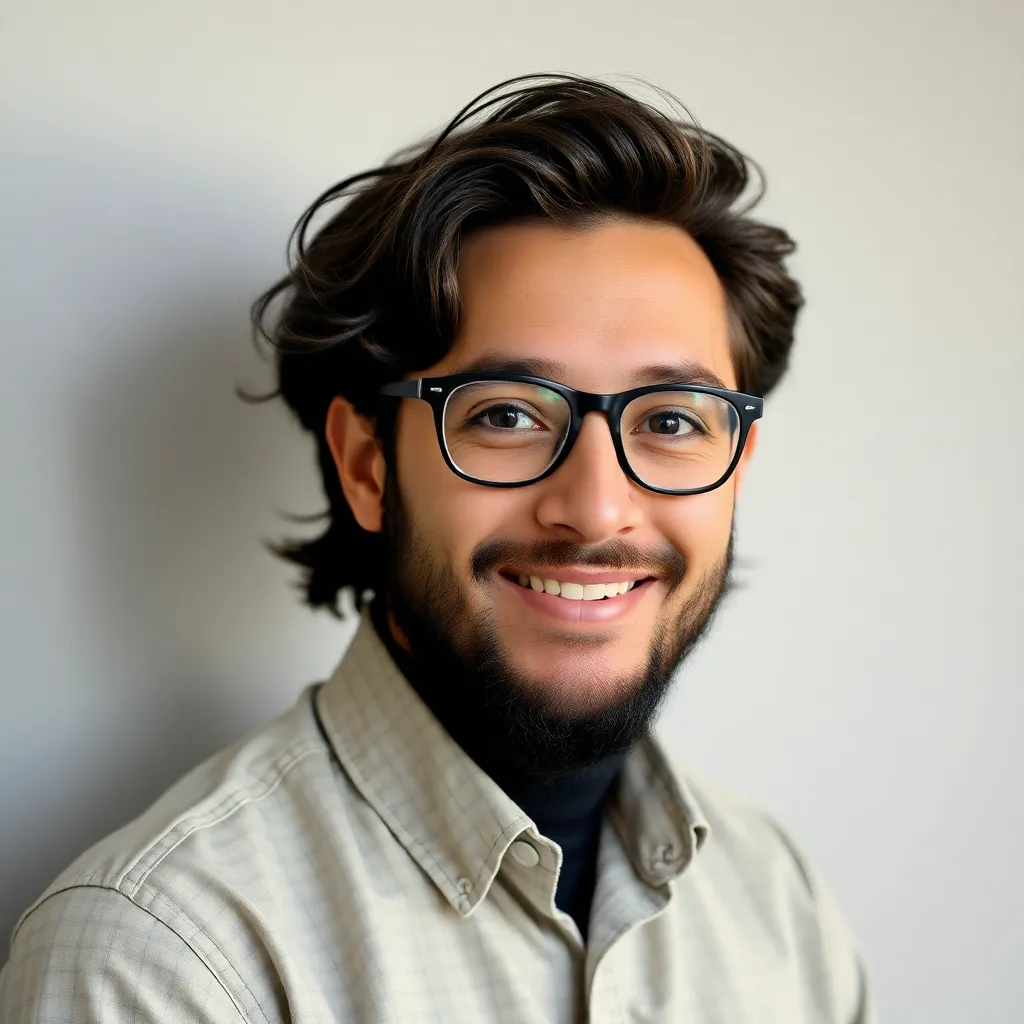
adminse
Apr 18, 2025 · 9 min read

Table of Contents
Decoding the Bell Curve: Understanding Normal Distribution and its Significance in Finance
What if the future of financial modeling hinges on a thorough understanding of the bell curve? This ubiquitous statistical concept underpins countless financial decisions, from risk management to portfolio optimization.
Editor’s Note: This article on the bell curve, normal distribution, and its applications in finance was published today, providing readers with current and relevant information. The insights offered are grounded in established statistical principles and real-world financial examples.
Why the Bell Curve Matters: Relevance, Practical Applications, and Industry Significance
The bell curve, more formally known as the normal distribution, is a fundamental concept in statistics and holds immense significance across various fields, particularly finance. Its relevance stems from its ability to model a wide range of phenomena, from the distribution of asset returns to the measurement of risk. Understanding the normal distribution allows financial professionals to make more informed decisions, optimize strategies, and effectively manage risk. From pricing options to assessing portfolio performance, the normal distribution provides a crucial framework for understanding and predicting financial outcomes. Its implications are far-reaching, impacting investment strategies, regulatory frameworks, and overall market stability.
Overview: What This Article Covers
This article delves into the core aspects of the normal distribution, starting with its definition and underlying principles. We will explore its practical applications in finance, highlighting examples from various domains such as portfolio management, risk assessment, and option pricing. The article will also address common misconceptions and limitations of the normal distribution, paving the way for a more nuanced understanding of its role in financial modeling. Finally, we'll examine how deviations from normality can impact financial decisions.
The Research and Effort Behind the Insights
This article is the result of extensive research, drawing upon established statistical literature, reputable financial textbooks, and peer-reviewed academic papers. The explanations and examples provided are supported by evidence and aim to offer a clear, concise, and accurate understanding of the topic. The structured approach ensures readers receive reliable and trustworthy information.
Key Takeaways:
- Definition and Core Concepts: A precise definition of the normal distribution and its key characteristics, including mean, median, mode, and standard deviation.
- Practical Applications in Finance: Detailed examples of how the normal distribution is used in portfolio theory, risk management, and options pricing.
- Limitations and Deviations from Normality: A critical examination of the circumstances where the normal distribution may not accurately reflect real-world financial data, and the implications of such deviations.
- Alternative Distributions: A brief introduction to alternative distributions used when the assumption of normality is violated.
Smooth Transition to the Core Discussion
Having established the importance of the normal distribution in finance, let’s now delve into a detailed exploration of its definition, characteristics, and applications.
Exploring the Key Aspects of the Normal Distribution
Definition and Core Concepts:
The normal distribution, also known as the Gaussian distribution, is a continuous probability distribution characterized by its bell-shaped curve. It's defined by two parameters: the mean (μ) and the standard deviation (σ). The mean represents the average value of the distribution, while the standard deviation measures the dispersion or spread of the data around the mean. A higher standard deviation indicates greater variability. The normal distribution is symmetric around its mean, meaning that the probability of observing a value below the mean is equal to the probability of observing a value above the mean. The area under the curve represents the total probability, which is always equal to 1.
Applications Across Industries: Focus on Finance
The normal distribution is extensively used in finance due to its ability to approximate the distribution of many financial variables. Some key applications include:
-
Portfolio Theory: Modern portfolio theory (MPT) relies heavily on the assumption of normally distributed asset returns. The mean and standard deviation of returns are used to calculate portfolio risk and return, enabling investors to construct efficient portfolios that maximize return for a given level of risk, or minimize risk for a given level of return. The Sharpe ratio, a widely used performance metric, also relies on the assumption of normal returns.
-
Risk Management: The normal distribution is frequently employed in risk management to model and quantify various types of financial risks, such as market risk, credit risk, and operational risk. Value at Risk (VaR) calculations, a crucial tool for assessing potential losses, often assume that asset returns are normally distributed. However, it's crucial to note that the accuracy of VaR calculations can be significantly affected if the assumption of normality is violated.
-
Options Pricing: The Black-Scholes model, a cornerstone of options pricing, assumes that the underlying asset's price follows a geometric Brownian motion, which implies that the logarithm of the asset price is normally distributed. This model provides a theoretical framework for determining fair prices for options contracts.
Challenges and Solutions: Addressing Limitations of Normality
While the normal distribution is a powerful tool, it's crucial to acknowledge its limitations. Real-world financial data often exhibits features that deviate from normality, such as:
- Fat Tails: Financial returns often exhibit heavier tails than predicted by the normal distribution, meaning that extreme events (large gains or losses) occur more frequently than expected under normality.
- Skewness: The distribution of financial returns may be skewed, meaning that it is not symmetric around the mean. Positive skewness indicates a longer right tail, while negative skewness indicates a longer left tail.
- Kurtosis: The distribution may have excess kurtosis, indicating a higher concentration of data around the mean and fatter tails compared to a normal distribution.
These deviations from normality can lead to inaccurate risk assessments and flawed investment decisions. To address these limitations, several approaches are employed:
- Alternative Distributions: When normality assumptions are violated, alternative distributions such as the t-distribution, skewed normal distribution, or stable distributions can be used to better model financial data.
- Non-parametric methods: These methods do not rely on distributional assumptions and are often more robust to deviations from normality.
- Monte Carlo simulations: These simulations allow for the generation of numerous scenarios, reflecting the uncertainty and complexity of financial markets, without relying on a specific distributional assumption.
Impact on Innovation: Adapting to Non-Normal Data
The recognition of deviations from normality has spurred innovation in financial modeling and risk management. Researchers are continuously developing new methods and tools to deal with non-normal data, leading to more accurate and robust models.
Closing Insights: Summarizing the Core Discussion
The normal distribution, while a powerful tool, is not a universal model for financial data. Understanding its limitations and the potential consequences of deviations from normality is crucial for making informed financial decisions. The use of alternative models and robust methods is essential for navigating the complexities and uncertainties inherent in financial markets.
Exploring the Connection Between Volatility Clustering and the Normal Distribution
Volatility clustering, a phenomenon where periods of high volatility tend to be followed by other periods of high volatility, and vice versa, presents a challenge to the assumption of constant volatility inherent in some models based on the normal distribution. The normal distribution assumes constant variance, which is often not the case in financial markets.
Key Factors to Consider:
-
Roles and Real-World Examples: Volatility clustering is observable in many financial time series. For instance, stock prices often exhibit periods of increased volatility followed by relatively calm periods. This violates the constant variance assumption of the normal distribution, leading to miscalculations in risk models such as VaR.
-
Risks and Mitigations: The failure to account for volatility clustering can lead to underestimation of risk, especially during periods of high volatility. To mitigate this risk, models that incorporate time-varying volatility, such as GARCH (Generalized Autoregressive Conditional Heteroskedasticity) models, are often employed. These models explicitly account for the clustering of volatility, providing more accurate risk assessments.
-
Impact and Implications: Ignoring volatility clustering can lead to substantial errors in risk management and investment strategies. It can result in under-diversification of portfolios, inappropriate risk exposures, and poor risk-adjusted returns. Understanding volatility clustering and employing appropriate models are crucial for effective risk management.
Conclusion: Reinforcing the Connection
The interplay between volatility clustering and the normal distribution highlights the limitations of relying solely on the normal distribution for modeling financial data. The failure to account for time-varying volatility can lead to flawed models and potentially disastrous consequences. The adoption of more sophisticated models that account for volatility clustering is critical for building more realistic and accurate financial models.
Further Analysis: Examining Volatility Clustering in Greater Detail
Volatility clustering is a complex phenomenon that is not fully understood. Researchers continue to investigate its causes and implications. Some potential contributing factors include market sentiment, news events, and feedback loops between price changes and volatility. The development of more accurate and robust models that capture the dynamic nature of volatility is an ongoing area of research.
FAQ Section: Answering Common Questions About the Normal Distribution
What is the normal distribution?
The normal distribution is a continuous probability distribution characterized by its bell-shaped curve. It is defined by its mean (μ) and standard deviation (σ), which describe the distribution's central tendency and spread, respectively.
How is the normal distribution used in finance?
The normal distribution is widely used in finance for various purposes, including portfolio optimization, risk management (VaR calculations), options pricing (Black-Scholes model), and hypothesis testing.
What are the limitations of the normal distribution in finance?
The normal distribution’s limitations include its inability to accurately model real-world financial data that exhibit fat tails, skewness, and volatility clustering.
What are alternative distributions used in finance when the assumption of normality is violated?
Alternative distributions include the t-distribution, skewed normal distribution, stable distributions, and others. Non-parametric methods also offer a viable approach.
Practical Tips: Maximizing the Benefits of Understanding the Normal Distribution
- Understand the Basics: Start by grasping the fundamental concepts of the normal distribution, including its parameters (mean and standard deviation) and characteristics (symmetry, area under the curve).
- Recognize Limitations: Be aware of the limitations of the normal distribution and the potential for deviations from normality in real-world financial data.
- Explore Alternatives: Familiarize yourself with alternative distributions and non-parametric methods that can be used when the assumption of normality is violated.
- Use appropriate software: Leverage statistical software packages to perform calculations, generate visualizations, and test for normality.
Final Conclusion: Wrapping Up with Lasting Insights
The normal distribution serves as a fundamental building block in many financial models, providing a valuable framework for understanding and predicting financial outcomes. However, its limitations should be acknowledged, and a nuanced understanding of its applicability is crucial. By recognizing its strengths and weaknesses, and by employing alternative models when necessary, financial professionals can build more robust and accurate models, leading to better informed decisions and more effective risk management. The bell curve remains a cornerstone of financial analysis, but its application must always be considered in the context of real-world complexities.
Latest Posts
Related Post
Thank you for visiting our website which covers about Bell Curve Definition Normal Distribution Meaning Example In Finance . We hope the information provided has been useful to you. Feel free to contact us if you have any questions or need further assistance. See you next time and don't miss to bookmark.